کد مقاله | کد نشریه | سال انتشار | مقاله انگلیسی | نسخه تمام متن |
---|---|---|---|---|
6267626 | 1614601 | 2016 | 6 صفحه PDF | دانلود رایگان |
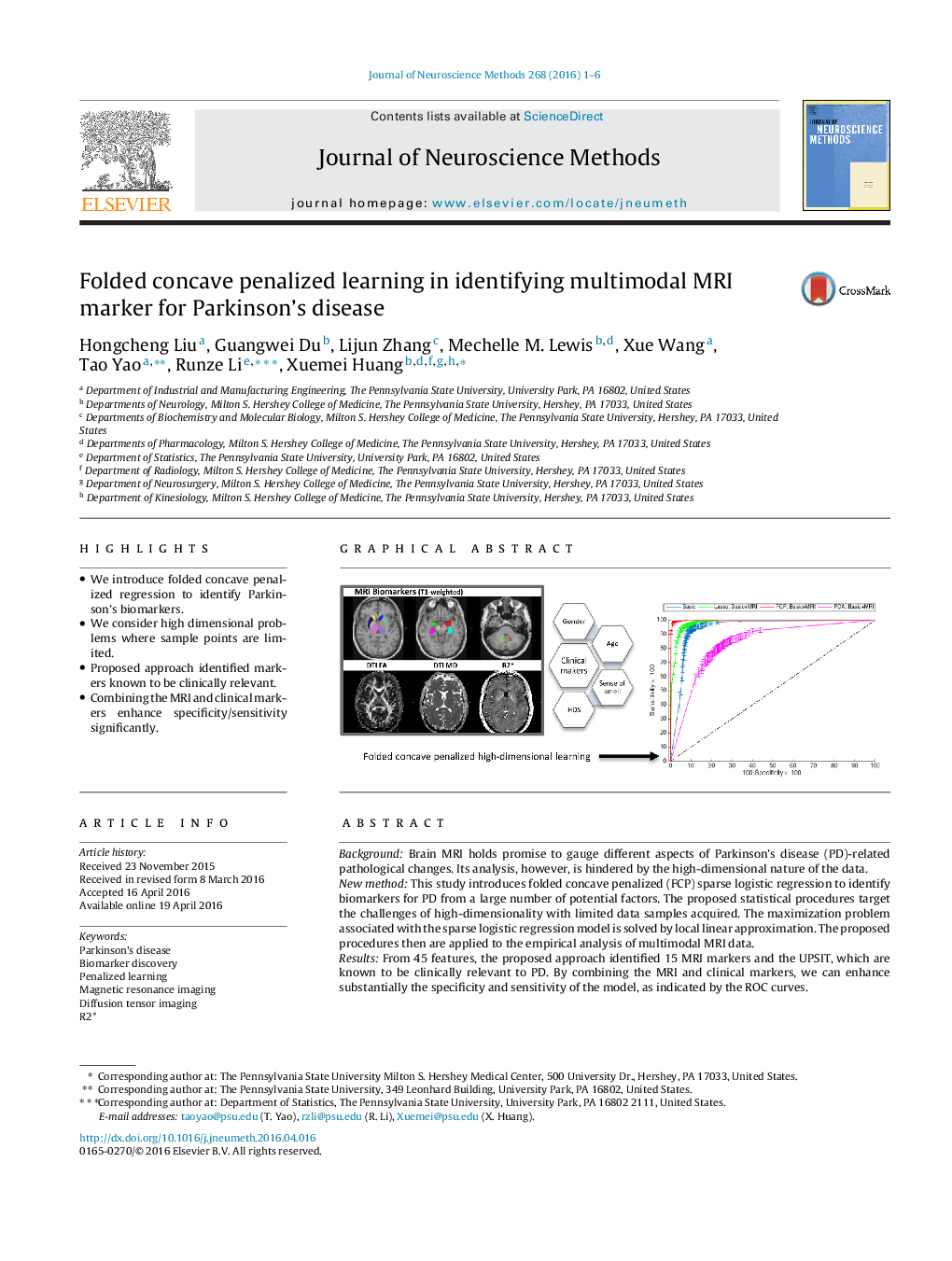
- We introduce folded concave penalized regression to identify Parkinson's biomarkers.
- We consider high dimensional problems where sample points are limited.
- Proposed approach identified markers known to be clinically relevant.
- Combining the MRI and clinical markers enhance specificity/sensitivity significantly.
BackgroundBrain MRI holds promise to gauge different aspects of Parkinson's disease (PD)-related pathological changes. Its analysis, however, is hindered by the high-dimensional nature of the data.New methodThis study introduces folded concave penalized (FCP) sparse logistic regression to identify biomarkers for PD from a large number of potential factors. The proposed statistical procedures target the challenges of high-dimensionality with limited data samples acquired. The maximization problem associated with the sparse logistic regression model is solved by local linear approximation. The proposed procedures then are applied to the empirical analysis of multimodal MRI data.ResultsFrom 45 features, the proposed approach identified 15 MRI markers and the UPSIT, which are known to be clinically relevant to PD. By combining the MRI and clinical markers, we can enhance substantially the specificity and sensitivity of the model, as indicated by the ROC curves.Comparison to existing methodsWe compare the folded concave penalized learning scheme with both the Lasso penalized scheme and the principle component analysis-based feature selection (PCA) in the Parkinson's biomarker identification problem that takes into account both the clinical features and MRI markers. The folded concave penalty method demonstrates a substantially better clinical potential than both the Lasso and PCA in terms of specificity and sensitivity.ConclusionsFor the first time, we applied the FCP learning method to MRI biomarker discovery in PD. The proposed approach successfully identified MRI markers that are clinically relevant. Combining these biomarkers with clinical features can substantially enhance performance.
240
Journal: Journal of Neuroscience Methods - Volume 268, 1 August 2016, Pages 1-6