کد مقاله | کد نشریه | سال انتشار | مقاله انگلیسی | نسخه تمام متن |
---|---|---|---|---|
6933221 | 867592 | 2014 | 21 صفحه PDF | دانلود رایگان |
عنوان انگلیسی مقاله ISI
An adaptive ANOVA-based PCKF for high-dimensional nonlinear inverse modeling
دانلود مقاله + سفارش ترجمه
دانلود مقاله ISI انگلیسی
رایگان برای ایرانیان
کلمات کلیدی
موضوعات مرتبط
مهندسی و علوم پایه
مهندسی کامپیوتر
نرم افزارهای علوم کامپیوتر
پیش نمایش صفحه اول مقاله
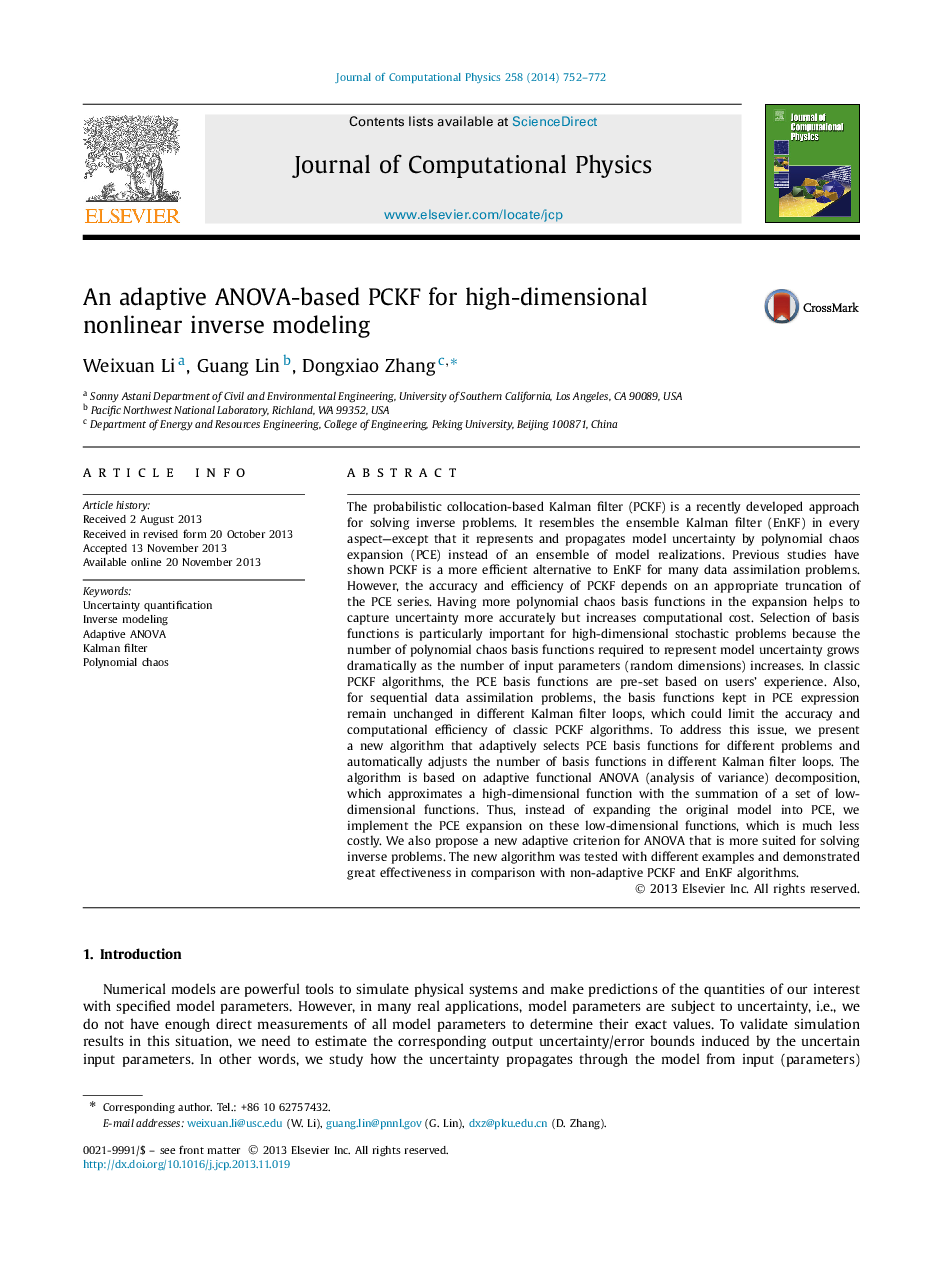
چکیده انگلیسی
The probabilistic collocation-based Kalman filter (PCKF) is a recently developed approach for solving inverse problems. It resembles the ensemble Kalman filter (EnKF) in every aspect-except that it represents and propagates model uncertainty by polynomial chaos expansion (PCE) instead of an ensemble of model realizations. Previous studies have shown PCKF is a more efficient alternative to EnKF for many data assimilation problems. However, the accuracy and efficiency of PCKF depends on an appropriate truncation of the PCE series. Having more polynomial chaos basis functions in the expansion helps to capture uncertainty more accurately but increases computational cost. Selection of basis functions is particularly important for high-dimensional stochastic problems because the number of polynomial chaos basis functions required to represent model uncertainty grows dramatically as the number of input parameters (random dimensions) increases. In classic PCKF algorithms, the PCE basis functions are pre-set based on usersʼ experience. Also, for sequential data assimilation problems, the basis functions kept in PCE expression remain unchanged in different Kalman filter loops, which could limit the accuracy and computational efficiency of classic PCKF algorithms. To address this issue, we present a new algorithm that adaptively selects PCE basis functions for different problems and automatically adjusts the number of basis functions in different Kalman filter loops. The algorithm is based on adaptive functional ANOVA (analysis of variance) decomposition, which approximates a high-dimensional function with the summation of a set of low-dimensional functions. Thus, instead of expanding the original model into PCE, we implement the PCE expansion on these low-dimensional functions, which is much less costly. We also propose a new adaptive criterion for ANOVA that is more suited for solving inverse problems. The new algorithm was tested with different examples and demonstrated great effectiveness in comparison with non-adaptive PCKF and EnKF algorithms.
ناشر
Database: Elsevier - ScienceDirect (ساینس دایرکت)
Journal: Journal of Computational Physics - Volume 258, 1 February 2014, Pages 752-772
Journal: Journal of Computational Physics - Volume 258, 1 February 2014, Pages 752-772
نویسندگان
Weixuan Li, Guang Lin, Dongxiao Zhang,