کد مقاله | کد نشریه | سال انتشار | مقاله انگلیسی | نسخه تمام متن |
---|---|---|---|---|
7546412 | 1489632 | 2018 | 14 صفحه PDF | دانلود رایگان |
عنوان انگلیسی مقاله ISI
An RKHS-based approach to double-penalized regression in high-dimensional partially linear models
دانلود مقاله + سفارش ترجمه
دانلود مقاله ISI انگلیسی
رایگان برای ایرانیان
کلمات کلیدی
موضوعات مرتبط
مهندسی و علوم پایه
ریاضیات
آنالیز عددی
پیش نمایش صفحه اول مقاله
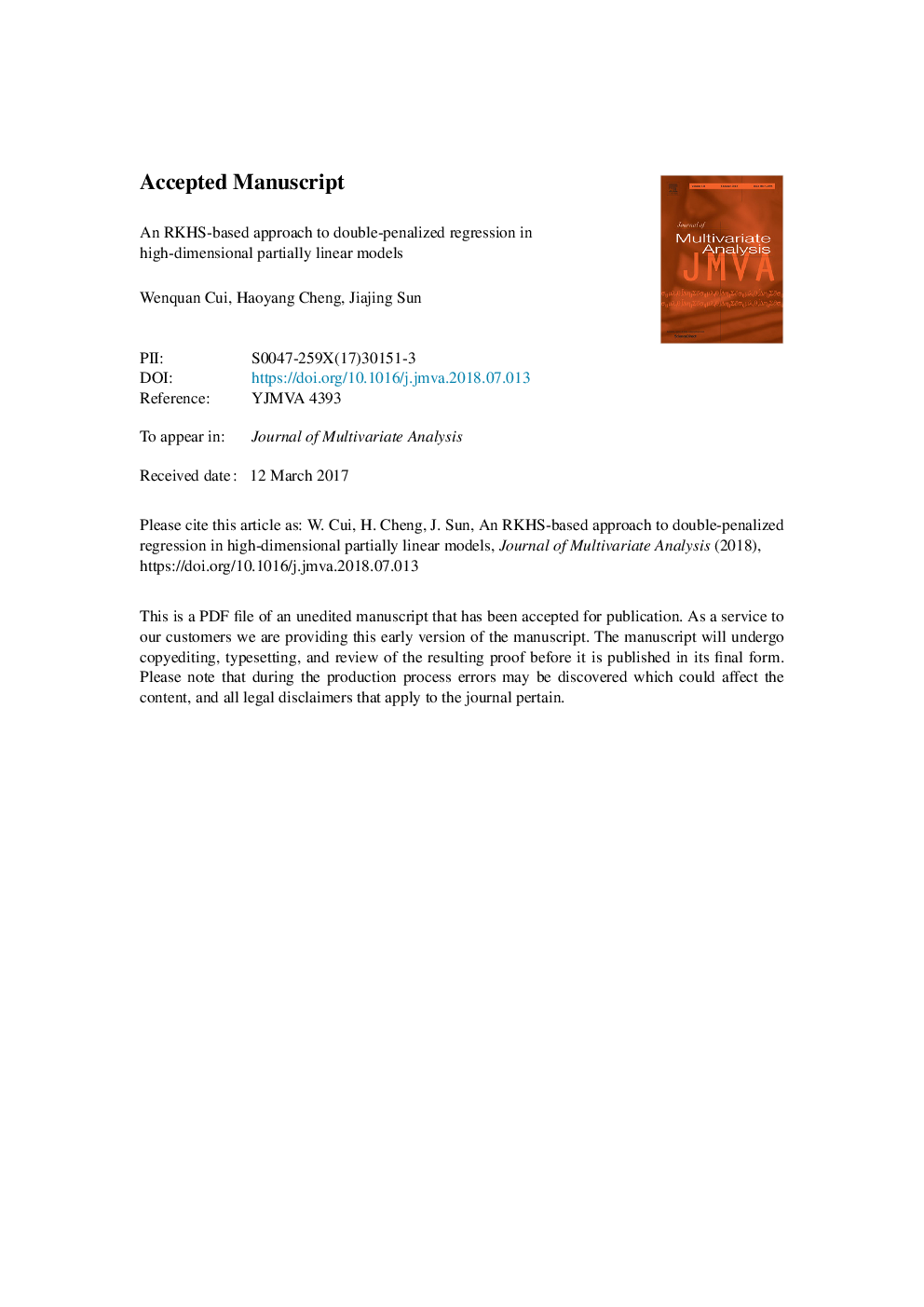
چکیده انگلیسی
We study simultaneous variable selection and estimation in high-dimensional partially linear models under the assumption that the nonparametric component is from a reproducing kernel Hilbert space (RKHS) and that the vector of regression coefficients for the parametric component is sparse. A double penalty is used to deal with the problem. The estimate of the nonparametric component is subject to a roughness penalty based on the squared semi-norm on the RKHS, and a penalty with oracle properties is used to achieve sparsity in the parametric component. Under regularity conditions, we establish the consistency and rate of convergence of the parametric estimation together with the consistency of variable selection. The proposed estimators of the non-zero coefficients are also shown to have the asymptotic oracle property. Simulations and empirical studies illustrate the performance of the method.
ناشر
Database: Elsevier - ScienceDirect (ساینس دایرکت)
Journal: Journal of Multivariate Analysis - Volume 168, November 2018, Pages 201-210
Journal: Journal of Multivariate Analysis - Volume 168, November 2018, Pages 201-210
نویسندگان
Wenquan Cui, Haoyang Cheng, Jiajing Sun,