کد مقاله | کد نشریه | سال انتشار | مقاله انگلیسی | نسخه تمام متن |
---|---|---|---|---|
9653607 | 679206 | 2005 | 23 صفحه PDF | دانلود رایگان |
عنوان انگلیسی مقاله ISI
Asynchronous neurocomputing for optimal control and reinforcement learning with large state spaces
دانلود مقاله + سفارش ترجمه
دانلود مقاله ISI انگلیسی
رایگان برای ایرانیان
موضوعات مرتبط
مهندسی و علوم پایه
مهندسی کامپیوتر
هوش مصنوعی
پیش نمایش صفحه اول مقاله
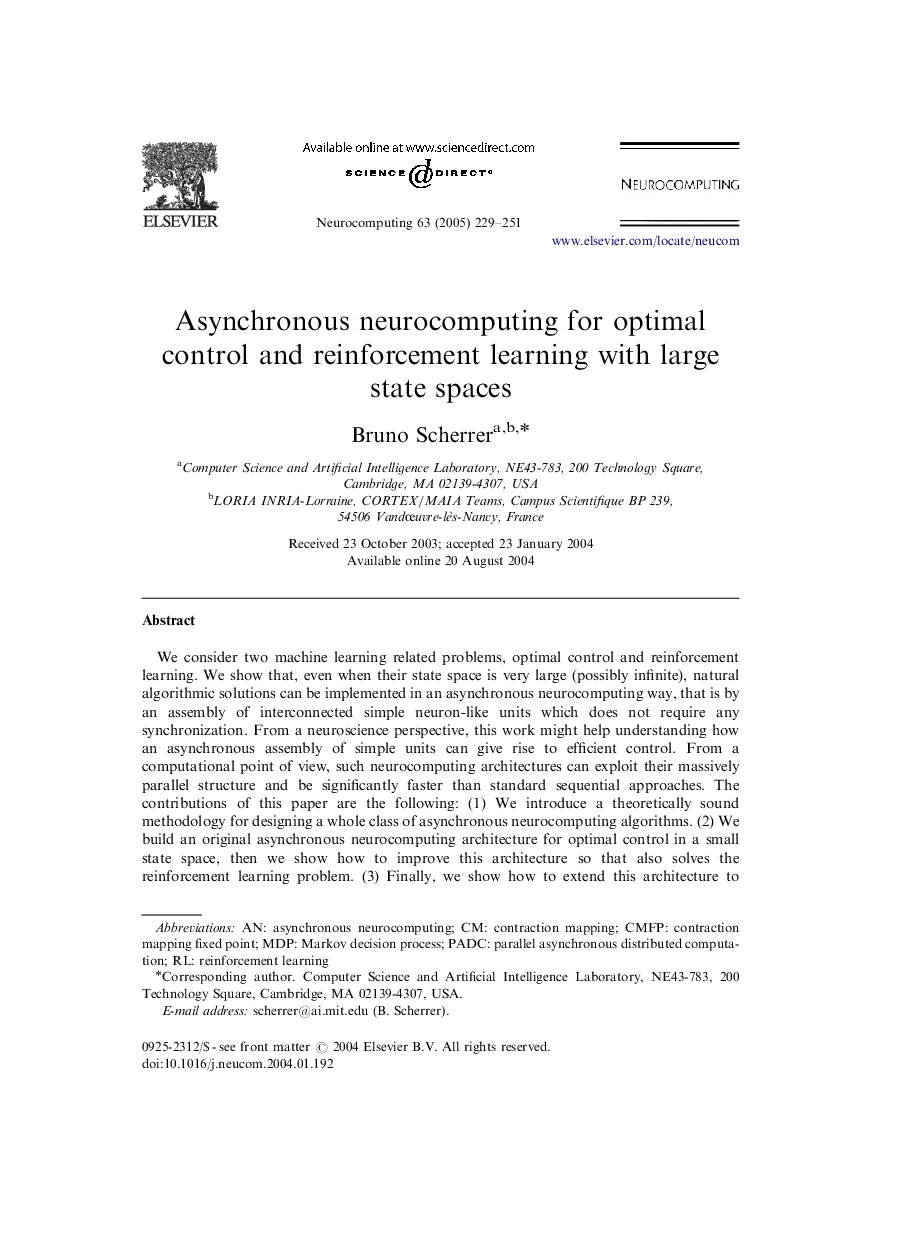
چکیده انگلیسی
We consider two machine learning related problems, optimal control and reinforcement learning. We show that, even when their state space is very large (possibly infinite), natural algorithmic solutions can be implemented in an asynchronous neurocomputing way, that is by an assembly of interconnected simple neuron-like units which does not require any synchronization. From a neuroscience perspective, this work might help understanding how an asynchronous assembly of simple units can give rise to efficient control. From a computational point of view, such neurocomputing architectures can exploit their massively parallel structure and be significantly faster than standard sequential approaches. The contributions of this paper are the following: (1) We introduce a theoretically sound methodology for designing a whole class of asynchronous neurocomputing algorithms. (2) We build an original asynchronous neurocomputing architecture for optimal control in a small state space, then we show how to improve this architecture so that also solves the reinforcement learning problem. (3) Finally, we show how to extend this architecture to address the case where the state space is large (possibly infinite) by using an asynchronous neurocomputing adaptive approximation scheme. We illustrate this approximation scheme on two continuous space control problems.
ناشر
Database: Elsevier - ScienceDirect (ساینس دایرکت)
Journal: Neurocomputing - Volume 63, January 2005, Pages 229-251
Journal: Neurocomputing - Volume 63, January 2005, Pages 229-251
نویسندگان
Bruno Scherrer,