کد مقاله | کد نشریه | سال انتشار | مقاله انگلیسی | نسخه تمام متن |
---|---|---|---|---|
6854826 | 1437596 | 2018 | 8 صفحه PDF | دانلود رایگان |
عنوان انگلیسی مقاله ISI
Curriculum learning based approach for noise robust language identification using DNN with attention
دانلود مقاله + سفارش ترجمه
دانلود مقاله ISI انگلیسی
رایگان برای ایرانیان
موضوعات مرتبط
مهندسی و علوم پایه
مهندسی کامپیوتر
هوش مصنوعی
پیش نمایش صفحه اول مقاله
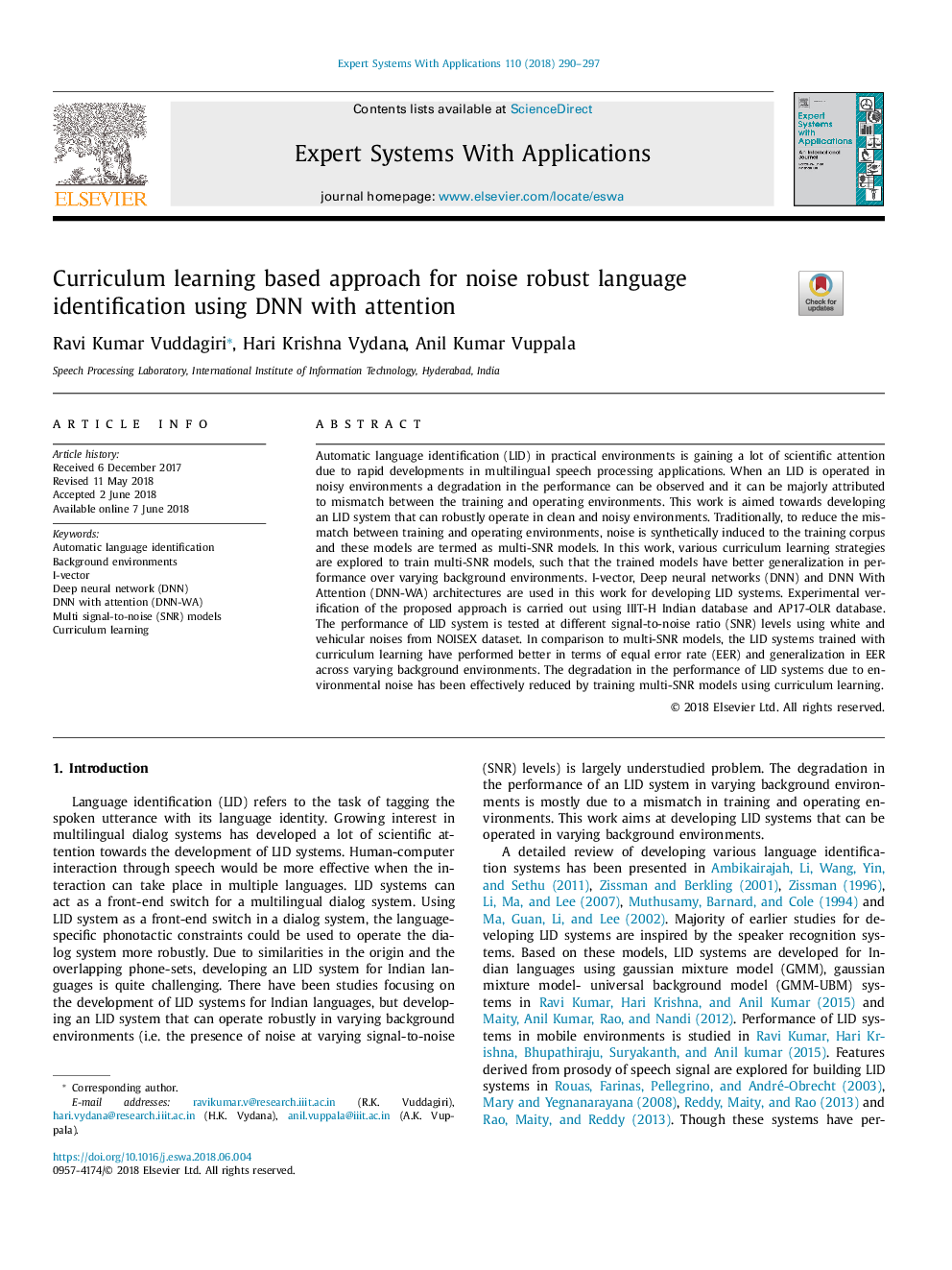
چکیده انگلیسی
Automatic language identification (LID) in practical environments is gaining a lot of scientific attention due to rapid developments in multilingual speech processing applications. When an LID is operated in noisy environments a degradation in the performance can be observed and it can be majorly attributed to mismatch between the training and operating environments. This work is aimed towards developing an LID system that can robustly operate in clean and noisy environments. Traditionally, to reduce the mismatch between training and operating environments, noise is synthetically induced to the training corpus and these models are termed as multi-SNR models. In this work, various curriculum learning strategies are explored to train multi-SNR models, such that the trained models have better generalization in performance over varying background environments. I-vector, Deep neural networks (DNN) and DNN With Attention (DNN-WA) architectures are used in this work for developing LID systems. Experimental verification of the proposed approach is carried out using IIIT-H Indian database and AP17-OLR database. The performance of LID system is tested at different signal-to-noise ratio (SNR) levels using white and vehicular noises from NOISEX dataset. In comparison to multi-SNR models, the LID systems trained with curriculum learning have performed better in terms of equal error rate (EER) and generalization in EER across varying background environments. The degradation in the performance of LID systems due to environmental noise has been effectively reduced by training multi-SNR models using curriculum learning.
ناشر
Database: Elsevier - ScienceDirect (ساینس دایرکت)
Journal: Expert Systems with Applications - Volume 110, 15 November 2018, Pages 290-297
Journal: Expert Systems with Applications - Volume 110, 15 November 2018, Pages 290-297
نویسندگان
Ravi Kumar Vuddagiri, Hari Krishna Vydana, Anil Kumar Vuppala,