کد مقاله | کد نشریه | سال انتشار | مقاله انگلیسی | نسخه تمام متن |
---|---|---|---|---|
8953564 | 1645950 | 2018 | 30 صفحه PDF | دانلود رایگان |
عنوان انگلیسی مقاله ISI
A novel optimized SVM classification algorithm with multi-domain feature and its application to fault diagnosis of rolling bearing
دانلود مقاله + سفارش ترجمه
دانلود مقاله ISI انگلیسی
رایگان برای ایرانیان
کلمات کلیدی
موضوعات مرتبط
مهندسی و علوم پایه
مهندسی کامپیوتر
هوش مصنوعی
پیش نمایش صفحه اول مقاله
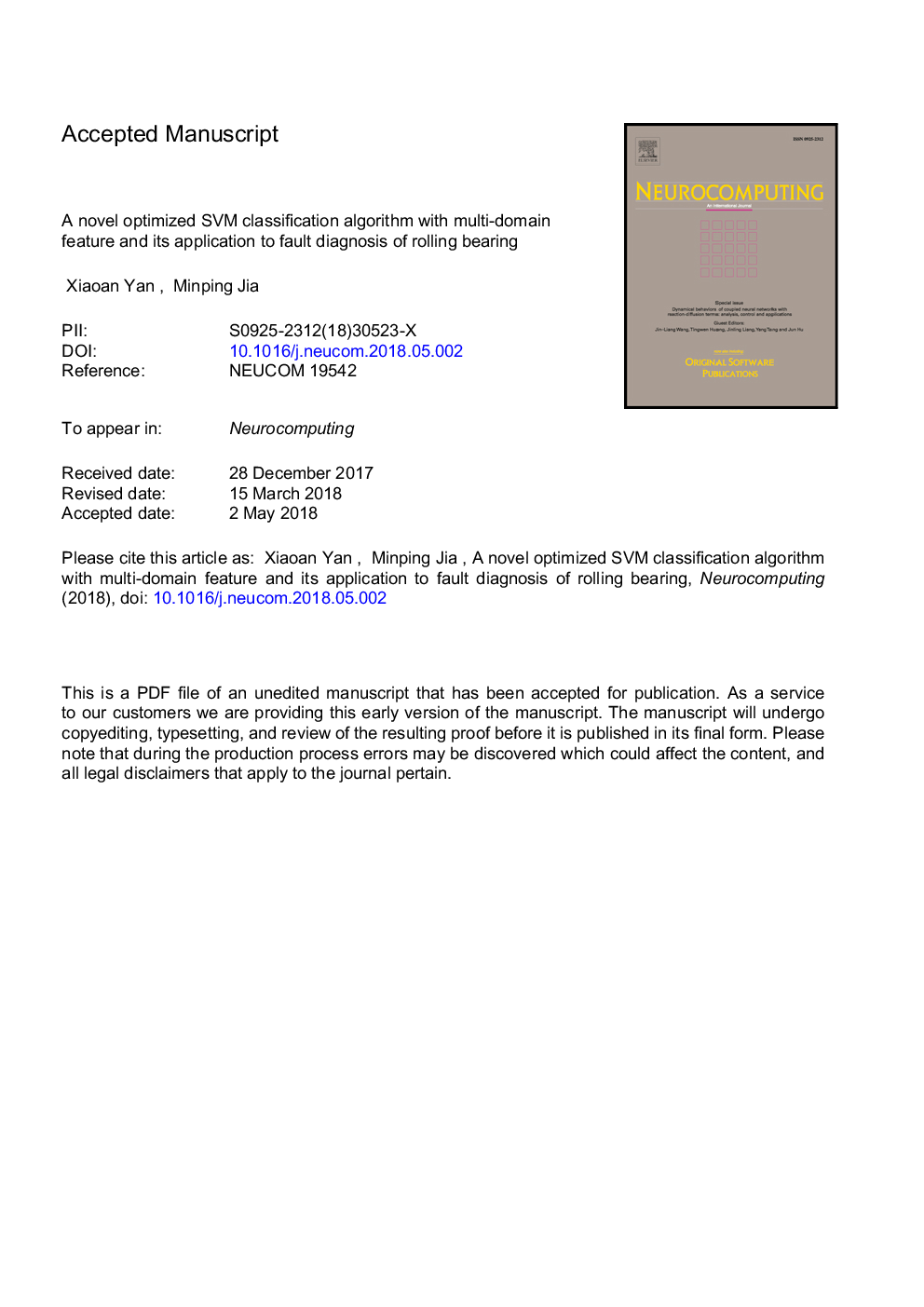
چکیده انگلیسی
Sensitive feature extraction from the raw vibration signal is still a great challenge for intelligent fault diagnosis of rolling bearing. Current fault classification framework generally concentrates on the pattern of classifier with single-domain feature, which is easy to induce insufficient feature extraction and low recognition accuracy. Therefore, to address this issue and improve intelligent diagnostic accuracy of rolling bearing, this paper proposes a novel fault classification algorithm based on optimized SVM with multi-domain feature, which mainly consists of three stages (i.e. multi-domain feature extraction, feature selection and fault identification). In this first stage, three approaches (i.e. statistical analysis, FFT and VMD) are separately applied to extract the fault feature information from multi-domain aspect (e.g. time-domain, frequency-domain and time-frequency domain), which can excavate comprehensively the condition information and intrinsic property of the raw vibration signal. Secondly, Laplace score algorithm is introduced to select automatically the meaningful sensitive feature according to the importance of each feature, which is aimed at removing some redundant information and improving the calculation efficiency. Finally, particle swarm optimization-based support vector machine (PSO-SVM) classification model is employed to implement the identification of multiple fault condition of rolling bearing. Performance of the proposed method is evaluated on two experimental examples of rolling bearing fault diagnosis. Experimental results show that the proposed method achieves high diagnosis accuracy for different working conditions of rolling bearing and outperforms some traditional methods both mentioned in this paper and published in other literature.
ناشر
Database: Elsevier - ScienceDirect (ساینس دایرکت)
Journal: Neurocomputing - Volume 313, 3 November 2018, Pages 47-64
Journal: Neurocomputing - Volume 313, 3 November 2018, Pages 47-64
نویسندگان
Yan Xiaoan, Jia Minping,