کد مقاله | کد نشریه | سال انتشار | مقاله انگلیسی | نسخه تمام متن |
---|---|---|---|---|
6949216 | 1451236 | 2018 | 16 صفحه PDF | دانلود رایگان |
عنوان انگلیسی مقاله ISI
Spectral-spatial classification of hyperspectral data with mutual information based segmented stacked autoencoder approach
دانلود مقاله + سفارش ترجمه
دانلود مقاله ISI انگلیسی
رایگان برای ایرانیان
کلمات کلیدی
موضوعات مرتبط
مهندسی و علوم پایه
مهندسی کامپیوتر
سیستم های اطلاعاتی
پیش نمایش صفحه اول مقاله
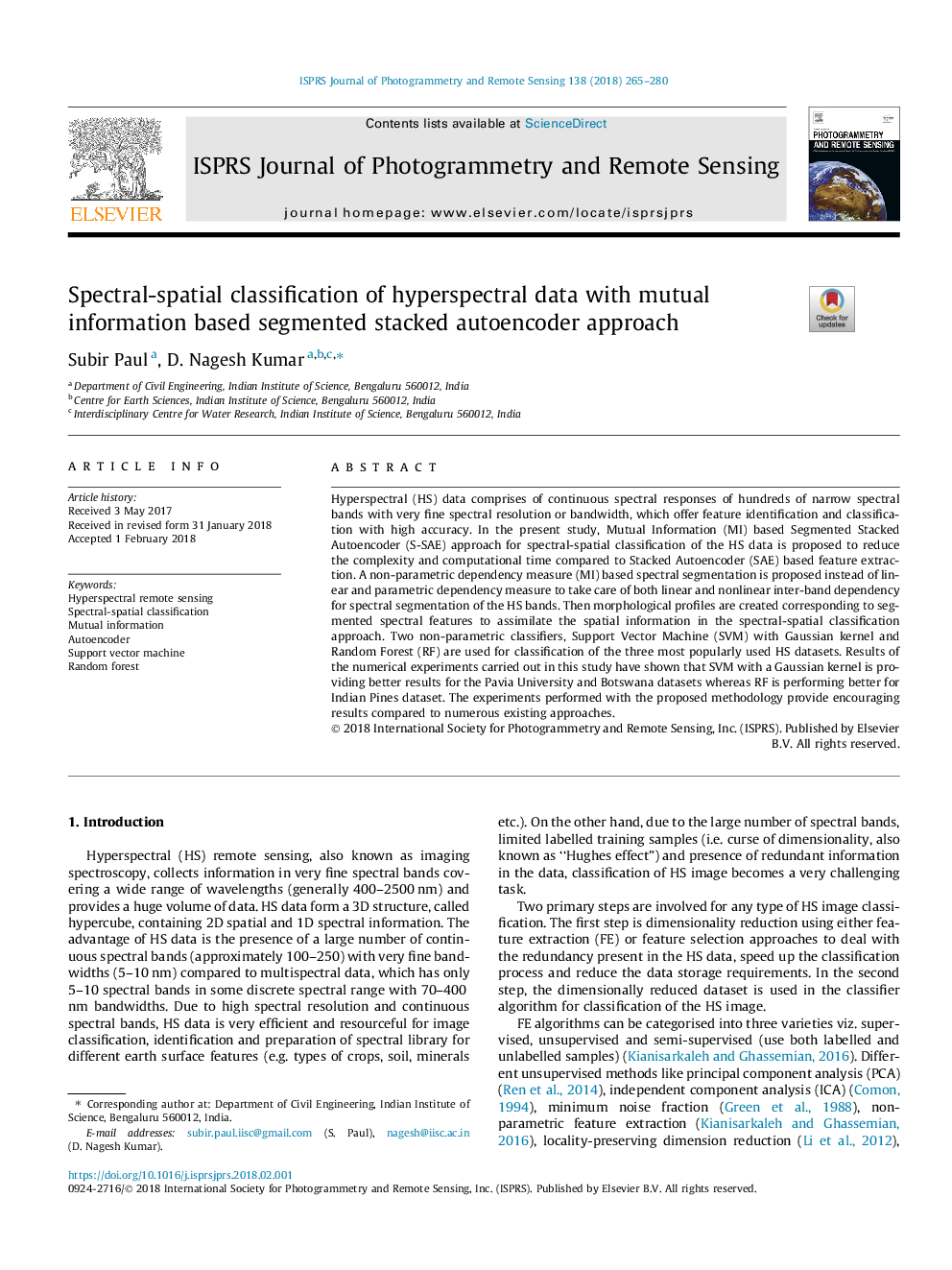
چکیده انگلیسی
Hyperspectral (HS) data comprises of continuous spectral responses of hundreds of narrow spectral bands with very fine spectral resolution or bandwidth, which offer feature identification and classification with high accuracy. In the present study, Mutual Information (MI) based Segmented Stacked Autoencoder (S-SAE) approach for spectral-spatial classification of the HS data is proposed to reduce the complexity and computational time compared to Stacked Autoencoder (SAE) based feature extraction. A non-parametric dependency measure (MI) based spectral segmentation is proposed instead of linear and parametric dependency measure to take care of both linear and nonlinear inter-band dependency for spectral segmentation of the HS bands. Then morphological profiles are created corresponding to segmented spectral features to assimilate the spatial information in the spectral-spatial classification approach. Two non-parametric classifiers, Support Vector Machine (SVM) with Gaussian kernel and Random Forest (RF) are used for classification of the three most popularly used HS datasets. Results of the numerical experiments carried out in this study have shown that SVM with a Gaussian kernel is providing better results for the Pavia University and Botswana datasets whereas RF is performing better for Indian Pines dataset. The experiments performed with the proposed methodology provide encouraging results compared to numerous existing approaches.
ناشر
Database: Elsevier - ScienceDirect (ساینس دایرکت)
Journal: ISPRS Journal of Photogrammetry and Remote Sensing - Volume 138, April 2018, Pages 265-280
Journal: ISPRS Journal of Photogrammetry and Remote Sensing - Volume 138, April 2018, Pages 265-280
نویسندگان
Subir Paul, D. Nagesh Kumar,