کد مقاله | کد نشریه | سال انتشار | مقاله انگلیسی | نسخه تمام متن |
---|---|---|---|---|
1145888 | 1489683 | 2013 | 14 صفحه PDF | دانلود رایگان |
عنوان انگلیسی مقاله ISI
Mixtures of common factor analyzers for high-dimensional data with missing information
دانلود مقاله + سفارش ترجمه
دانلود مقاله ISI انگلیسی
رایگان برای ایرانیان
کلمات کلیدی
موضوعات مرتبط
مهندسی و علوم پایه
ریاضیات
آنالیز عددی
پیش نمایش صفحه اول مقاله
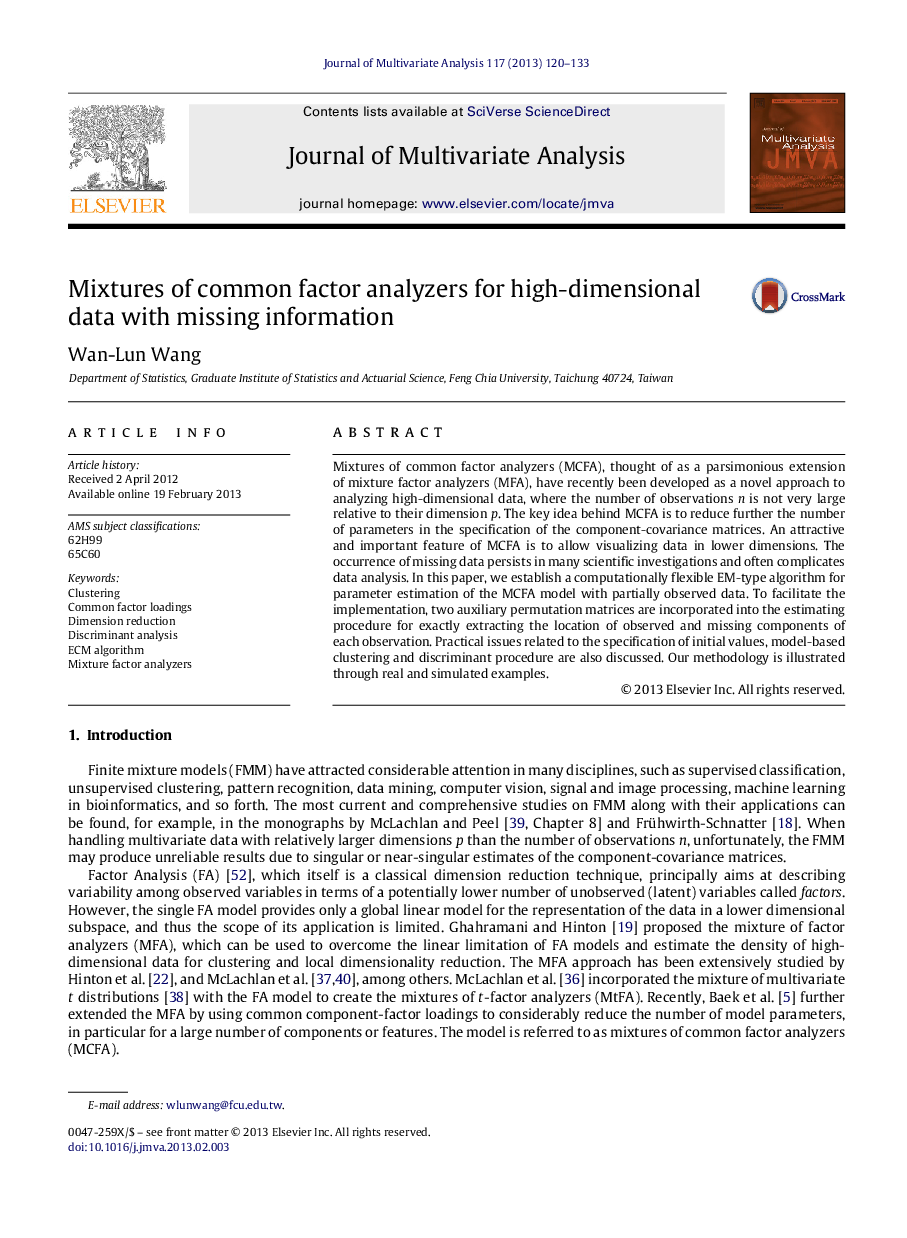
چکیده انگلیسی
Mixtures of common factor analyzers (MCFA), thought of as a parsimonious extension of mixture factor analyzers (MFA), have recently been developed as a novel approach to analyzing high-dimensional data, where the number of observations n is not very large relative to their dimension p. The key idea behind MCFA is to reduce further the number of parameters in the specification of the component-covariance matrices. An attractive and important feature of MCFA is to allow visualizing data in lower dimensions. The occurrence of missing data persists in many scientific investigations and often complicates data analysis. In this paper, we establish a computationally flexible EM-type algorithm for parameter estimation of the MCFA model with partially observed data. To facilitate the implementation, two auxiliary permutation matrices are incorporated into the estimating procedure for exactly extracting the location of observed and missing components of each observation. Practical issues related to the specification of initial values, model-based clustering and discriminant procedure are also discussed. Our methodology is illustrated through real and simulated examples.
ناشر
Database: Elsevier - ScienceDirect (ساینس دایرکت)
Journal: Journal of Multivariate Analysis - Volume 117, May 2013, Pages 120-133
Journal: Journal of Multivariate Analysis - Volume 117, May 2013, Pages 120-133
نویسندگان
Wan-Lun Wang,