کد مقاله | کد نشریه | سال انتشار | مقاله انگلیسی | نسخه تمام متن |
---|---|---|---|---|
6938661 | 1449963 | 2018 | 11 صفحه PDF | دانلود رایگان |
عنوان انگلیسی مقاله ISI
MoE-SPNet: A mixture-of-experts scene parsing network
دانلود مقاله + سفارش ترجمه
دانلود مقاله ISI انگلیسی
رایگان برای ایرانیان
کلمات کلیدی
موضوعات مرتبط
مهندسی و علوم پایه
مهندسی کامپیوتر
چشم انداز کامپیوتر و تشخیص الگو
پیش نمایش صفحه اول مقاله
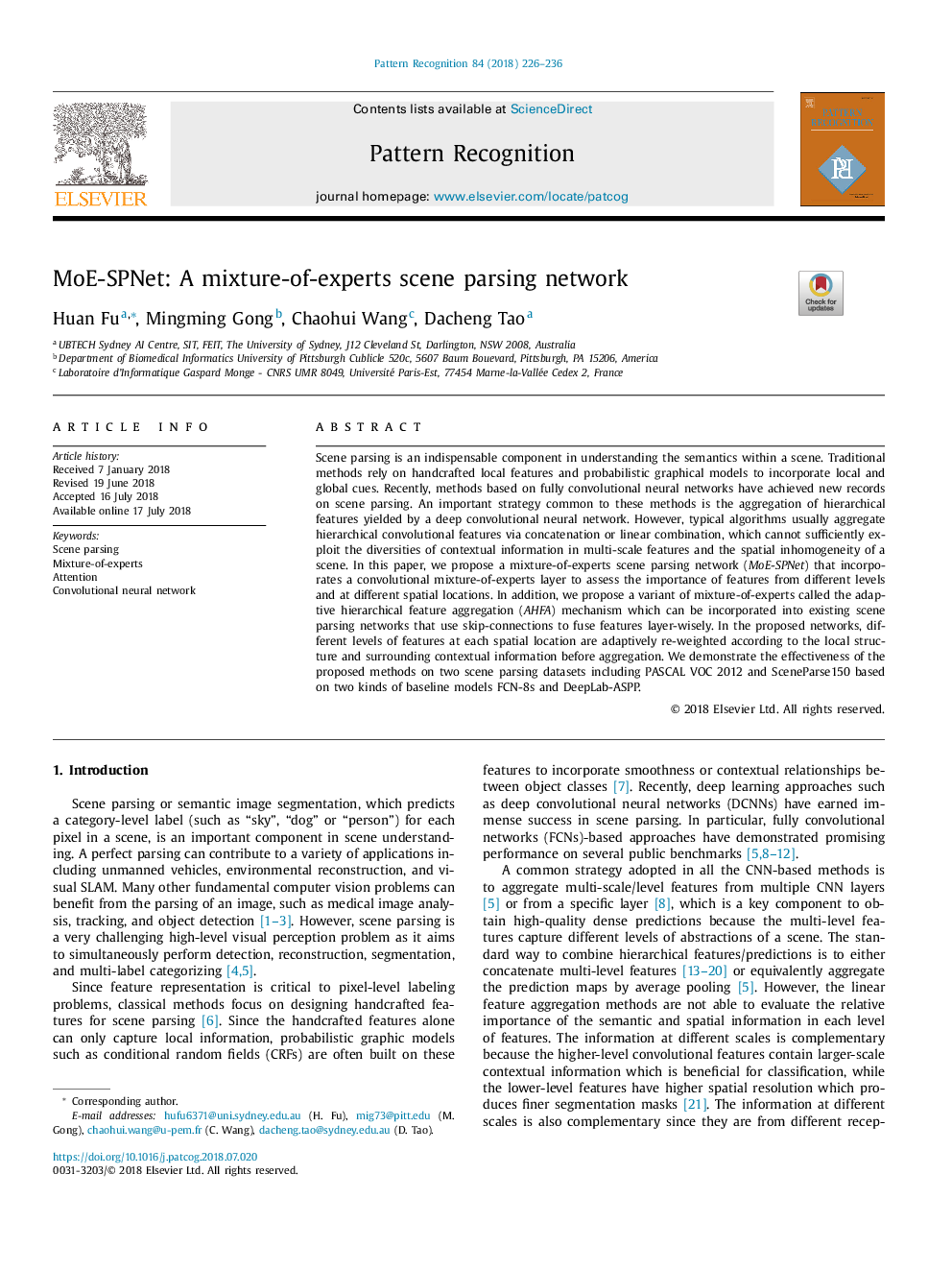
چکیده انگلیسی
Scene parsing is an indispensable component in understanding the semantics within a scene. Traditional methods rely on handcrafted local features and probabilistic graphical models to incorporate local and global cues. Recently, methods based on fully convolutional neural networks have achieved new records on scene parsing. An important strategy common to these methods is the aggregation of hierarchical features yielded by a deep convolutional neural network. However, typical algorithms usually aggregate hierarchical convolutional features via concatenation or linear combination, which cannot sufficiently exploit the diversities of contextual information in multi-scale features and the spatial inhomogeneity of a scene. In this paper, we propose a mixture-of-experts scene parsing network (MoE-SPNet) that incorporates a convolutional mixture-of-experts layer to assess the importance of features from different levels and at different spatial locations. In addition, we propose a variant of mixture-of-experts called the adaptive hierarchical feature aggregation (AHFA) mechanism which can be incorporated into existing scene parsing networks that use skip-connections to fuse features layer-wisely. In the proposed networks, different levels of features at each spatial location are adaptively re-weighted according to the local structure and surrounding contextual information before aggregation. We demonstrate the effectiveness of the proposed methods on two scene parsing datasets including PASCAL VOC 2012 and SceneParse150 based on two kinds of baseline models FCN-8s and DeepLab-ASPP.
ناشر
Database: Elsevier - ScienceDirect (ساینس دایرکت)
Journal: Pattern Recognition - Volume 84, December 2018, Pages 226-236
Journal: Pattern Recognition - Volume 84, December 2018, Pages 226-236
نویسندگان
Huan Fu, Mingming Gong, Chaohui Wang, Dacheng Tao,