کد مقاله | کد نشریه | سال انتشار | مقاله انگلیسی | نسخه تمام متن |
---|---|---|---|---|
6268837 | 1614648 | 2014 | 8 صفحه PDF | دانلود رایگان |
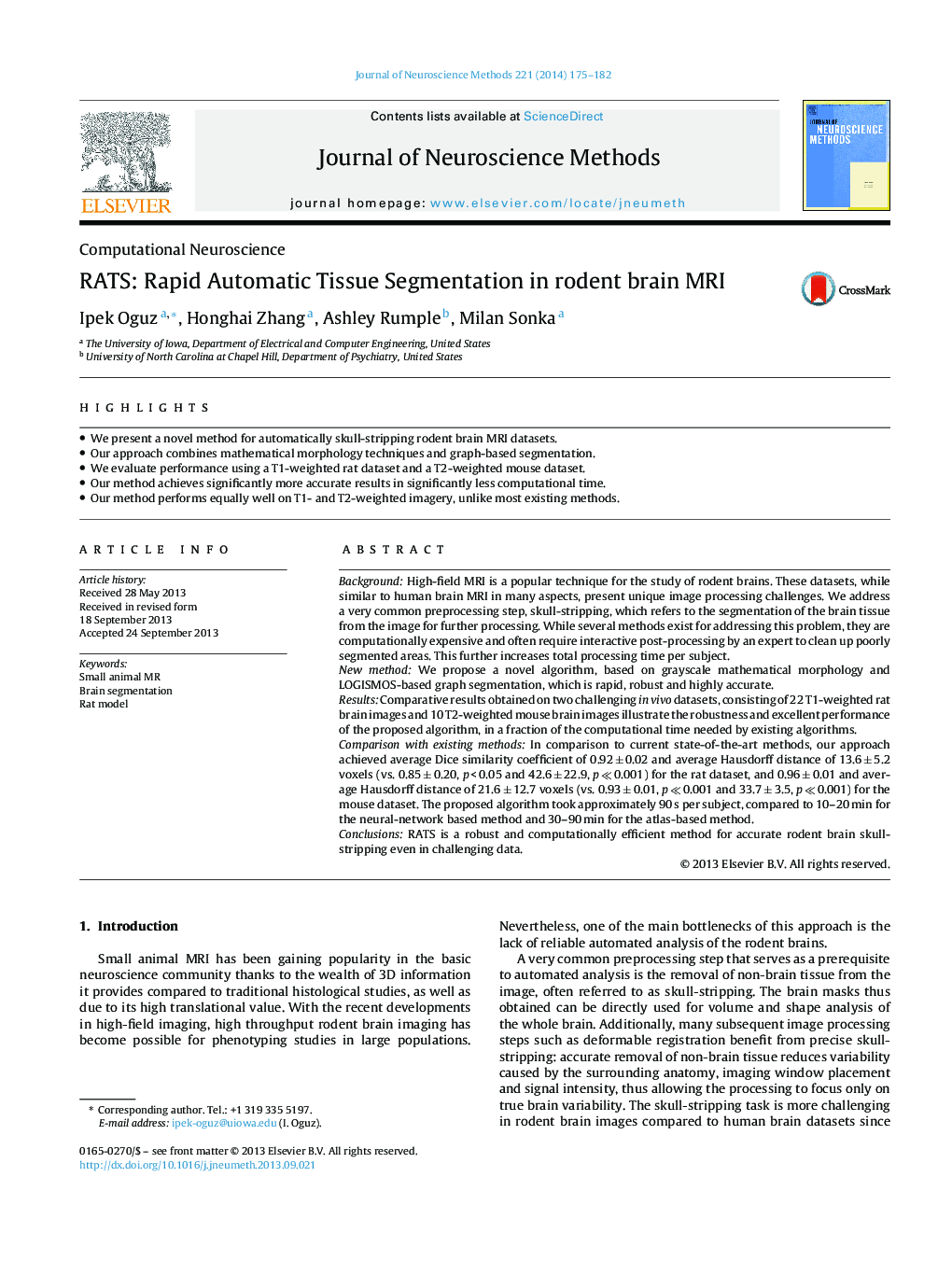
- We present a novel method for automatically skull-stripping rodent brain MRI datasets.
- Our approach combines mathematical morphology techniques and graph-based segmentation.
- We evaluate performance using a T1-weighted rat dataset and a T2-weighted mouse dataset.
- Our method achieves significantly more accurate results in significantly less computational time.
- Our method performs equally well on T1- and T2-weighted imagery, unlike most existing methods.
BackgroundHigh-field MRI is a popular technique for the study of rodent brains. These datasets, while similar to human brain MRI in many aspects, present unique image processing challenges. We address a very common preprocessing step, skull-stripping, which refers to the segmentation of the brain tissue from the image for further processing. While several methods exist for addressing this problem, they are computationally expensive and often require interactive post-processing by an expert to clean up poorly segmented areas. This further increases total processing time per subject.New methodWe propose a novel algorithm, based on grayscale mathematical morphology and LOGISMOS-based graph segmentation, which is rapid, robust and highly accurate.ResultsComparative results obtained on two challenging in vivo datasets, consisting of 22 T1-weighted rat brain images and 10 T2-weighted mouse brain images illustrate the robustness and excellent performance of the proposed algorithm, in a fraction of the computational time needed by existing algorithms.Comparison with existing methodsIn comparison to current state-of-the-art methods, our approach achieved average Dice similarity coefficient of 0.92 ± 0.02 and average Hausdorff distance of 13.6 ± 5.2 voxels (vs. 0.85 ± 0.20, p < 0.05 and 42.6 ± 22.9, p âªÂ 0.001) for the rat dataset, and 0.96 ± 0.01 and average Hausdorff distance of 21.6 ± 12.7 voxels (vs. 0.93 ± 0.01, p âªÂ 0.001 and 33.7 ± 3.5, p âªÂ 0.001) for the mouse dataset. The proposed algorithm took approximately 90 s per subject, compared to 10-20 min for the neural-network based method and 30-90 min for the atlas-based method.ConclusionsRATS is a robust and computationally efficient method for accurate rodent brain skull-stripping even in challenging data.
Journal: Journal of Neuroscience Methods - Volume 221, 15 January 2014, Pages 175-182