کد مقاله | کد نشریه | سال انتشار | مقاله انگلیسی | نسخه تمام متن |
---|---|---|---|---|
6369953 | 1623841 | 2015 | 10 صفحه PDF | دانلود رایگان |
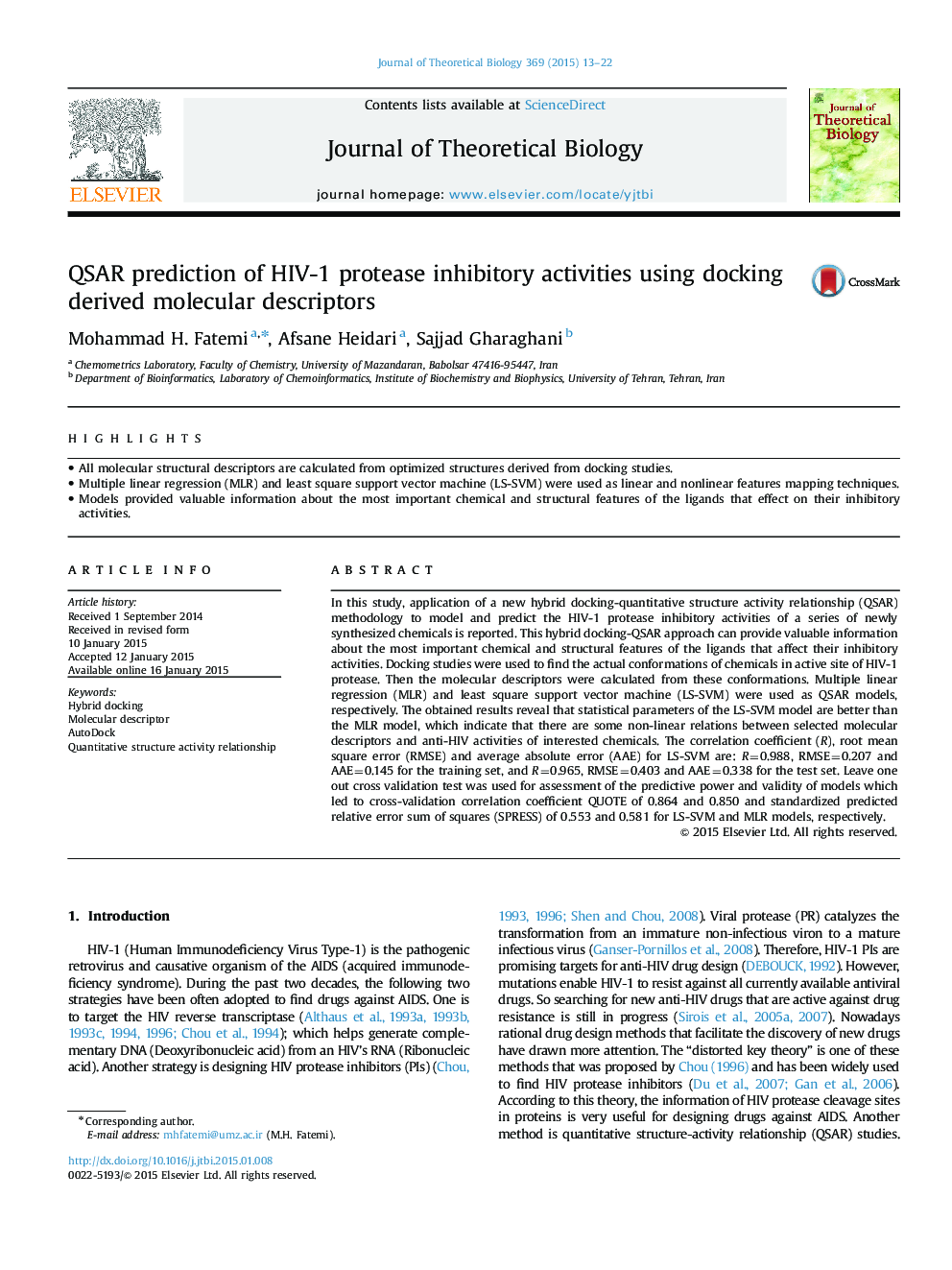
- All molecular structural descriptors are calculated from optimized structures derived from docking studies.
- Multiple linear regression (MLR) and least square support vector machine (LS-SVM) were used as linear and nonlinear features mapping techniques.
- Models provided valuable information about the most important chemical and structural features of the ligands that effect on their inhibitory activities.
In this study, application of a new hybrid docking-quantitative structure activity relationship (QSAR) methodology to model and predict the HIV-1 protease inhibitory activities of a series of newly synthesized chemicals is reported. This hybrid docking-QSAR approach can provide valuable information about the most important chemical and structural features of the ligands that affect their inhibitory activities. Docking studies were used to find the actual conformations of chemicals in active site of HIV-1 protease. Then the molecular descriptors were calculated from these conformations. Multiple linear regression (MLR) and least square support vector machine (LS-SVM) were used as QSAR models, respectively. The obtained results reveal that statistical parameters of the LS-SVM model are better than the MLR model, which indicate that there are some non-linear relations between selected molecular descriptors and anti-HIV activities of interested chemicals. The correlation coefficient (R), root mean square error (RMSE) and average absolute error (AAE) for LS-SVM are: R=0.988, RMSE=0.207 and AAE=0.145 for the training set, and R=0.965, RMSE=0.403 and AAE=0.338 for the test set. Leave one out cross validation test was used for assessment of the predictive power and validity of models which led to cross-validation correlation coefficient QUOTE of 0.864 and 0.850 and standardized predicted relative error sum of squares (SPRESS) of 0.553 and 0.581 for LS-SVM and MLR models, respectively.
Journal: Journal of Theoretical Biology - Volume 369, 21 March 2015, Pages 13-22