کد مقاله | کد نشریه | سال انتشار | مقاله انگلیسی | نسخه تمام متن |
---|---|---|---|---|
832297 | 908118 | 2010 | 11 صفحه PDF | دانلود رایگان |
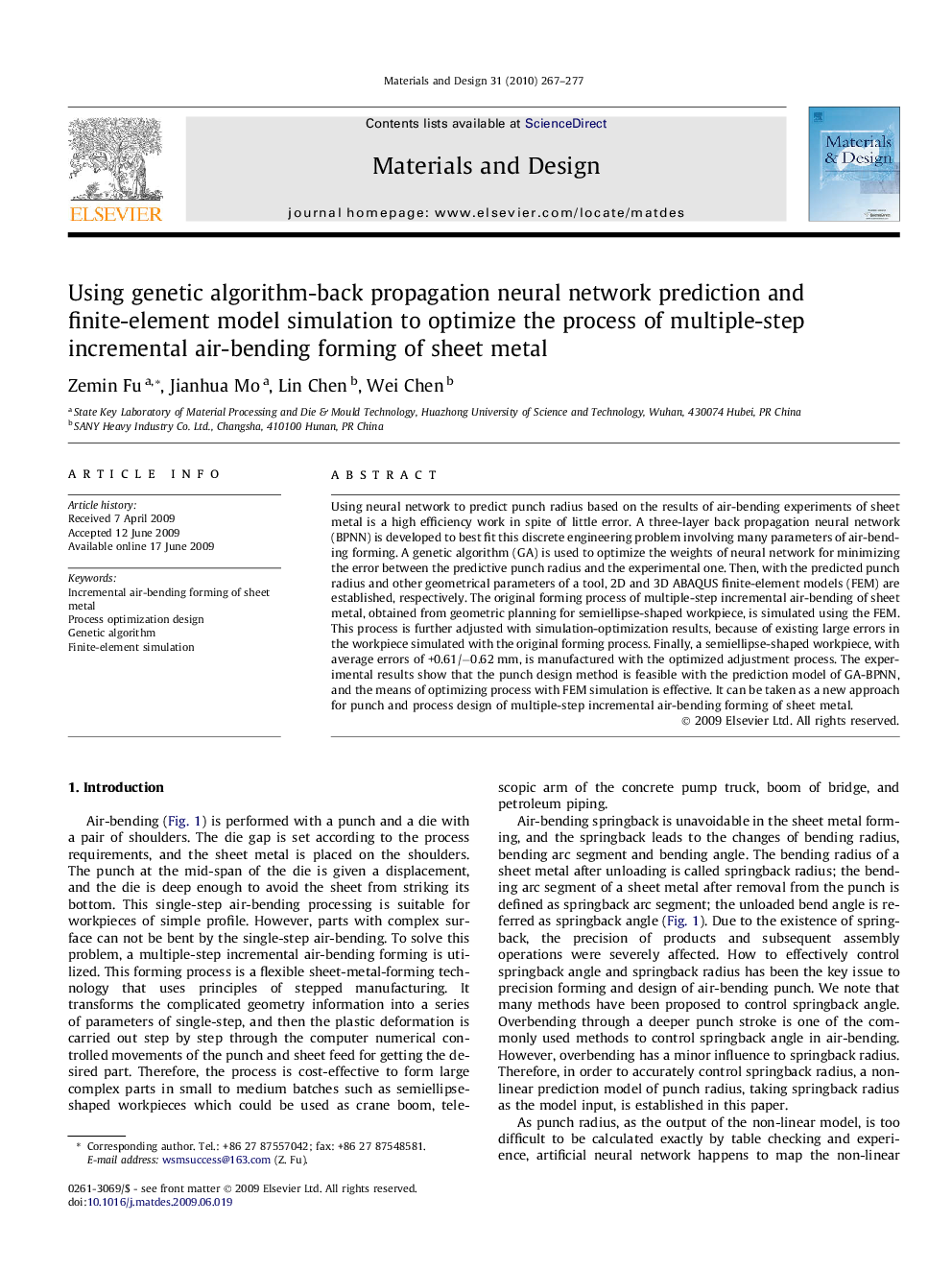
Using neural network to predict punch radius based on the results of air-bending experiments of sheet metal is a high efficiency work in spite of little error. A three-layer back propagation neural network (BPNN) is developed to best fit this discrete engineering problem involving many parameters of air-bending forming. A genetic algorithm (GA) is used to optimize the weights of neural network for minimizing the error between the predictive punch radius and the experimental one. Then, with the predicted punch radius and other geometrical parameters of a tool, 2D and 3D ABAQUS finite-element models (FEM) are established, respectively. The original forming process of multiple-step incremental air-bending of sheet metal, obtained from geometric planning for semiellipse-shaped workpiece, is simulated using the FEM. This process is further adjusted with simulation-optimization results, because of existing large errors in the workpiece simulated with the original forming process. Finally, a semiellipse-shaped workpiece, with average errors of +0.61/−0.62 mm, is manufactured with the optimized adjustment process. The experimental results show that the punch design method is feasible with the prediction model of GA-BPNN, and the means of optimizing process with FEM simulation is effective. It can be taken as a new approach for punch and process design of multiple-step incremental air-bending forming of sheet metal.
Journal: Materials & Design - Volume 31, Issue 1, January 2010, Pages 267–277