کد مقاله | کد نشریه | سال انتشار | مقاله انگلیسی | نسخه تمام متن |
---|---|---|---|---|
6854661 | 1437592 | 2018 | 11 صفحه PDF | دانلود رایگان |
عنوان انگلیسی مقاله ISI
ECG classification using three-level fusion of different feature descriptors
دانلود مقاله + سفارش ترجمه
دانلود مقاله ISI انگلیسی
رایگان برای ایرانیان
کلمات کلیدی
موضوعات مرتبط
مهندسی و علوم پایه
مهندسی کامپیوتر
هوش مصنوعی
پیش نمایش صفحه اول مقاله
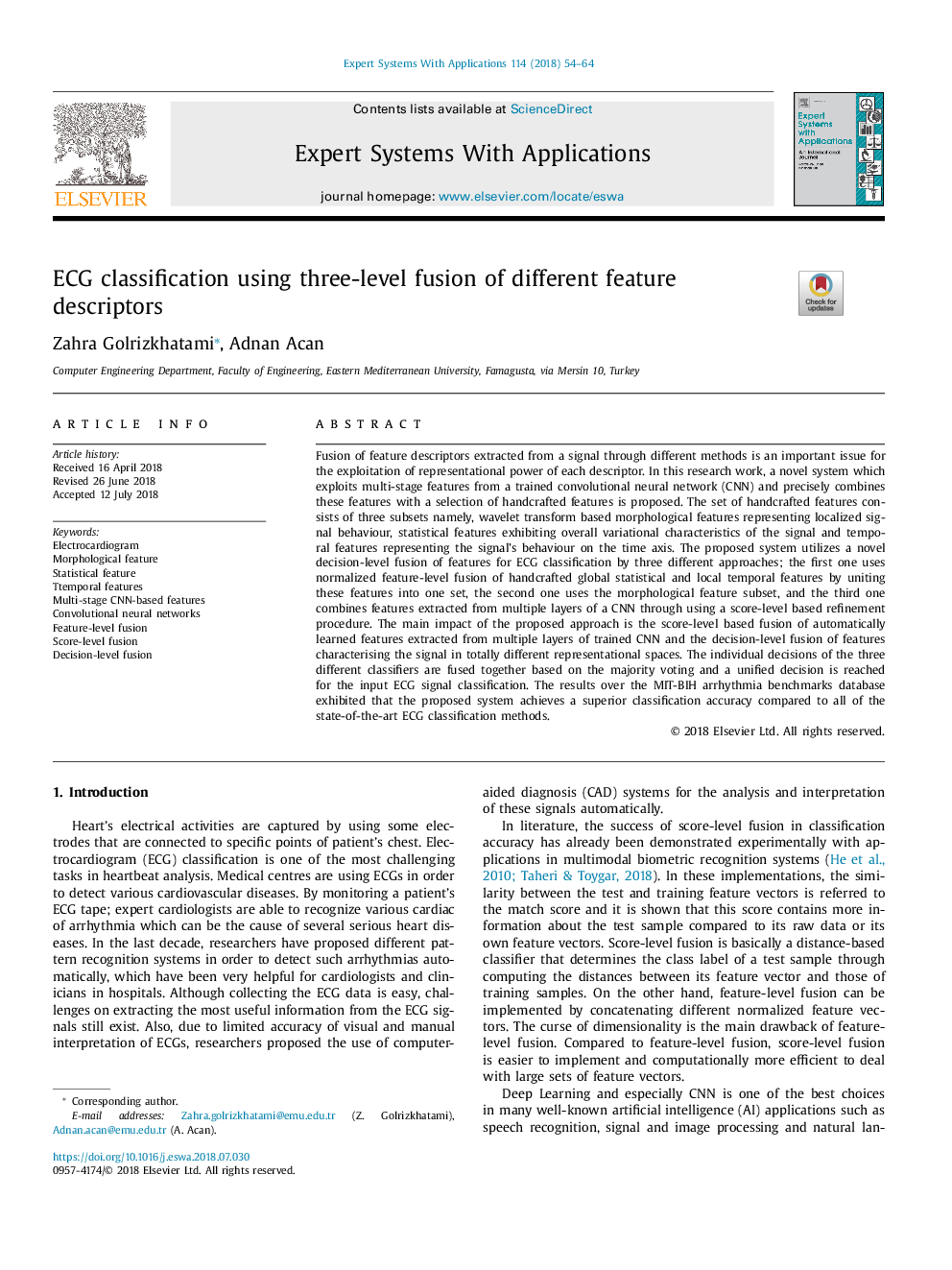
چکیده انگلیسی
Fusion of feature descriptors extracted from a signal through different methods is an important issue for the exploitation of representational power of each descriptor. In this research work, a novel system which exploits multi-stage features from a trained convolutional neural network (CNN) and precisely combines these features with a selection of handcrafted features is proposed. The set of handcrafted features consists of three subsets namely, wavelet transform based morphological features representing localized signal behaviour, statistical features exhibiting overall variational characteristics of the signal and temporal features representing the signal's behaviour on the time axis. The proposed system utilizes a novel decision-level fusion of features for ECG classification by three different approaches; the first one uses normalized feature-level fusion of handcrafted global statistical and local temporal features by uniting these features into one set, the second one uses the morphological feature subset, and the third one combines features extracted from multiple layers of a CNN through using a score-level based refinement procedure. The main impact of the proposed approach is the score-level based fusion of automatically learned features extracted from multiple layers of trained CNN and the decision-level fusion of features characterising the signal in totally different representational spaces. The individual decisions of the three different classifiers are fused together based on the majority voting and a unified decision is reached for the input ECG signal classification. The results over the MIT-BIH arrhythmia benchmarks database exhibited that the proposed system achieves a superior classification accuracy compared to all of the state-of-the-art ECG classification methods.
ناشر
Database: Elsevier - ScienceDirect (ساینس دایرکت)
Journal: Expert Systems with Applications - Volume 114, 30 December 2018, Pages 54-64
Journal: Expert Systems with Applications - Volume 114, 30 December 2018, Pages 54-64
نویسندگان
Zahra Golrizkhatami, Adnan Acan,