کد مقاله | کد نشریه | سال انتشار | مقاله انگلیسی | نسخه تمام متن |
---|---|---|---|---|
11021164 | 1715033 | 2018 | 31 صفحه PDF | دانلود رایگان |
عنوان انگلیسی مقاله ISI
Unsupervised pixel-wise classification for Chaetoceros image segmentation
دانلود مقاله + سفارش ترجمه
دانلود مقاله ISI انگلیسی
رایگان برای ایرانیان
کلمات کلیدی
موضوعات مرتبط
مهندسی و علوم پایه
مهندسی کامپیوتر
هوش مصنوعی
پیش نمایش صفحه اول مقاله
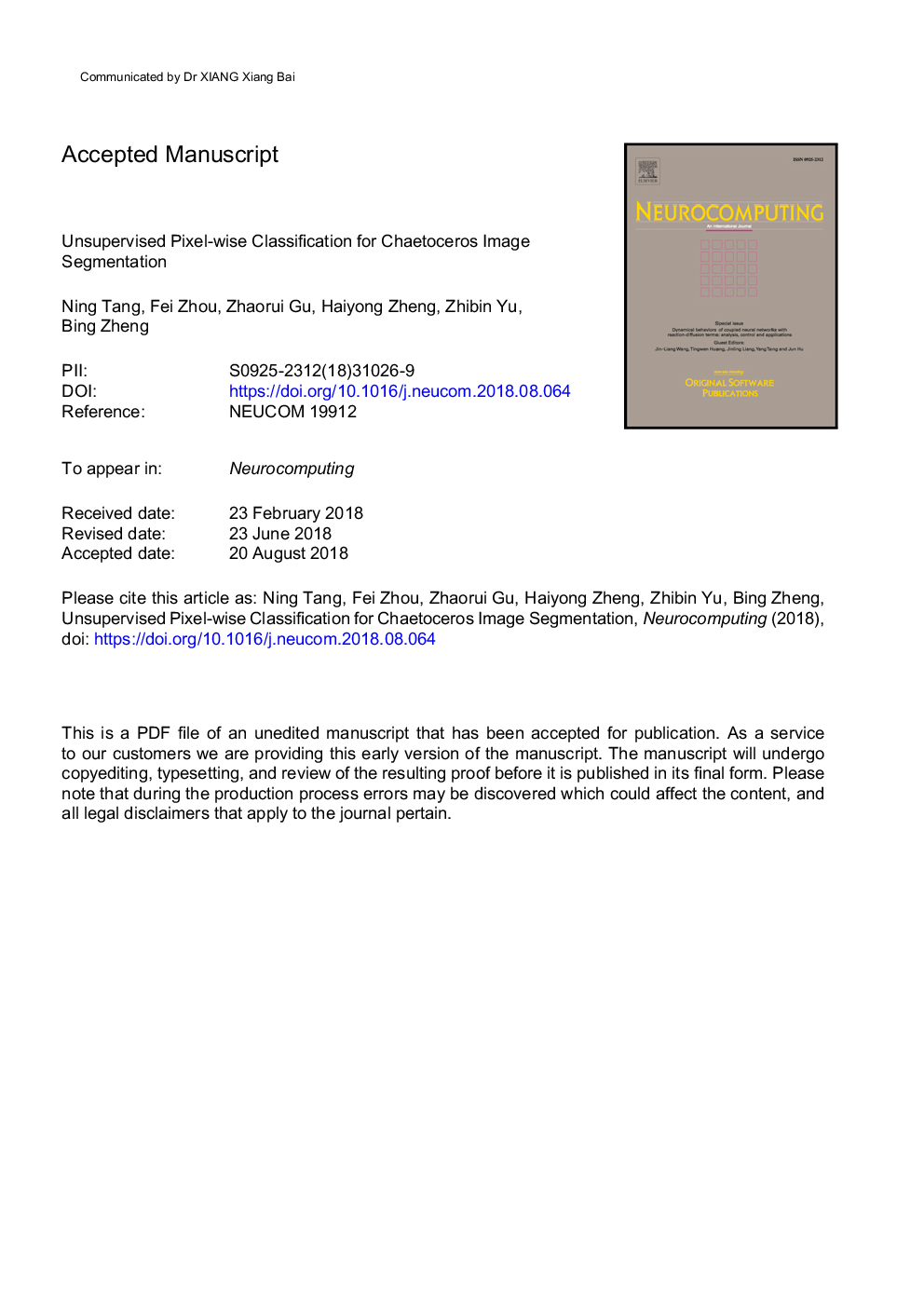
چکیده انگلیسی
Chaetoceros is a dominant genus of marine planktonic diatoms with worldwide distribution. Due to the difficulty of extracting setae from Chaetoceros images, automatic segmentation of Chaetoceros is still a challenging task. In this paper, we address this difficult task by regarding the whole segmentation process as unsupervised pixel-wise classification without human participation. First, we automatically produce positive (object) and negative (background) samples for follow-up training, by combining the advantages of two image processing algorithms: Grayscale Surface Direction Angle Model (GSDAM) for extracting setae information and Canny for detecting cell edges from low-contrast and strong-noisy microscopic images. Second, we develop pixel-wise training by using the produced samples in the training process of Deep Convolutional Neural Network (DCNN). At last, the trained DCNN is used to label other pixels into object and background for final segmentation. We compare our method with eight mainstream segmentation approaches: Otsu's thresholding, Canny, Watershed, Mean Shift, gPb-owt-ucm, Normalized Cut, Efficient Graph-based method and GSDAM. To objectively evaluate segmentation results, we apply six well-known evaluation indexes. Experimental results on a new Chaetoceros image dataset with human labelled ground truth show that our method outperforms the eight mainstream segmentation methods in terms of both quantitative and qualitative evaluation.
ناشر
Database: Elsevier - ScienceDirect (ساینس دایرکت)
Journal: Neurocomputing - Volume 318, 27 November 2018, Pages 261-270
Journal: Neurocomputing - Volume 318, 27 November 2018, Pages 261-270
نویسندگان
Ning Tang, Fei Zhou, Zhaorui Gu, Haiyong Zheng, Zhibin Yu, Bing Zheng,