کد مقاله | کد نشریه | سال انتشار | مقاله انگلیسی | نسخه تمام متن |
---|---|---|---|---|
6268726 | 1614645 | 2014 | 13 صفحه PDF | دانلود رایگان |
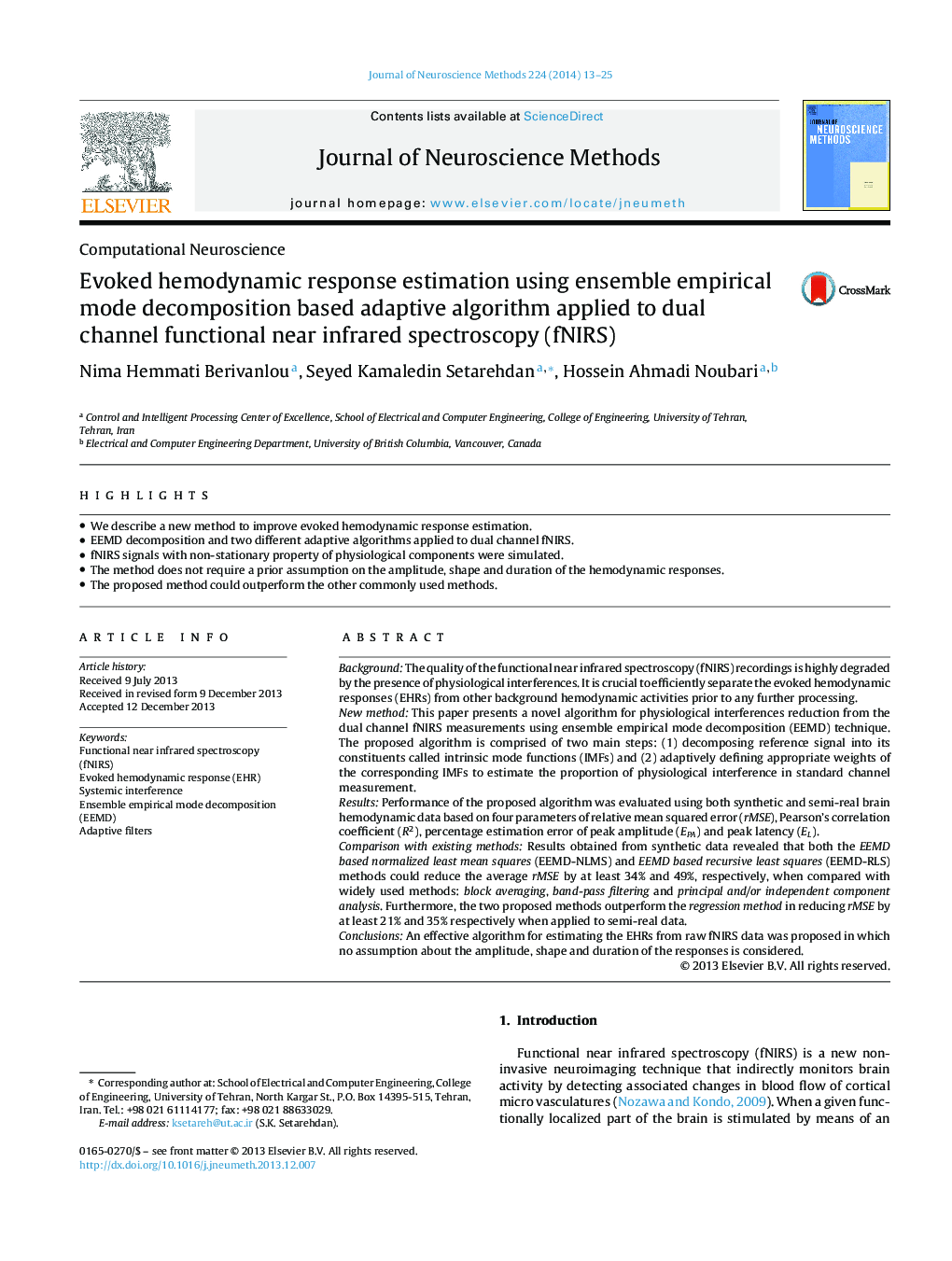
- We describe a new method to improve evoked hemodynamic response estimation.
- EEMD decomposition and two different adaptive algorithms applied to dual channel fNIRS.
- fNIRS signals with non-stationary property of physiological components were simulated.
- The method does not require a prior assumption on the amplitude, shape and duration of the hemodynamic responses.
- The proposed method could outperform the other commonly used methods.
BackgroundThe quality of the functional near infrared spectroscopy (fNIRS) recordings is highly degraded by the presence of physiological interferences. It is crucial to efficiently separate the evoked hemodynamic responses (EHRs) from other background hemodynamic activities prior to any further processing.New methodThis paper presents a novel algorithm for physiological interferences reduction from the dual channel fNIRS measurements using ensemble empirical mode decomposition (EEMD) technique. The proposed algorithm is comprised of two main steps: (1) decomposing reference signal into its constituents called intrinsic mode functions (IMFs) and (2) adaptively defining appropriate weights of the corresponding IMFs to estimate the proportion of physiological interference in standard channel measurement.ResultsPerformance of the proposed algorithm was evaluated using both synthetic and semi-real brain hemodynamic data based on four parameters of relative mean squared error (rMSE), Pearson's correlation coefficient (R2), percentage estimation error of peak amplitude (EPA) and peak latency (EL).Comparison with existing methodsResults obtained from synthetic data revealed that both the EEMD based normalized least mean squares (EEMD-NLMS) and EEMD based recursive least squares (EEMD-RLS) methods could reduce the average rMSE by at least 34% and 49%, respectively, when compared with widely used methods: block averaging, band-pass filtering and principal and/or independent component analysis. Furthermore, the two proposed methods outperform the regression method in reducing rMSE by at least 21% and 35% respectively when applied to semi-real data.ConclusionsAn effective algorithm for estimating the EHRs from raw fNIRS data was proposed in which no assumption about the amplitude, shape and duration of the responses is considered.
Journal: Journal of Neuroscience Methods - Volume 224, 15 March 2014, Pages 13-25