کد مقاله | کد نشریه | سال انتشار | مقاله انگلیسی | نسخه تمام متن |
---|---|---|---|---|
7541074 | 1489047 | 2018 | 15 صفحه PDF | دانلود رایگان |
عنوان انگلیسی مقاله ISI
Optimal Bayesian control policy for gear shaft fault detection using hidden semi-Markov model
دانلود مقاله + سفارش ترجمه
دانلود مقاله ISI انگلیسی
رایگان برای ایرانیان
کلمات کلیدی
موضوعات مرتبط
مهندسی و علوم پایه
سایر رشته های مهندسی
مهندسی صنعتی و تولید
پیش نمایش صفحه اول مقاله
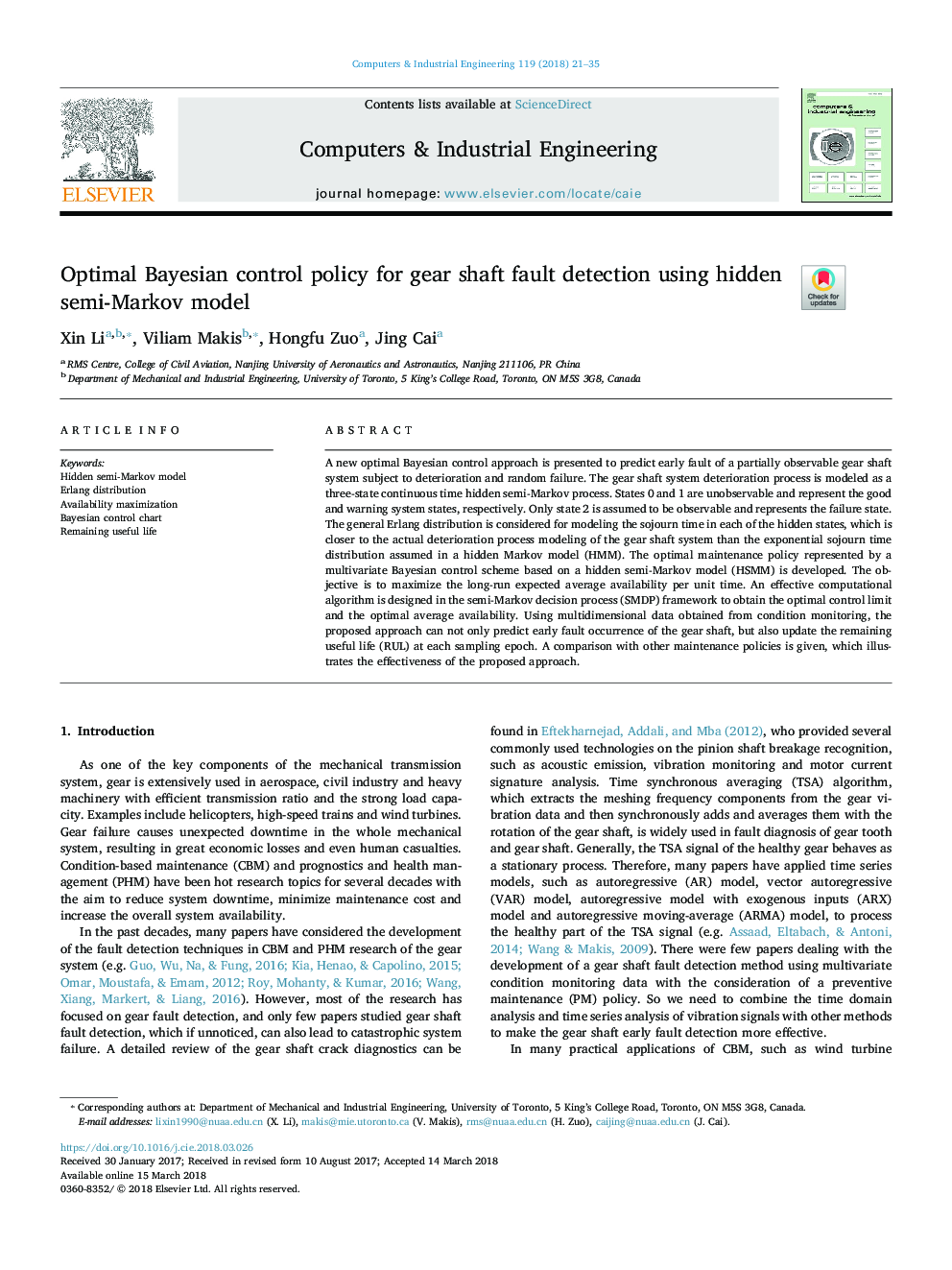
چکیده انگلیسی
A new optimal Bayesian control approach is presented to predict early fault of a partially observable gear shaft system subject to deterioration and random failure. The gear shaft system deterioration process is modeled as a three-state continuous time hidden semi-Markov process. States 0 and 1 are unobservable and represent the good and warning system states, respectively. Only state 2 is assumed to be observable and represents the failure state. The general Erlang distribution is considered for modeling the sojourn time in each of the hidden states, which is closer to the actual deterioration process modeling of the gear shaft system than the exponential sojourn time distribution assumed in a hidden Markov model (HMM). The optimal maintenance policy represented by a multivariate Bayesian control scheme based on a hidden semi-Markov model (HSMM) is developed. The objective is to maximize the long-run expected average availability per unit time. An effective computational algorithm is designed in the semi-Markov decision process (SMDP) framework to obtain the optimal control limit and the optimal average availability. Using multidimensional data obtained from condition monitoring, the proposed approach can not only predict early fault occurrence of the gear shaft, but also update the remaining useful life (RUL) at each sampling epoch. A comparison with other maintenance policies is given, which illustrates the effectiveness of the proposed approach.
ناشر
Database: Elsevier - ScienceDirect (ساینس دایرکت)
Journal: Computers & Industrial Engineering - Volume 119, May 2018, Pages 21-35
Journal: Computers & Industrial Engineering - Volume 119, May 2018, Pages 21-35
نویسندگان
Xin Li, Viliam Makis, Hongfu Zuo, Jing Cai,