کد مقاله | کد نشریه | سال انتشار | مقاله انگلیسی | نسخه تمام متن |
---|---|---|---|---|
6757035 | 1431092 | 2018 | 11 صفحه PDF | دانلود رایگان |
عنوان انگلیسی مقاله ISI
A novel wind speed prediction method: Hybrid of correlation-aided DWT, LSSVM and GARCH
دانلود مقاله + سفارش ترجمه
دانلود مقاله ISI انگلیسی
رایگان برای ایرانیان
کلمات کلیدی
موضوعات مرتبط
مهندسی و علوم پایه
مهندسی انرژی
انرژی های تجدید پذیر، توسعه پایدار و محیط زیست
پیش نمایش صفحه اول مقاله
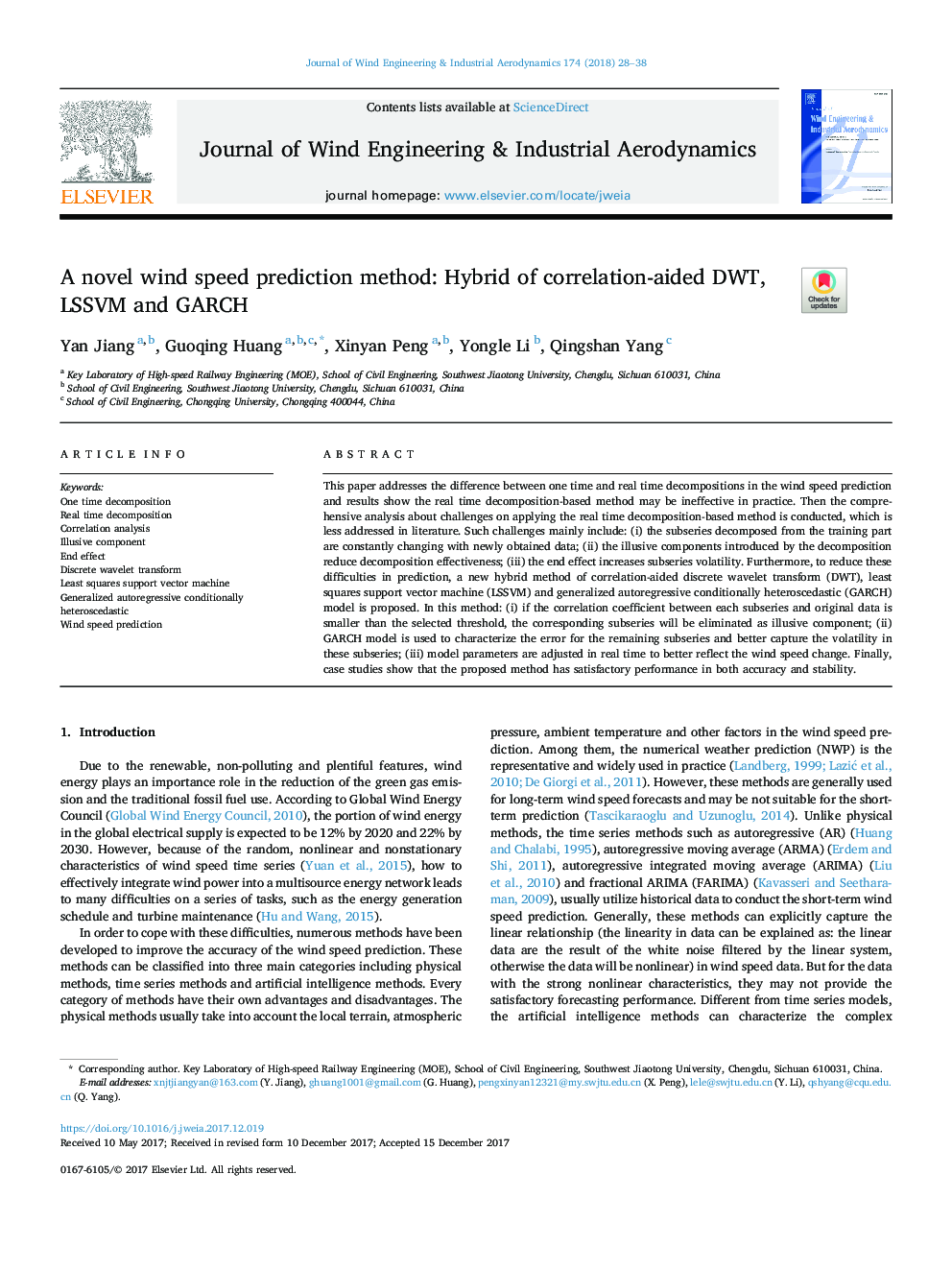
چکیده انگلیسی
This paper addresses the difference between one time and real time decompositions in the wind speed prediction and results show the real time decomposition-based method may be ineffective in practice. Then the comprehensive analysis about challenges on applying the real time decomposition-based method is conducted, which is less addressed in literature. Such challenges mainly include: (i) the subseries decomposed from the training part are constantly changing with newly obtained data; (ii) the illusive components introduced by the decomposition reduce decomposition effectiveness; (iii) the end effect increases subseries volatility. Furthermore, to reduce these difficulties in prediction, a new hybrid method of correlation-aided discrete wavelet transform (DWT), least squares support vector machine (LSSVM) and generalized autoregressive conditionally heteroscedastic (GARCH) model is proposed. In this method: (i) if the correlation coefficient between each subseries and original data is smaller than the selected threshold, the corresponding subseries will be eliminated as illusive component; (ii) GARCH model is used to characterize the error for the remaining subseries and better capture the volatility in these subseries; (iii) model parameters are adjusted in real time to better reflect the wind speed change. Finally, case studies show that the proposed method has satisfactory performance in both accuracy and stability.
ناشر
Database: Elsevier - ScienceDirect (ساینس دایرکت)
Journal: Journal of Wind Engineering and Industrial Aerodynamics - Volume 174, March 2018, Pages 28-38
Journal: Journal of Wind Engineering and Industrial Aerodynamics - Volume 174, March 2018, Pages 28-38
نویسندگان
Yan Jiang, Guoqing Huang, Xinyan Peng, Yongle Li, Qingshan Yang,