کد مقاله | کد نشریه | سال انتشار | مقاله انگلیسی | نسخه تمام متن |
---|---|---|---|---|
6935847 | 1449656 | 2018 | 26 صفحه PDF | دانلود رایگان |
عنوان انگلیسی مقاله ISI
Quantifying uncertainty in short-term traffic prediction and its application to optimal staffing plan development
دانلود مقاله + سفارش ترجمه
دانلود مقاله ISI انگلیسی
رایگان برای ایرانیان
کلمات کلیدی
موضوعات مرتبط
مهندسی و علوم پایه
مهندسی کامپیوتر
نرم افزارهای علوم کامپیوتر
پیش نمایش صفحه اول مقاله
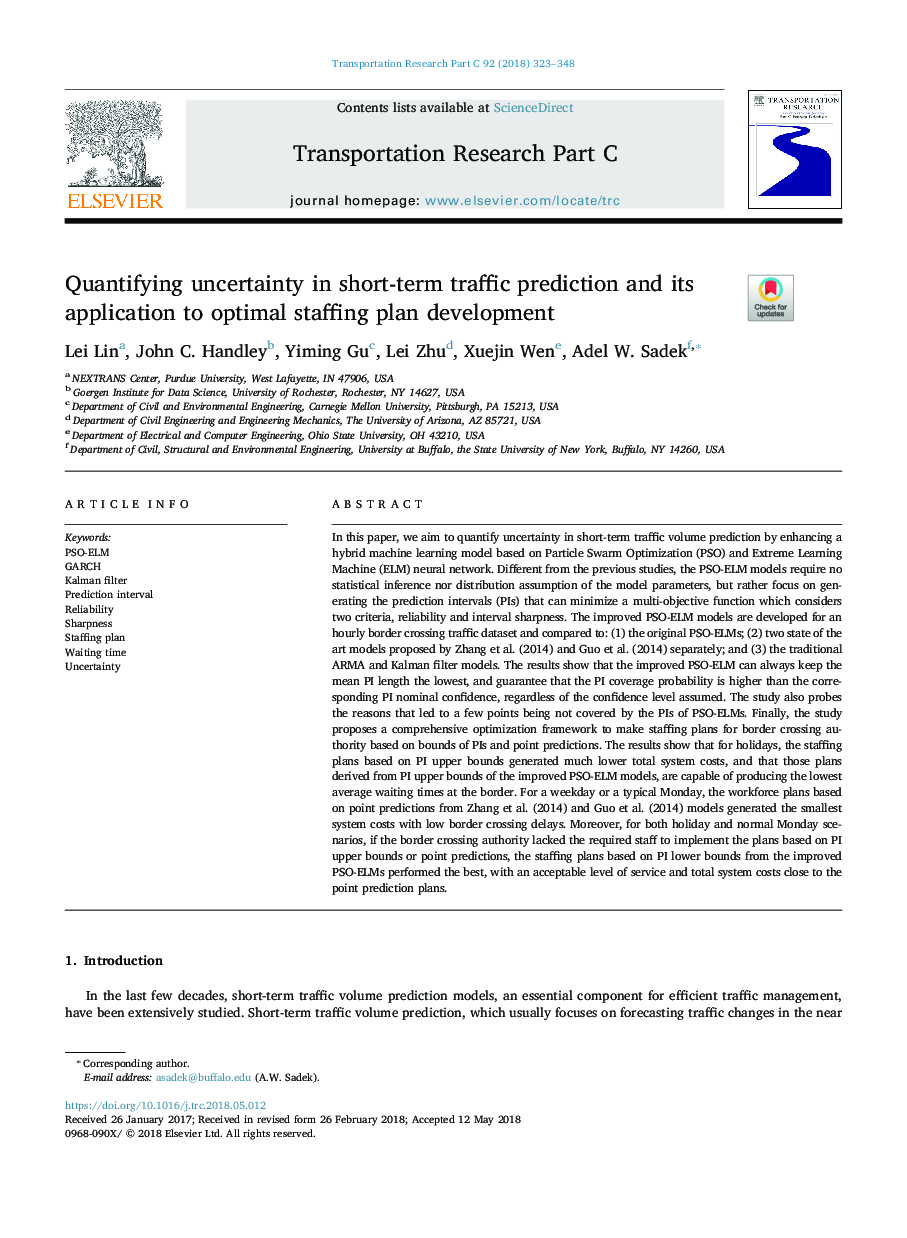
چکیده انگلیسی
In this paper, we aim to quantify uncertainty in short-term traffic volume prediction by enhancing a hybrid machine learning model based on Particle Swarm Optimization (PSO) and Extreme Learning Machine (ELM) neural network. Different from the previous studies, the PSO-ELM models require no statistical inference nor distribution assumption of the model parameters, but rather focus on generating the prediction intervals (PIs) that can minimize a multi-objective function which considers two criteria, reliability and interval sharpness. The improved PSO-ELM models are developed for an hourly border crossing traffic dataset and compared to: (1) the original PSO-ELMs; (2) two state of the art models proposed by Zhang et al. (2014) and Guo et al. (2014) separately; and (3) the traditional ARMA and Kalman filter models. The results show that the improved PSO-ELM can always keep the mean PI length the lowest, and guarantee that the PI coverage probability is higher than the corresponding PI nominal confidence, regardless of the confidence level assumed. The study also probes the reasons that led to a few points being not covered by the PIs of PSO-ELMs. Finally, the study proposes a comprehensive optimization framework to make staffing plans for border crossing authority based on bounds of PIs and point predictions. The results show that for holidays, the staffing plans based on PI upper bounds generated much lower total system costs, and that those plans derived from PI upper bounds of the improved PSO-ELM models, are capable of producing the lowest average waiting times at the border. For a weekday or a typical Monday, the workforce plans based on point predictions from Zhang et al. (2014) and Guo et al. (2014) models generated the smallest system costs with low border crossing delays. Moreover, for both holiday and normal Monday scenarios, if the border crossing authority lacked the required staff to implement the plans based on PI upper bounds or point predictions, the staffing plans based on PI lower bounds from the improved PSO-ELMs performed the best, with an acceptable level of service and total system costs close to the point prediction plans.
ناشر
Database: Elsevier - ScienceDirect (ساینس دایرکت)
Journal: Transportation Research Part C: Emerging Technologies - Volume 92, July 2018, Pages 323-348
Journal: Transportation Research Part C: Emerging Technologies - Volume 92, July 2018, Pages 323-348
نویسندگان
Lei Lin, John C. Handley, Yiming Gu, Lei Zhu, Xuejin Wen, Adel W. Sadek,