کد مقاله | کد نشریه | سال انتشار | مقاله انگلیسی | نسخه تمام متن |
---|---|---|---|---|
6268265 | 1614624 | 2015 | 9 صفحه PDF | دانلود رایگان |
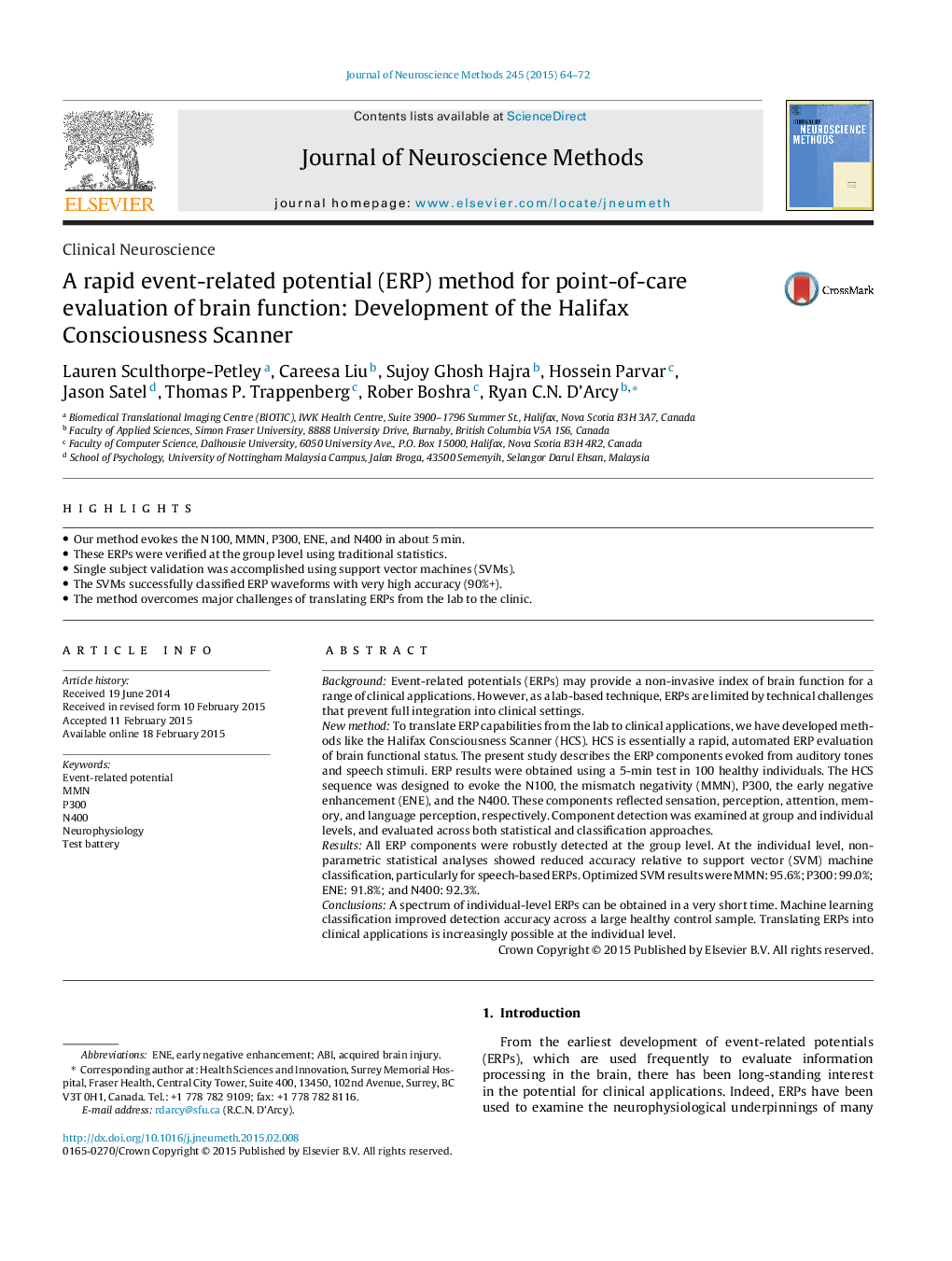
- Our method evokes the N100, MMN, P300, ENE, and N400 in about 5Â min.
- These ERPs were verified at the group level using traditional statistics.
- Single subject validation was accomplished using support vector machines (SVMs).
- The SVMs successfully classified ERP waveforms with very high accuracy (90%+).
- The method overcomes major challenges of translating ERPs from the lab to the clinic.
BackgroundEvent-related potentials (ERPs) may provide a non-invasive index of brain function for a range of clinical applications. However, as a lab-based technique, ERPs are limited by technical challenges that prevent full integration into clinical settings.New methodTo translate ERP capabilities from the lab to clinical applications, we have developed methods like the Halifax Consciousness Scanner (HCS). HCS is essentially a rapid, automated ERP evaluation of brain functional status. The present study describes the ERP components evoked from auditory tones and speech stimuli. ERP results were obtained using a 5-min test in 100 healthy individuals. The HCS sequence was designed to evoke the N100, the mismatch negativity (MMN), P300, the early negative enhancement (ENE), and the N400. These components reflected sensation, perception, attention, memory, and language perception, respectively. Component detection was examined at group and individual levels, and evaluated across both statistical and classification approaches.ResultsAll ERP components were robustly detected at the group level. At the individual level, nonparametric statistical analyses showed reduced accuracy relative to support vector (SVM) machine classification, particularly for speech-based ERPs. Optimized SVM results were MMN: 95.6%; P300: 99.0%; ENE: 91.8%; and N400: 92.3%.ConclusionsA spectrum of individual-level ERPs can be obtained in a very short time. Machine learning classification improved detection accuracy across a large healthy control sample. Translating ERPs into clinical applications is increasingly possible at the individual level.
Journal: Journal of Neuroscience Methods - Volume 245, 30 April 2015, Pages 64-72