کد مقاله | کد نشریه | سال انتشار | مقاله انگلیسی | نسخه تمام متن |
---|---|---|---|---|
11012516 | 1798843 | 2019 | 28 صفحه PDF | دانلود رایگان |
عنوان انگلیسی مقاله ISI
DCDG-EA: Dynamic convergence-diversity guided evolutionary algorithm for many-objective optimization
دانلود مقاله + سفارش ترجمه
دانلود مقاله ISI انگلیسی
رایگان برای ایرانیان
کلمات کلیدی
موضوعات مرتبط
مهندسی و علوم پایه
مهندسی کامپیوتر
هوش مصنوعی
پیش نمایش صفحه اول مقاله
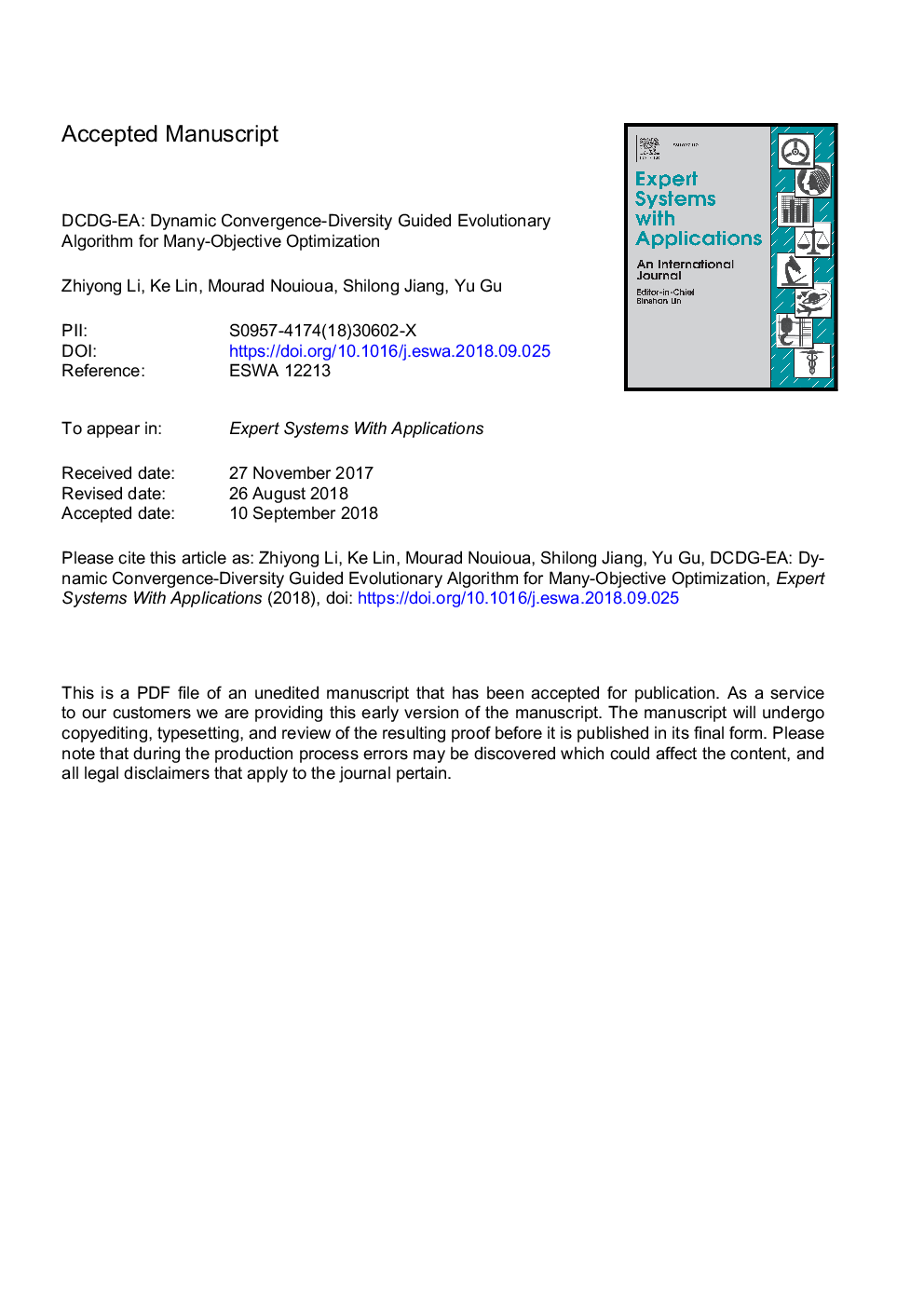
چکیده انگلیسی
Maintaining a good balance between the convergence and the diversity is particularly crucial for the performance of the evolutionary algorithms (EAs). However, the traditional multi-objective evolutionary algorithms, which have shown their competitive performance with a variety of practical problems involving two or three objectives, face significant challenges in case of problems with more than three objectives, namely many-objective optimization problems (MaOPs). This paper proposes a dynamic convergence-diversity guided evolutionary algorithm, namely (DCDG-EA) for MaOPs by employing the decomposition technique. Besides, the objective space of MaOPs is divided into K subspaces by a set of uniformly distributed reference vectors. Each subspace has its own subpopulation and evolves in parallel with the other subspaces. In DCDG-EA, the balance between the convergence and the diversity is achieved through the convergence-diversity based operator selection (CDOS) strategy and convergence-diversity based individual selection (CDIS) strategy. In CDOS, for each operator of the set of operators, a selection probability is assigned which is related to its convergence and diversity capabilities. Based on the attributed selection probabilities, an appropriate operator is selected to generate the offsprings. Furthermore, CDIS is used which allows to greatly overcome the inefficiency of the Pareto dominance approaches. It updates each subpopulation by using two independent distance measures that represent the convergence and the control diversity, respectively. The experimental results on DTLZ and WFG benchmark problems with up to 15 objectives show that our algorithm is highly competitive comparing with the four state-of-the-art evolutionary algorithms in terms of convergence and diversity.
ناشر
Database: Elsevier - ScienceDirect (ساینس دایرکت)
Journal: Expert Systems with Applications - Volume 118, 15 March 2019, Pages 35-51
Journal: Expert Systems with Applications - Volume 118, 15 March 2019, Pages 35-51
نویسندگان
Zhiyong Li, Ke Lin, Mourad Nouioua, Shilong Jiang, Yu Gu,