کد مقاله | کد نشریه | سال انتشار | مقاله انگلیسی | نسخه تمام متن |
---|---|---|---|---|
6865669 | 678082 | 2015 | 9 صفحه PDF | دانلود رایگان |
عنوان انگلیسی مقاله ISI
Feature learning from incomplete EEG with denoising autoencoder
دانلود مقاله + سفارش ترجمه
دانلود مقاله ISI انگلیسی
رایگان برای ایرانیان
کلمات کلیدی
موضوعات مرتبط
مهندسی و علوم پایه
مهندسی کامپیوتر
هوش مصنوعی
پیش نمایش صفحه اول مقاله
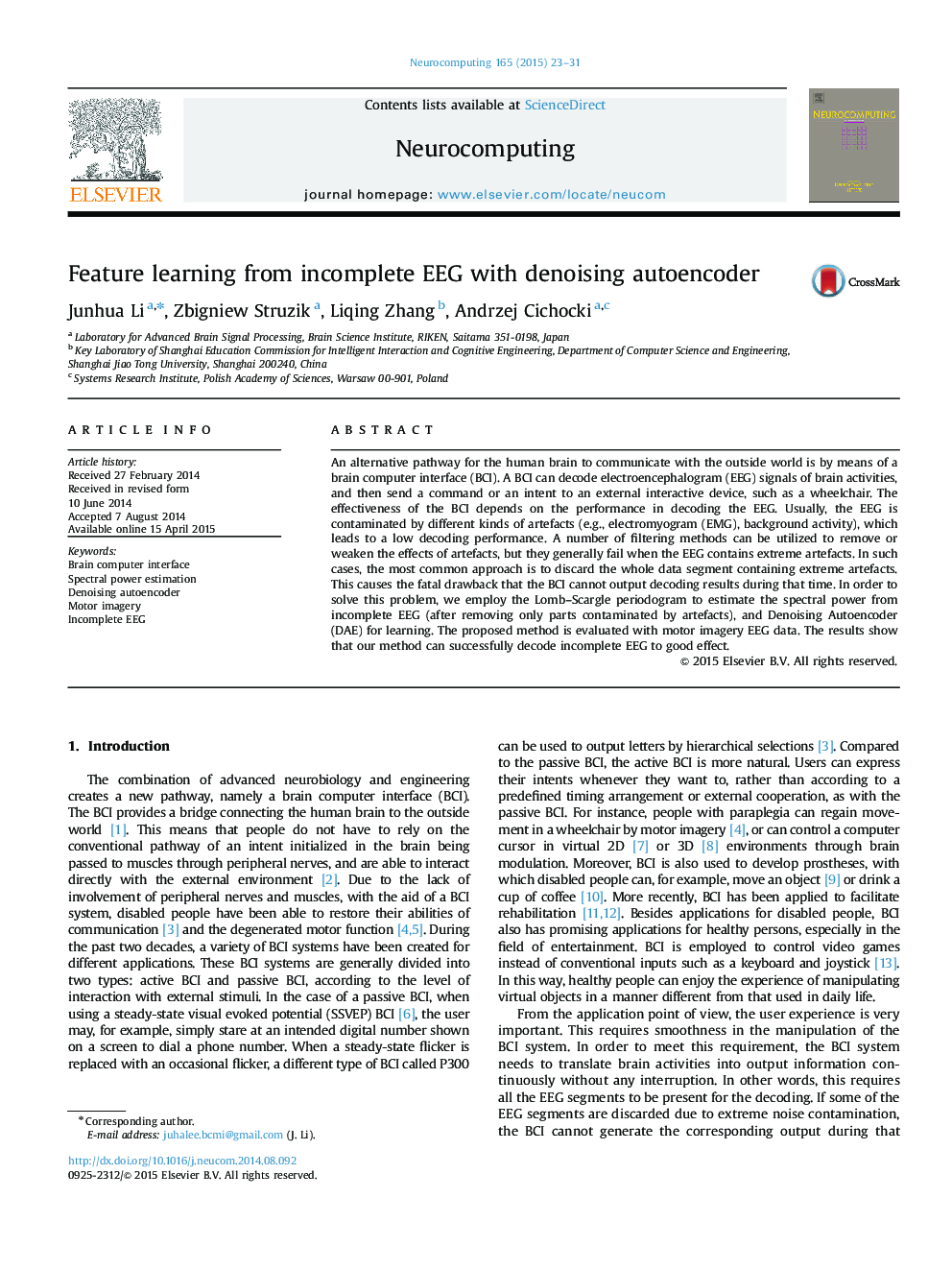
چکیده انگلیسی
An alternative pathway for the human brain to communicate with the outside world is by means of a brain computer interface (BCI). A BCI can decode electroencephalogram (EEG) signals of brain activities, and then send a command or an intent to an external interactive device, such as a wheelchair. The effectiveness of the BCI depends on the performance in decoding the EEG. Usually, the EEG is contaminated by different kinds of artefacts (e.g., electromyogram (EMG), background activity), which leads to a low decoding performance. A number of filtering methods can be utilized to remove or weaken the effects of artefacts, but they generally fail when the EEG contains extreme artefacts. In such cases, the most common approach is to discard the whole data segment containing extreme artefacts. This causes the fatal drawback that the BCI cannot output decoding results during that time. In order to solve this problem, we employ the Lomb-Scargle periodogram to estimate the spectral power from incomplete EEG (after removing only parts contaminated by artefacts), and Denoising Autoencoder (DAE) for learning. The proposed method is evaluated with motor imagery EEG data. The results show that our method can successfully decode incomplete EEG to good effect.
ناشر
Database: Elsevier - ScienceDirect (ساینس دایرکت)
Journal: Neurocomputing - Volume 165, 1 October 2015, Pages 23-31
Journal: Neurocomputing - Volume 165, 1 October 2015, Pages 23-31
نویسندگان
Junhua Li, Zbigniew Struzik, Liqing Zhang, Andrzej Cichocki,