کد مقاله | کد نشریه | سال انتشار | مقاله انگلیسی | نسخه تمام متن |
---|---|---|---|---|
1712799 | 1013161 | 2006 | 11 صفحه PDF | دانلود رایگان |
عنوان انگلیسی مقاله ISI
Daily Stream Flow Prediction Capability of Artificial Neural Networks as influenced by Minimum Air Temperature Data
دانلود مقاله + سفارش ترجمه
دانلود مقاله ISI انگلیسی
رایگان برای ایرانیان
موضوعات مرتبط
مهندسی و علوم پایه
سایر رشته های مهندسی
کنترل و سیستم های مهندسی
پیش نمایش صفحه اول مقاله
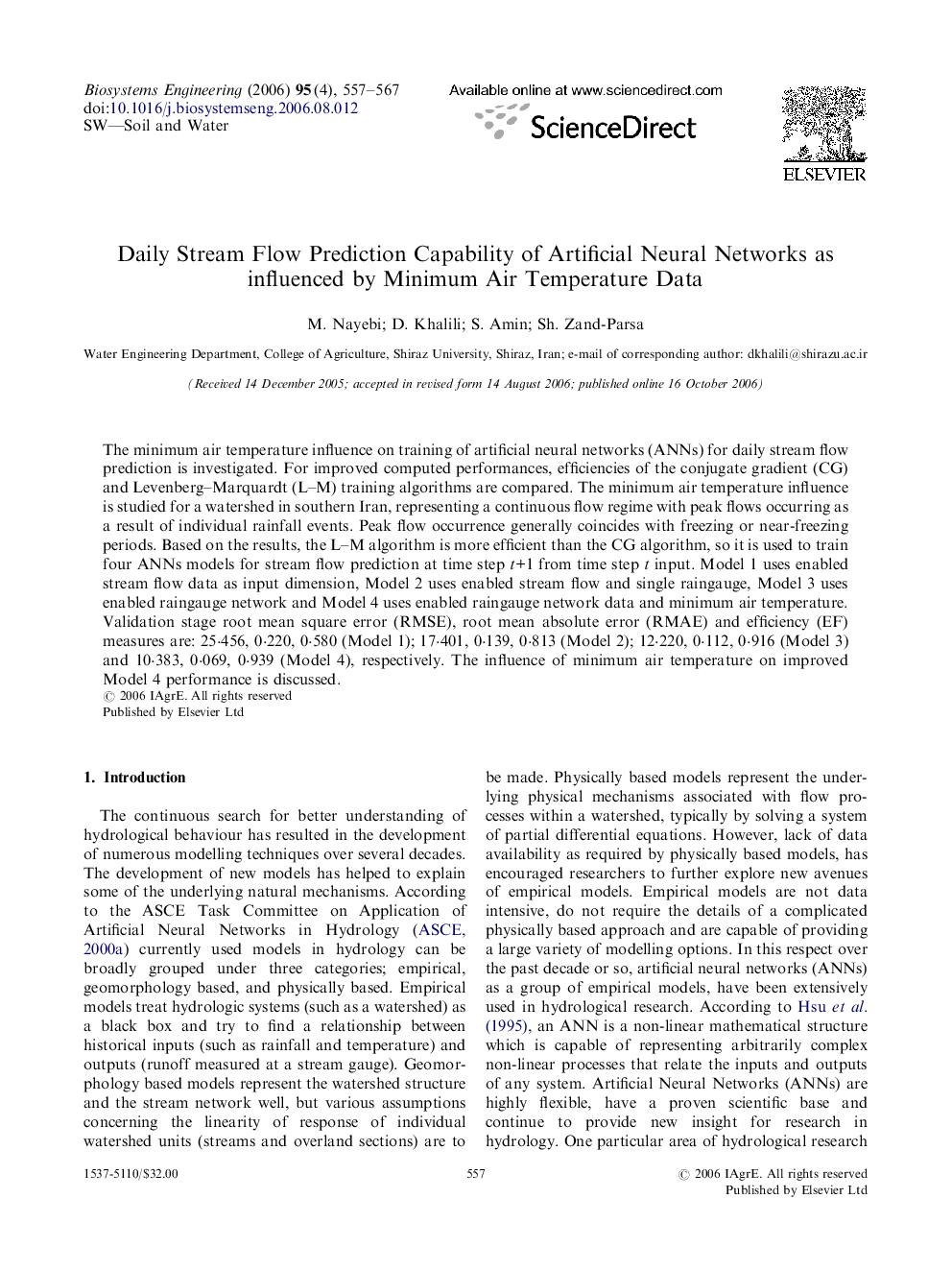
چکیده انگلیسی
The minimum air temperature influence on training of artificial neural networks (ANNs) for daily stream flow prediction is investigated. For improved computed performances, efficiencies of the conjugate gradient (CG) and Levenberg-Marquardt (L-M) training algorithms are compared. The minimum air temperature influence is studied for a watershed in southern Iran, representing a continuous flow regime with peak flows occurring as a result of individual rainfall events. Peak flow occurrence generally coincides with freezing or near-freezing periods. Based on the results, the L-M algorithm is more efficient than the CG algorithm, so it is used to train four ANNs models for stream flow prediction at time step t+1 from time step t input. Model 1 uses enabled stream flow data as input dimension, Model 2 uses enabled stream flow and single raingauge, Model 3 uses enabled raingauge network and Model 4 uses enabled raingauge network data and minimum air temperature. Validation stage root mean square error (RMSE), root mean absolute error (RMAE) and efficiency (EF) measures are: 25·456, 0·220, 0·580 (Model 1); 17·401, 0·139, 0·813 (Model 2); 12·220, 0·112, 0·916 (Model 3) and 10·383, 0·069, 0·939 (Model 4), respectively. The influence of minimum air temperature on improved Model 4 performance is discussed.
ناشر
Database: Elsevier - ScienceDirect (ساینس دایرکت)
Journal: Biosystems Engineering - Volume 95, Issue 4, December 2006, Pages 557-567
Journal: Biosystems Engineering - Volume 95, Issue 4, December 2006, Pages 557-567
نویسندگان
M. Nayebi, D. Khalili, S. Amin, Sh. Zand-Parsa,