کد مقاله | کد نشریه | سال انتشار | مقاله انگلیسی | نسخه تمام متن |
---|---|---|---|---|
6864555 | 1439544 | 2018 | 9 صفحه PDF | دانلود رایگان |
عنوان انگلیسی مقاله ISI
Robust principal component analysis via optimal mean by joint â2,1 and Schatten p-norms minimization
دانلود مقاله + سفارش ترجمه
دانلود مقاله ISI انگلیسی
رایگان برای ایرانیان
کلمات کلیدی
موضوعات مرتبط
مهندسی و علوم پایه
مهندسی کامپیوتر
هوش مصنوعی
پیش نمایش صفحه اول مقاله
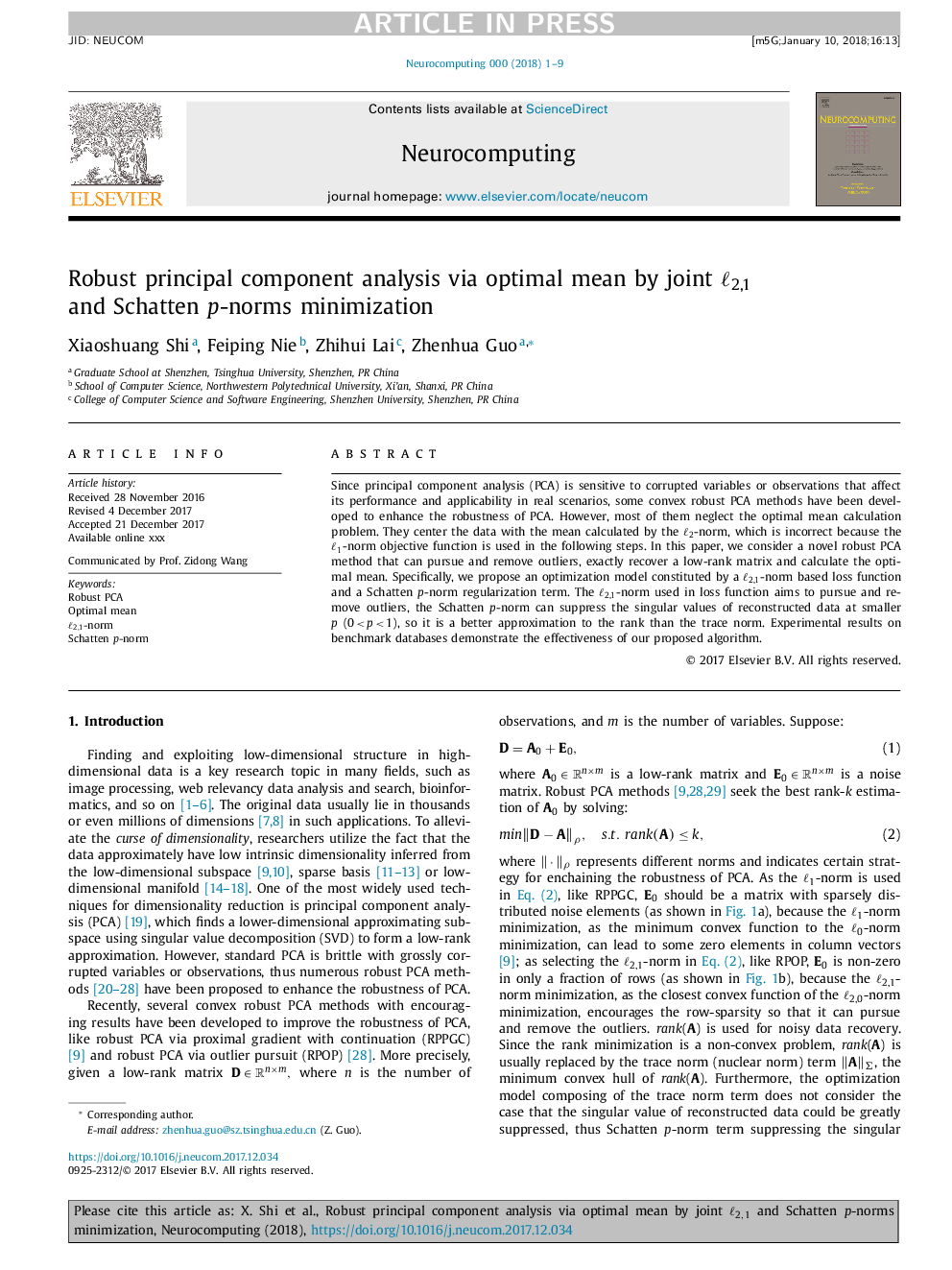
چکیده انگلیسی
Since principal component analysis (PCA) is sensitive to corrupted variables or observations that affect its performance and applicability in real scenarios, some convex robust PCA methods have been developed to enhance the robustness of PCA. However, most of them neglect the optimal mean calculation problem. They center the data with the mean calculated by the â2-norm, which is incorrect because the â1-norm objective function is used in the following steps. In this paper, we consider a novel robust PCA method that can pursue and remove outliers, exactly recover a low-rank matrix and calculate the optimal mean. Specifically, we propose an optimization model constituted by a â2,1-norm based loss function and a Schatten p-norm regularization term. The â2,1-norm used in loss function aims to pursue and remove outliers, the Schatten p-norm can suppress the singular values of reconstructed data at smaller p (0â¯<â¯pâ¯<â¯1), so it is a better approximation to the rank than the trace norm. Experimental results on benchmark databases demonstrate the effectiveness of our proposed algorithm.
ناشر
Database: Elsevier - ScienceDirect (ساینس دایرکت)
Journal: Neurocomputing - Volume 283, 29 March 2018, Pages 205-213
Journal: Neurocomputing - Volume 283, 29 March 2018, Pages 205-213
نویسندگان
Xiaoshuang Shi, Feiping Nie, Zhihui Lai, Zhenhua Guo,