کد مقاله | کد نشریه | سال انتشار | مقاله انگلیسی | نسخه تمام متن |
---|---|---|---|---|
6863634 | 1439516 | 2018 | 46 صفحه PDF | دانلود رایگان |
عنوان انگلیسی مقاله ISI
Non-convex weighted âp nuclear norm based ADMM framework for image restoration
دانلود مقاله + سفارش ترجمه
دانلود مقاله ISI انگلیسی
رایگان برای ایرانیان
کلمات کلیدی
موضوعات مرتبط
مهندسی و علوم پایه
مهندسی کامپیوتر
هوش مصنوعی
پیش نمایش صفحه اول مقاله
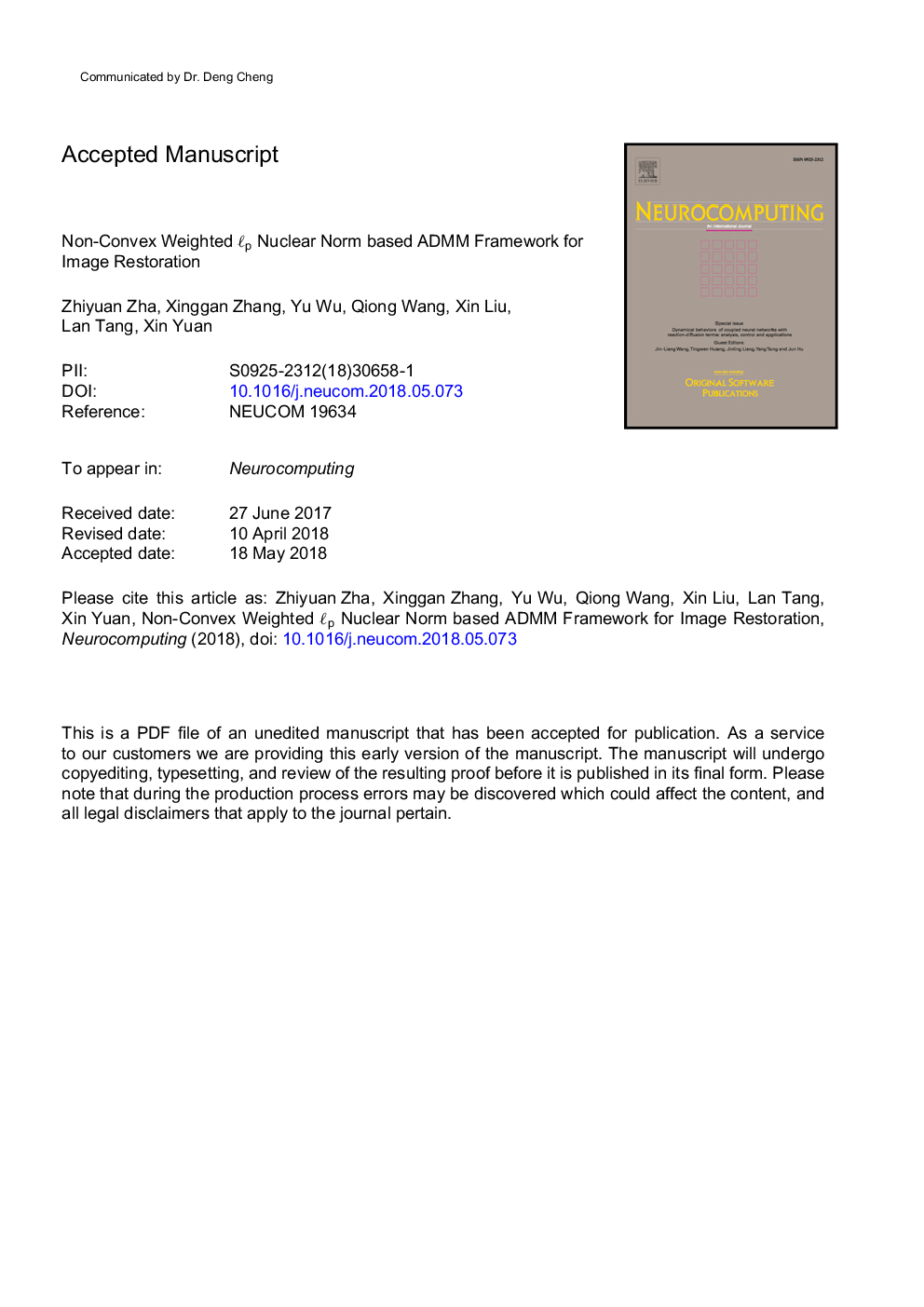
چکیده انگلیسی
Inspired by the fact that the matrix formed by nonlocal similar patches in a natural image is of low rank, the nuclear norm minimization (NNM) has been widely used in various image processing studies. Nonetheless, nuclear norm based convex surrogate of the rank function usually over-shrinks the rank components since it treats different components equally, and thus may produce a result far from the optimum. To alleviate the aforementioned limitations of the nuclear norm, in this paper we propose a new method for image restoration via the non-convex weighted âp nuclear norm minimization (NCW-NNM), which is able to accurately impose the image structural sparsity and self-similarity simultaneously. To make the proposed model tractable and robust, the alternating direction method of multiplier (ADMM) framework is adopted to solve the associated non-convex minimization problem. Experimental results on various image restoration problems, including image deblurring, image inpainting and image compressive sensing (CS) recovery, demonstrate that the proposed method outperforms many current state-of-the-art methods.
ناشر
Database: Elsevier - ScienceDirect (ساینس دایرکت)
Journal: Neurocomputing - Volume 311, 15 October 2018, Pages 209-224
Journal: Neurocomputing - Volume 311, 15 October 2018, Pages 209-224
نویسندگان
Zhiyuan Zha, Xinggan Zhang, Yu Wu, Qiong Wang, Xin Liu, Lan Tang, Xin Yuan,