کد مقاله | کد نشریه | سال انتشار | مقاله انگلیسی | نسخه تمام متن |
---|---|---|---|---|
741443 | 894242 | 2008 | 7 صفحه PDF | دانلود رایگان |
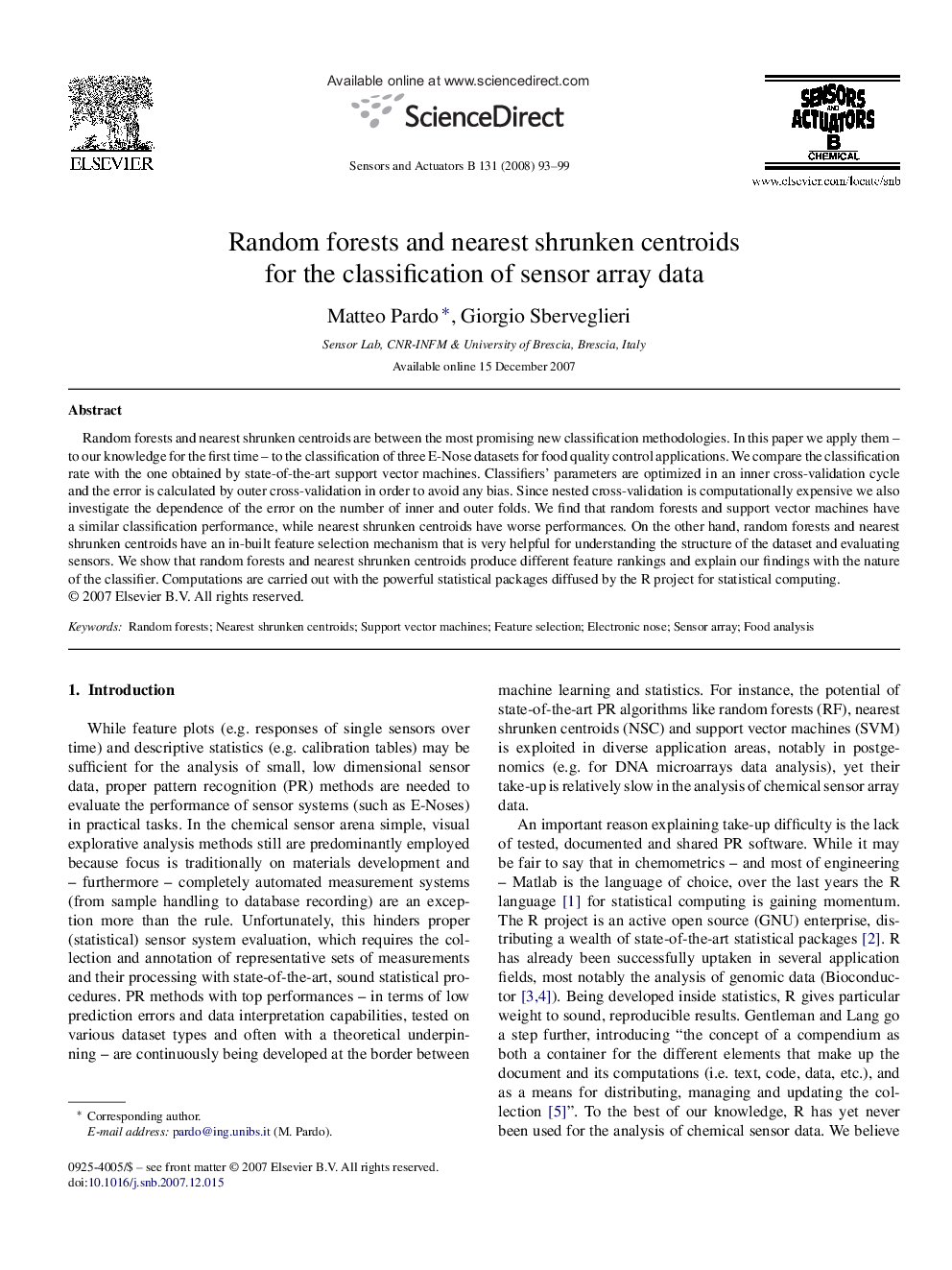
Random forests and nearest shrunken centroids are between the most promising new classification methodologies. In this paper we apply them – to our knowledge for the first time – to the classification of three E-Nose datasets for food quality control applications. We compare the classification rate with the one obtained by state-of-the-art support vector machines. Classifiers’ parameters are optimized in an inner cross-validation cycle and the error is calculated by outer cross-validation in order to avoid any bias. Since nested cross-validation is computationally expensive we also investigate the dependence of the error on the number of inner and outer folds. We find that random forests and support vector machines have a similar classification performance, while nearest shrunken centroids have worse performances. On the other hand, random forests and nearest shrunken centroids have an in-built feature selection mechanism that is very helpful for understanding the structure of the dataset and evaluating sensors. We show that random forests and nearest shrunken centroids produce different feature rankings and explain our findings with the nature of the classifier. Computations are carried out with the powerful statistical packages diffused by the R project for statistical computing.
Journal: Sensors and Actuators B: Chemical - Volume 131, Issue 1, 14 April 2008, Pages 93–99