کد مقاله | کد نشریه | سال انتشار | مقاله انگلیسی | نسخه تمام متن |
---|---|---|---|---|
6863774 | 1439522 | 2018 | 38 صفحه PDF | دانلود رایگان |
عنوان انگلیسی مقاله ISI
Supervised Dirichlet Process Mixtures of Principal Component Analysis
دانلود مقاله + سفارش ترجمه
دانلود مقاله ISI انگلیسی
رایگان برای ایرانیان
کلمات کلیدی
موضوعات مرتبط
مهندسی و علوم پایه
مهندسی کامپیوتر
هوش مصنوعی
پیش نمایش صفحه اول مقاله
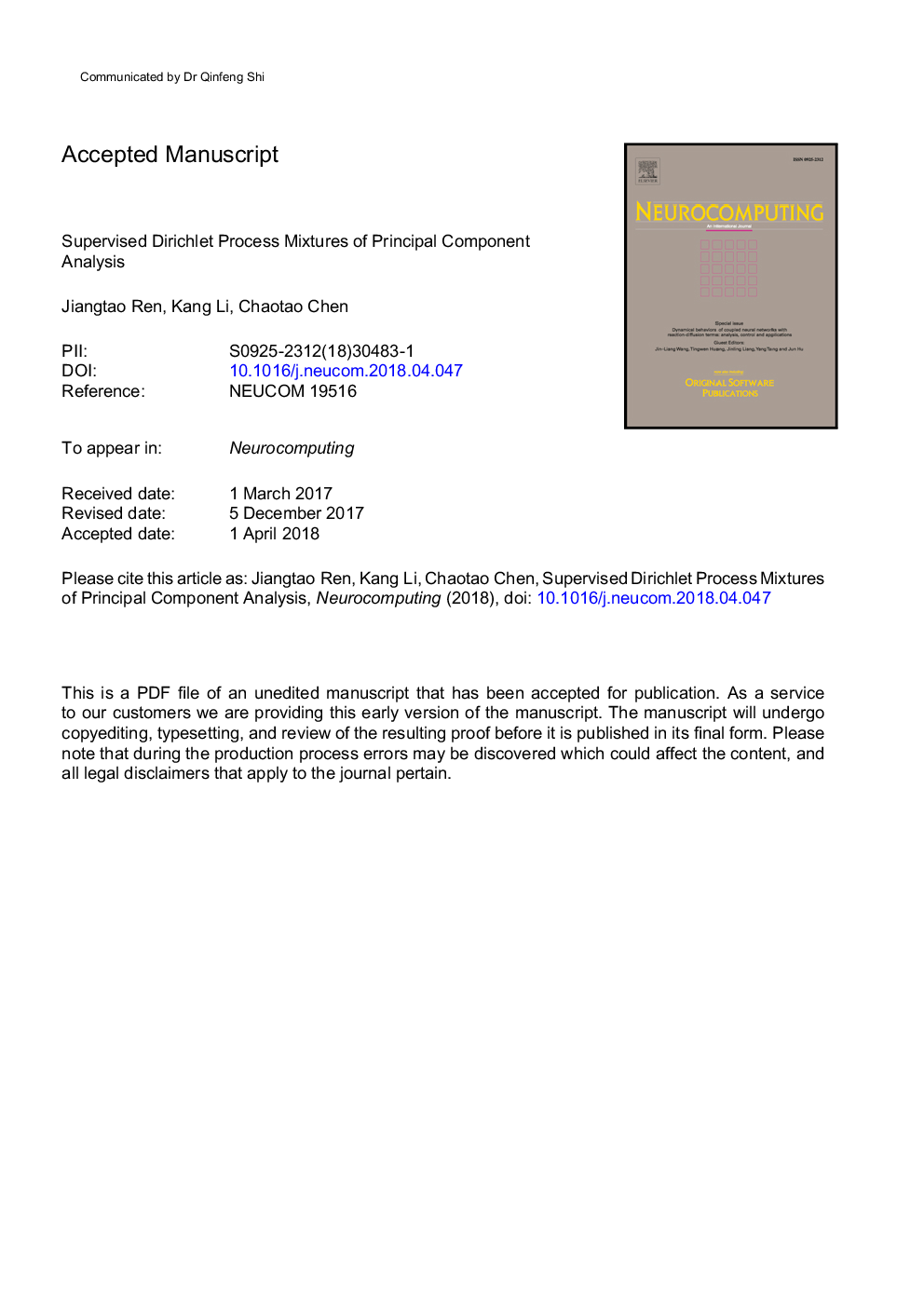
چکیده انگلیسی
We introduce probabilistic principal component analysis (PPCA) into Dirichlet Process Mixtures of Generalized Linear Models (DPGLM) and propose a new model called Supervised Dirichlet Process Mixtures of Principal Component Analysis (SDPM-PCA). In SDPM-PCA, we assume covariates and response variable are generated separately through the latent variable of PPCA, and nonparametrically modeled using the Dirichlet Process Mixture. By jointly learning the latent variable, cluster label and response variable, SDPM-PCA performs locally dimensionality reduction within each mixture component, and learns a supervised model based on the latent variable. In this way, SDPM-PCA improves the performance of both dimensionality reduction and prediction on high-dimensional data with all advantages of DPGLM. We also develop an inference algorithm for SDPM-PCA based on variational inference, which provides faster training speed and deterministic approximation compared with sampling algorithms based on MCMC method. Finally, we instantiate SDPM-PCA in regression problem with a Bayesian linear regression model. We test it on several real-world datasets and compare the prediction performance with DPGLM and other regular regression model. Experiment results show that by setting properly latent dimension number, SDPM-PCA would provide better prediction performance on high-dimensional regression problem and avoid the curse of dimensionality problem in DPGLM.
ناشر
Database: Elsevier - ScienceDirect (ساینس دایرکت)
Journal: Neurocomputing - Volume 305, 30 August 2018, Pages 15-26
Journal: Neurocomputing - Volume 305, 30 August 2018, Pages 15-26
نویسندگان
Jiangtao Ren, Kang Li, Chaotao Chen,