کد مقاله | کد نشریه | سال انتشار | مقاله انگلیسی | نسخه تمام متن |
---|---|---|---|---|
6268438 | 1614629 | 2015 | 9 صفحه PDF | دانلود رایگان |
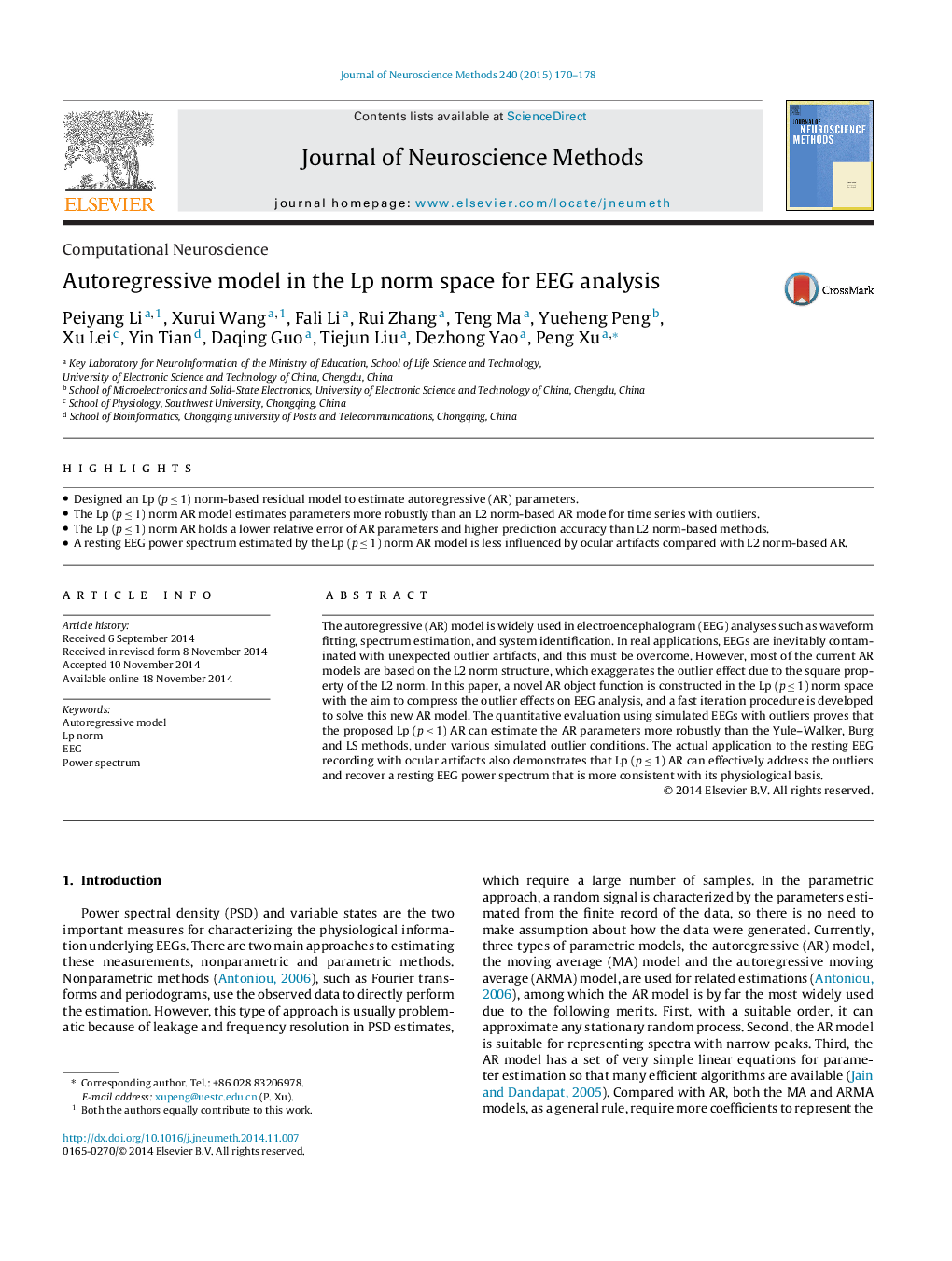
- Designed an Lp (p â¤Â 1) norm-based residual model to estimate autoregressive (AR) parameters.
- The Lp (p â¤Â 1) norm AR model estimates parameters more robustly than an L2 norm-based AR mode for time series with outliers.
- The Lp (p â¤Â 1) norm AR holds a lower relative error of AR parameters and higher prediction accuracy than L2 norm-based methods.
- A resting EEG power spectrum estimated by the Lp (p â¤Â 1) norm AR model is less influenced by ocular artifacts compared with L2 norm-based AR.
The autoregressive (AR) model is widely used in electroencephalogram (EEG) analyses such as waveform fitting, spectrum estimation, and system identification. In real applications, EEGs are inevitably contaminated with unexpected outlier artifacts, and this must be overcome. However, most of the current AR models are based on the L2 norm structure, which exaggerates the outlier effect due to the square property of the L2 norm. In this paper, a novel AR object function is constructed in the Lp (p â¤Â 1) norm space with the aim to compress the outlier effects on EEG analysis, and a fast iteration procedure is developed to solve this new AR model. The quantitative evaluation using simulated EEGs with outliers proves that the proposed Lp (p â¤Â 1) AR can estimate the AR parameters more robustly than the Yule-Walker, Burg and LS methods, under various simulated outlier conditions. The actual application to the resting EEG recording with ocular artifacts also demonstrates that Lp (p â¤Â 1) AR can effectively address the outliers and recover a resting EEG power spectrum that is more consistent with its physiological basis.
Journal: Journal of Neuroscience Methods - Volume 240, 30 January 2015, Pages 170-178