کد مقاله | کد نشریه | سال انتشار | مقاله انگلیسی | نسخه تمام متن |
---|---|---|---|---|
6679916 | 1428065 | 2018 | 14 صفحه PDF | دانلود رایگان |
عنوان انگلیسی مقاله ISI
Geologic CO2 sequestration monitoring design: A machine learning and uncertainty quantification based approach
دانلود مقاله + سفارش ترجمه
دانلود مقاله ISI انگلیسی
رایگان برای ایرانیان
کلمات کلیدی
موضوعات مرتبط
مهندسی و علوم پایه
مهندسی انرژی
مهندسی انرژی و فناوری های برق
پیش نمایش صفحه اول مقاله
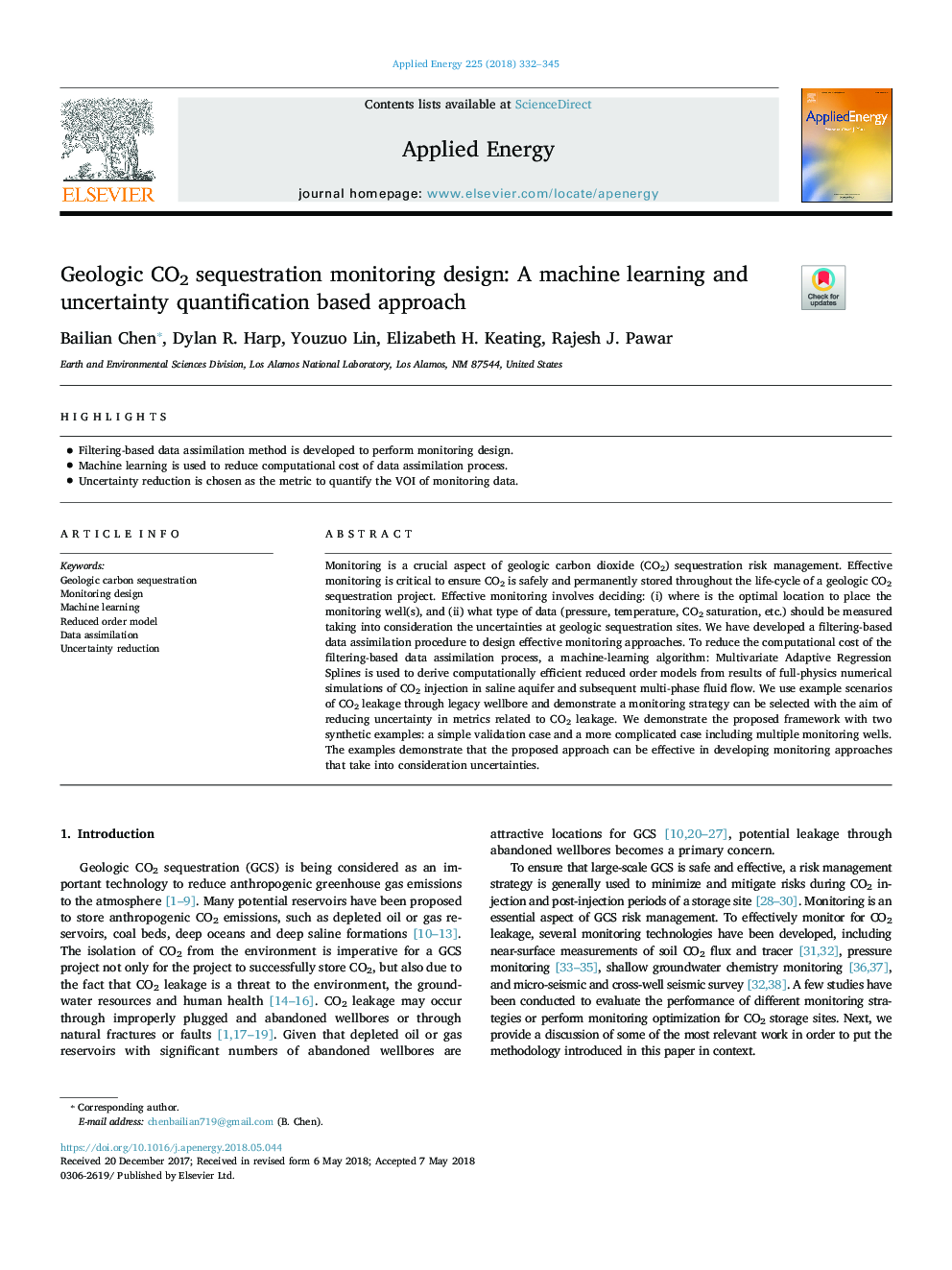
چکیده انگلیسی
Monitoring is a crucial aspect of geologic carbon dioxide (CO2) sequestration risk management. Effective monitoring is critical to ensure CO2 is safely and permanently stored throughout the life-cycle of a geologic CO2 sequestration project. Effective monitoring involves deciding: (i) where is the optimal location to place the monitoring well(s), and (ii) what type of data (pressure, temperature, CO2 saturation, etc.) should be measured taking into consideration the uncertainties at geologic sequestration sites. We have developed a filtering-based data assimilation procedure to design effective monitoring approaches. To reduce the computational cost of the filtering-based data assimilation process, a machine-learning algorithm: Multivariate Adaptive Regression Splines is used to derive computationally efficient reduced order models from results of full-physics numerical simulations of CO2 injection in saline aquifer and subsequent multi-phase fluid flow. We use example scenarios of CO2 leakage through legacy wellbore and demonstrate a monitoring strategy can be selected with the aim of reducing uncertainty in metrics related to CO2 leakage. We demonstrate the proposed framework with two synthetic examples: a simple validation case and a more complicated case including multiple monitoring wells. The examples demonstrate that the proposed approach can be effective in developing monitoring approaches that take into consideration uncertainties.
ناشر
Database: Elsevier - ScienceDirect (ساینس دایرکت)
Journal: Applied Energy - Volume 225, 1 September 2018, Pages 332-345
Journal: Applied Energy - Volume 225, 1 September 2018, Pages 332-345
نویسندگان
Bailian Chen, Dylan R. Harp, Youzuo Lin, Elizabeth H. Keating, Rajesh J. Pawar,