کد مقاله | کد نشریه | سال انتشار | مقاله انگلیسی | نسخه تمام متن |
---|---|---|---|---|
6940676 | 1450017 | 2018 | 7 صفحه PDF | دانلود رایگان |
عنوان انگلیسی مقاله ISI
Improved hypergraph regularized Nonnegative Matrix Factorization with sparse representation
دانلود مقاله + سفارش ترجمه
دانلود مقاله ISI انگلیسی
رایگان برای ایرانیان
کلمات کلیدی
موضوعات مرتبط
مهندسی و علوم پایه
مهندسی کامپیوتر
چشم انداز کامپیوتر و تشخیص الگو
پیش نمایش صفحه اول مقاله
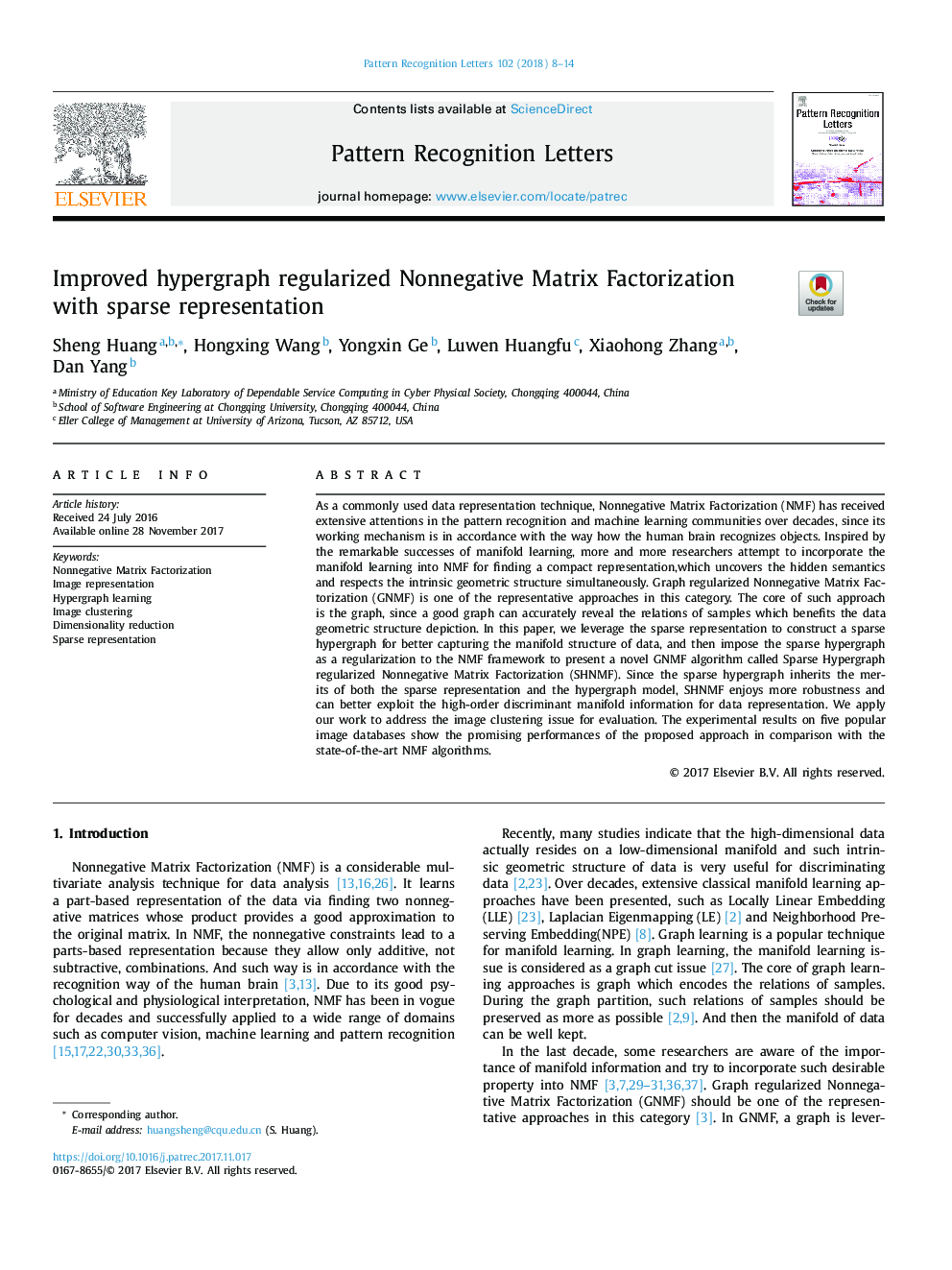
چکیده انگلیسی
As a commonly used data representation technique, Nonnegative Matrix Factorization (NMF) has received extensive attentions in the pattern recognition and machine learning communities over decades, since its working mechanism is in accordance with the way how the human brain recognizes objects. Inspired by the remarkable successes of manifold learning, more and more researchers attempt to incorporate the manifold learning into NMF for finding a compact representation,which uncovers the hidden semantics and respects the intrinsic geometric structure simultaneously. Graph regularized Nonnegative Matrix Factorization (GNMF) is one of the representative approaches in this category. The core of such approach is the graph, since a good graph can accurately reveal the relations of samples which benefits the data geometric structure depiction. In this paper, we leverage the sparse representation to construct a sparse hypergraph for better capturing the manifold structure of data, and then impose the sparse hypergraph as a regularization to the NMF framework to present a novel GNMF algorithm called Sparse Hypergraph regularized Nonnegative Matrix Factorization (SHNMF). Since the sparse hypergraph inherits the merits of both the sparse representation and the hypergraph model, SHNMF enjoys more robustness and can better exploit the high-order discriminant manifold information for data representation. We apply our work to address the image clustering issue for evaluation. The experimental results on five popular image databases show the promising performances of the proposed approach in comparison with the state-of-the-art NMF algorithms.
ناشر
Database: Elsevier - ScienceDirect (ساینس دایرکت)
Journal: Pattern Recognition Letters - Volume 102, 15 January 2018, Pages 8-14
Journal: Pattern Recognition Letters - Volume 102, 15 January 2018, Pages 8-14
نویسندگان
Sheng Huang, Hongxing Wang, Yongxin Ge, Luwen Huangfu, Xiaohong Zhang, Dan Yang,