کد مقاله | کد نشریه | سال انتشار | مقاله انگلیسی | نسخه تمام متن |
---|---|---|---|---|
807218 | 905483 | 2010 | 9 صفحه PDF | دانلود رایگان |
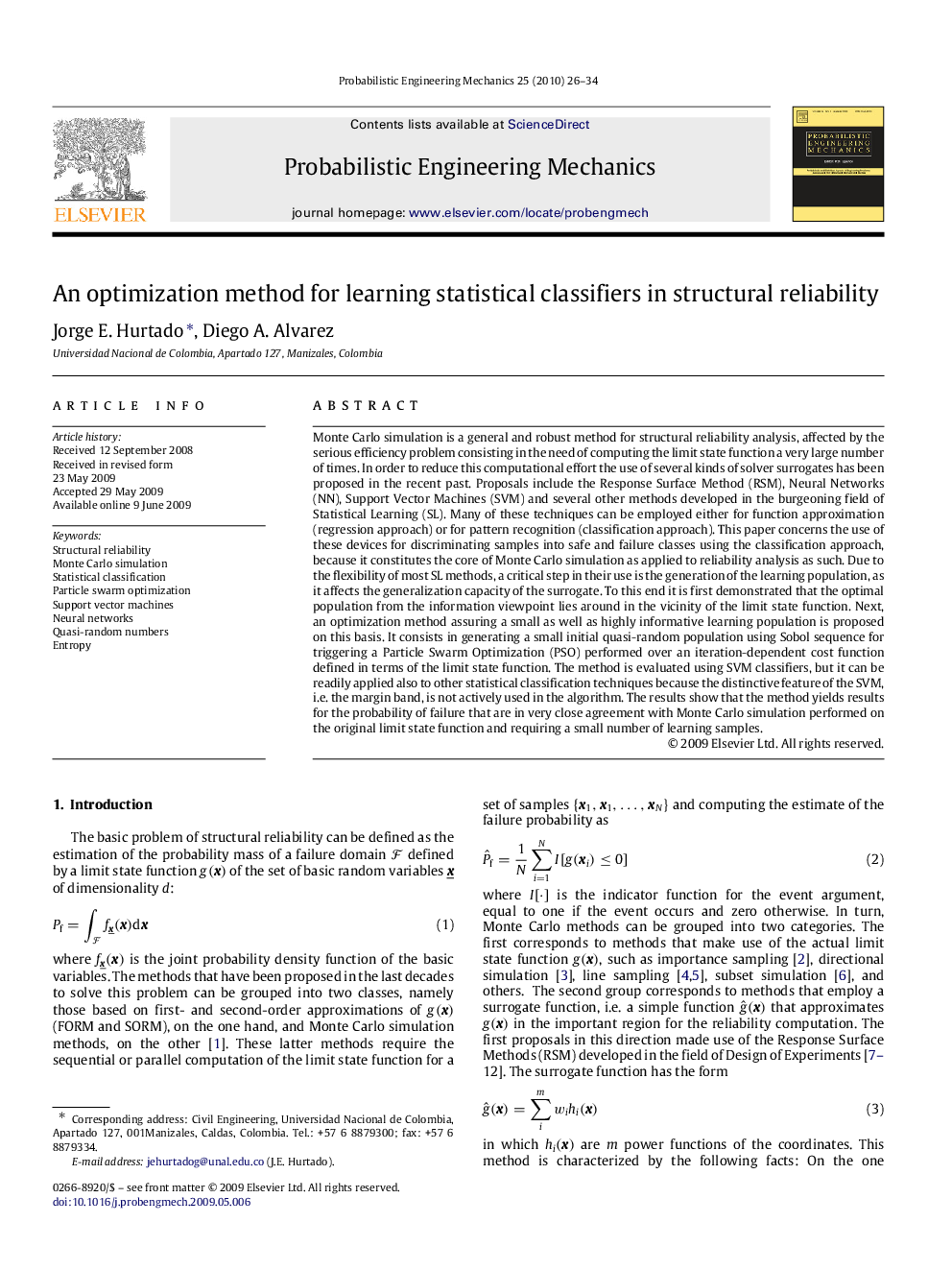
Monte Carlo simulation is a general and robust method for structural reliability analysis, affected by the serious efficiency problem consisting in the need of computing the limit state function a very large number of times. In order to reduce this computational effort the use of several kinds of solver surrogates has been proposed in the recent past. Proposals include the Response Surface Method (RSM), Neural Networks (NN), Support Vector Machines (SVM) and several other methods developed in the burgeoning field of Statistical Learning (SL). Many of these techniques can be employed either for function approximation (regression approach) or for pattern recognition (classification approach). This paper concerns the use of these devices for discriminating samples into safe and failure classes using the classification approach, because it constitutes the core of Monte Carlo simulation as applied to reliability analysis as such. Due to the flexibility of most SL methods, a critical step in their use is the generation of the learning population, as it affects the generalization capacity of the surrogate. To this end it is first demonstrated that the optimal population from the information viewpoint lies around in the vicinity of the limit state function. Next, an optimization method assuring a small as well as highly informative learning population is proposed on this basis. It consists in generating a small initial quasi-random population using Sobol sequence for triggering a Particle Swarm Optimization (PSO) performed over an iteration-dependent cost function defined in terms of the limit state function. The method is evaluated using SVM classifiers, but it can be readily applied also to other statistical classification techniques because the distinctive feature of the SVM, i.e. the margin band, is not actively used in the algorithm. The results show that the method yields results for the probability of failure that are in very close agreement with Monte Carlo simulation performed on the original limit state function and requiring a small number of learning samples.
Journal: Probabilistic Engineering Mechanics - Volume 25, Issue 1, January 2010, Pages 26–34