کد مقاله | کد نشریه | سال انتشار | مقاله انگلیسی | نسخه تمام متن |
---|---|---|---|---|
6855185 | 1437609 | 2018 | 20 صفحه PDF | دانلود رایگان |
عنوان انگلیسی مقاله ISI
Categorizing paintings in art styles based on qualitative color descriptors, quantitative global features and machine learning (QArt-Learn)
دانلود مقاله + سفارش ترجمه
دانلود مقاله ISI انگلیسی
رایگان برای ایرانیان
کلمات کلیدی
موضوعات مرتبط
مهندسی و علوم پایه
مهندسی کامپیوتر
هوش مصنوعی
پیش نمایش صفحه اول مقاله
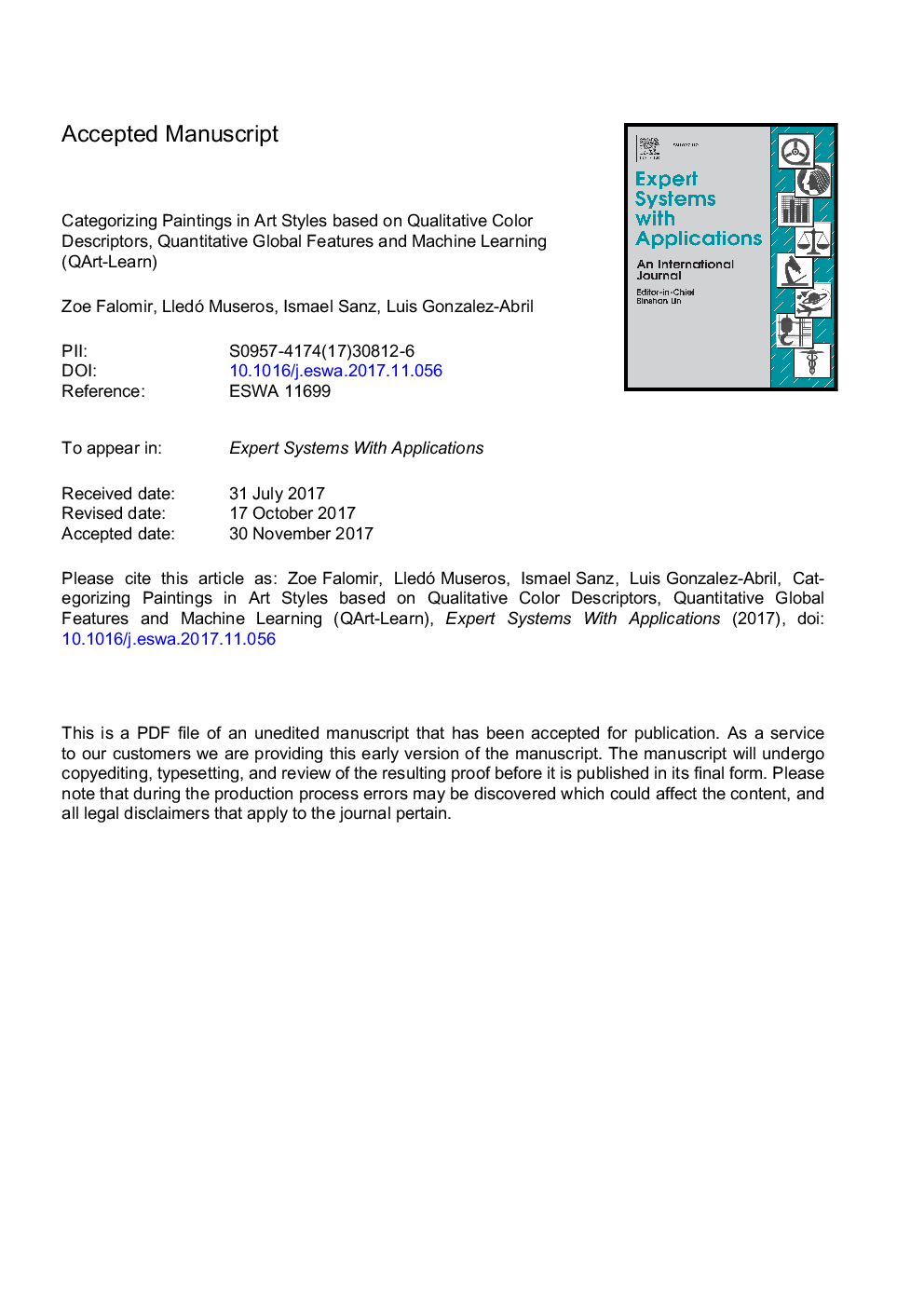
چکیده انگلیسی
The QArt-Learn approach for style painting categorization based on Qualitative Color Descriptors (QCD), color similarity (SimQCD), and quantitative global features (i.e. average of brightness, hue, saturation and lightness and brightness contrast) is presented in this paper. k-Nearest Neighbor (k-NN) and support vector machine (SVM) techniques have been used for learning the features of paintings from the Baroque, Impressionism and Post-Impressionism styles. Specifically two classifiers are built, and two different parameterizations have been applied for the QCD. For testing QArt-Learn approach, the Painting-91 dataset has been used, from which the paintings corresponding to Velázquez, Vermeer, Monet, Renoir, van Gogh and Gauguin were extracted, resulting in a set of 252 paintings. The results obtained have shown categorization accuracies higher than 65%, which are comparable to accuracies obtained in the literature. However, QArt-Learn uses qualitative color names which can describe style color palettes linguistically, so that they can be better understood by non-experts in art since QCDs are aligned with human perception.
ناشر
Database: Elsevier - ScienceDirect (ساینس دایرکت)
Journal: Expert Systems with Applications - Volume 97, 1 May 2018, Pages 83-94
Journal: Expert Systems with Applications - Volume 97, 1 May 2018, Pages 83-94
نویسندگان
Zoe Falomir, Lledó Museros, Ismael Sanz, Luis Gonzalez-Abril,