کد مقاله | کد نشریه | سال انتشار | مقاله انگلیسی | نسخه تمام متن |
---|---|---|---|---|
7541223 | 1489048 | 2018 | 20 صفحه PDF | دانلود رایگان |
عنوان انگلیسی مقاله ISI
A least squares-based parallel hybridization of statistical and intelligent models for time series forecasting
دانلود مقاله + سفارش ترجمه
دانلود مقاله ISI انگلیسی
رایگان برای ایرانیان
کلمات کلیدی
موضوعات مرتبط
مهندسی و علوم پایه
سایر رشته های مهندسی
مهندسی صنعتی و تولید
پیش نمایش صفحه اول مقاله
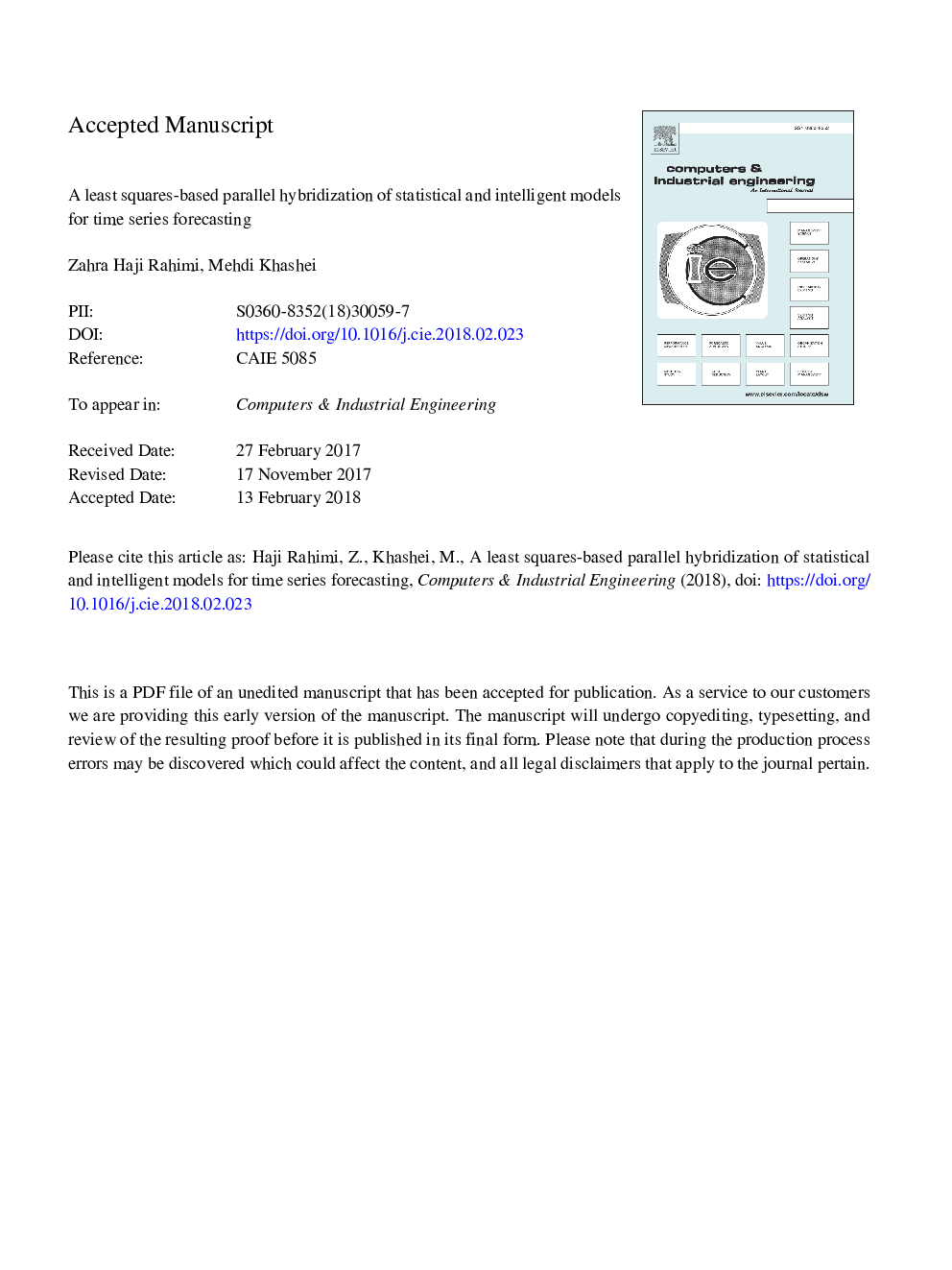
چکیده انگلیسی
Combining different models is one of the most well established strategies in the literature in order to capture different patterns of the data, lifting limitations of single models, and improve forecasting accuracy. In recent decades, various hybrid models have been developed by combining different models such as linear/nonlinear, supervised/unsupervised fuzzy/crisp, and statistical/intelligent together. Intelligent and statistical techniques due to their unique advantages are one of the most popular and the most widely-used candidates to build hybrid models for time series forecasting. These models are generally combined together in two different schemas: series and parallel. In series schema, components are sequentially used for modeling the underlying data; while in parallel schema, components are separately used and then their results are combined together. In this paper, a new parallel hybrid model is proposed, incorporating autoregressive integrated moving average (ARIMA) and multilayer perceptrons (MLPs), which are one of the most important intelligent and statistical time series models. In addition, a least squares process is used in order to find the optimum weights of each component. The main idea of the proposed model is to employ special features of ARIMA and MLP models in modeling linear and nonlinear patterns in the data simultaneously and to yield the best performance by reasonable computational costs. To evaluate the effectiveness of the least squares based proposed hybrid model (LPHM) in financial forecasting, three well-known benchmark data sets including the closing of Standard & Poor's 500 index (S&P500), the closing of the Shenzhen Integrated Index (SZII), and the opening of Dow Jones Industrial Average Index (DJIAI) are elected. Empirical results indicate that LPHM outperforms its constituent as well as genetic algorithm based hybrid model (GAHM) and simple average hybrid model (SAHM) in stock price forecasting. While, the computational cost of the proposed model is significantly lower than the GAHM and roughly equal to the SAHM.
ناشر
Database: Elsevier - ScienceDirect (ساینس دایرکت)
Journal: Computers & Industrial Engineering - Volume 118, April 2018, Pages 44-53
Journal: Computers & Industrial Engineering - Volume 118, April 2018, Pages 44-53
نویسندگان
Zahra Haji Rahimi, Mehdi Khashei,