کد مقاله | کد نشریه | سال انتشار | مقاله انگلیسی | نسخه تمام متن |
---|---|---|---|---|
6941177 | 870156 | 2015 | 7 صفحه PDF | دانلود رایگان |
عنوان انگلیسی مقاله ISI
Measure for data partitioning in m à 2 cross-validation
دانلود مقاله + سفارش ترجمه
دانلود مقاله ISI انگلیسی
رایگان برای ایرانیان
کلمات کلیدی
موضوعات مرتبط
مهندسی و علوم پایه
مهندسی کامپیوتر
چشم انداز کامپیوتر و تشخیص الگو
پیش نمایش صفحه اول مقاله
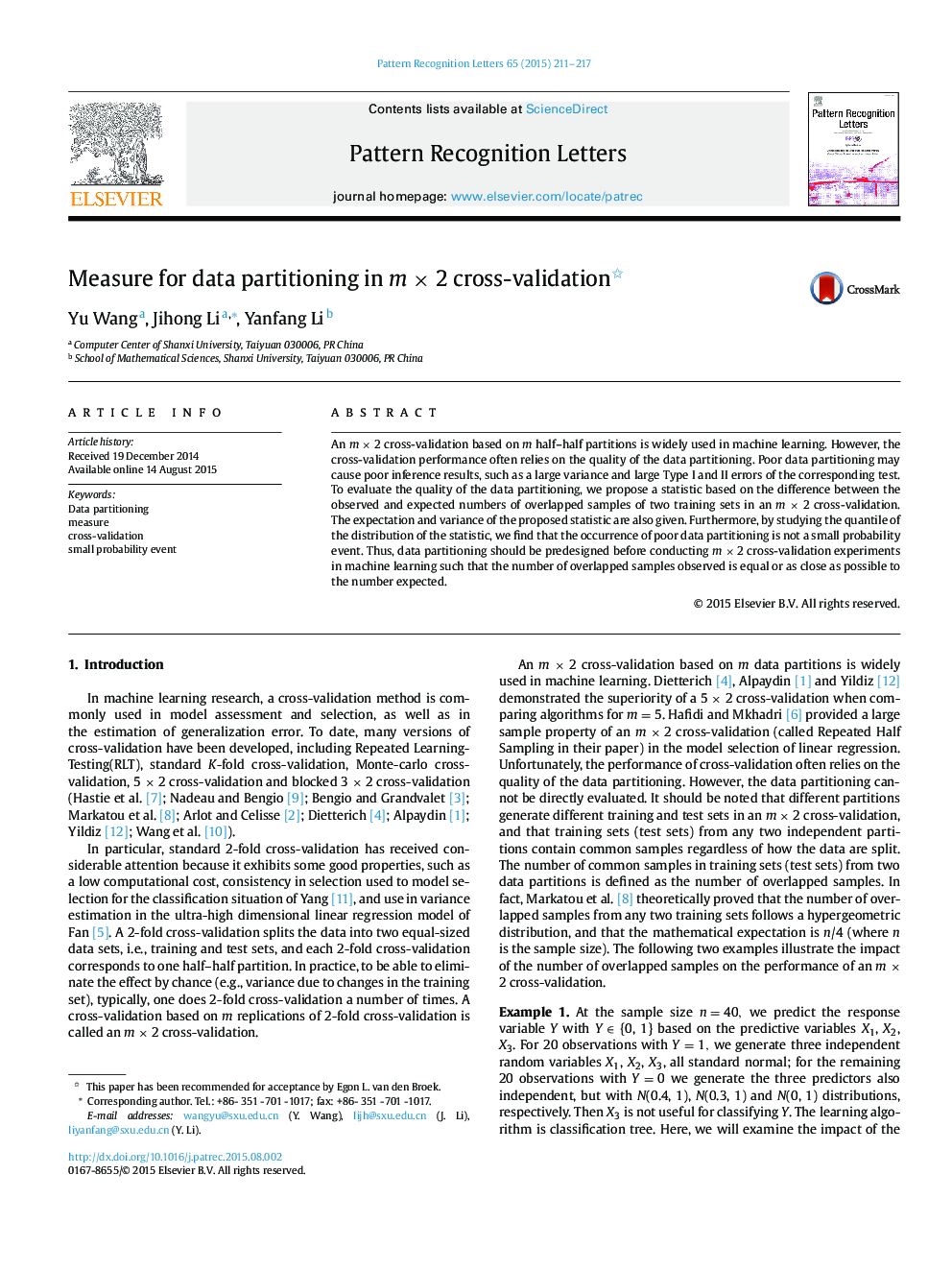
چکیده انگلیسی
An m à 2 cross-validation based on m half-half partitions is widely used in machine learning. However, the cross-validation performance often relies on the quality of the data partitioning. Poor data partitioning may cause poor inference results, such as a large variance and large Type I and II errors of the corresponding test. To evaluate the quality of the data partitioning, we propose a statistic based on the difference between the observed and expected numbers of overlapped samples of two training sets in an m à 2 cross-validation. The expectation and variance of the proposed statistic are also given. Furthermore, by studying the quantile of the distribution of the statistic, we find that the occurrence of poor data partitioning is not a small probability event. Thus, data partitioning should be predesigned before conducting m à 2 cross-validation experiments in machine learning such that the number of overlapped samples observed is equal or as close as possible to the number expected.
ناشر
Database: Elsevier - ScienceDirect (ساینس دایرکت)
Journal: Pattern Recognition Letters - Volume 65, 1 November 2015, Pages 211-217
Journal: Pattern Recognition Letters - Volume 65, 1 November 2015, Pages 211-217
نویسندگان
Yu Wang, Jihong Li, Yanfang Li,