کد مقاله | کد نشریه | سال انتشار | مقاله انگلیسی | نسخه تمام متن |
---|---|---|---|---|
730460 | 892974 | 2011 | 8 صفحه PDF | دانلود رایگان |
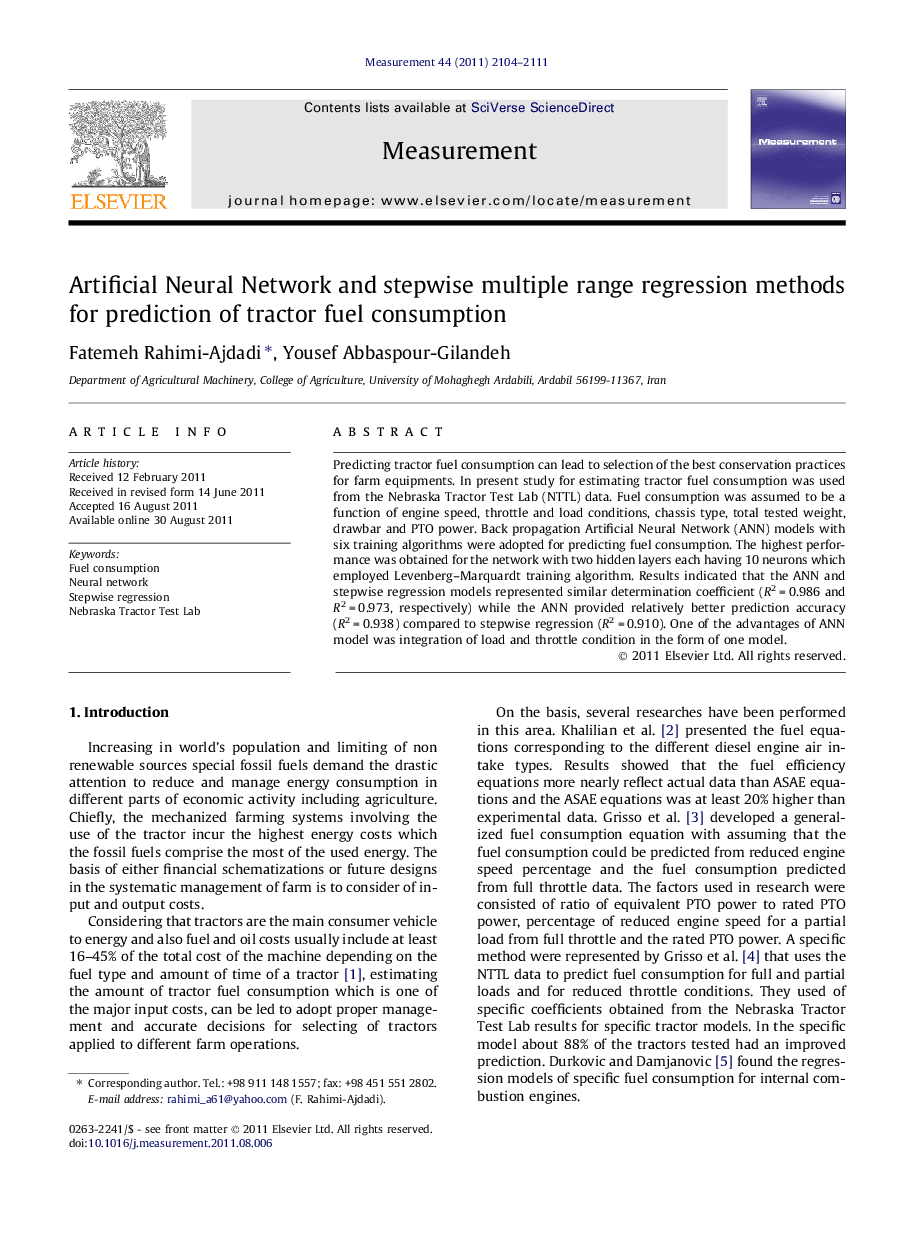
Predicting tractor fuel consumption can lead to selection of the best conservation practices for farm equipments. In present study for estimating tractor fuel consumption was used from the Nebraska Tractor Test Lab (NTTL) data. Fuel consumption was assumed to be a function of engine speed, throttle and load conditions, chassis type, total tested weight, drawbar and PTO power. Back propagation Artificial Neural Network (ANN) models with six training algorithms were adopted for predicting fuel consumption. The highest performance was obtained for the network with two hidden layers each having 10 neurons which employed Levenberg–Marquardt training algorithm. Results indicated that the ANN and stepwise regression models represented similar determination coefficient (R2 = 0.986 and R2 = 0.973, respectively) while the ANN provided relatively better prediction accuracy (R2 = 0.938) compared to stepwise regression (R2 = 0.910). One of the advantages of ANN model was integration of load and throttle condition in the form of one model.
► ANN and multiple regression models can be suggested to predict fuel consumption of tractor engines.
► The best structure of ANN was two hidden layers with 10 neurons and Levenberg–Marquardt algorithm.
► ANN provided better prediction accuracy (R2 = 0.938) compared to stepwise regression (R2 = 0.910).
► One of the advantages of ANN model was integration of load and throttle condition in the form of one model.
Journal: Measurement - Volume 44, Issue 10, December 2011, Pages 2104–2111