کد مقاله | کد نشریه | سال انتشار | مقاله انگلیسی | نسخه تمام متن |
---|---|---|---|---|
231801 | 1427451 | 2008 | 6 صفحه PDF | دانلود رایگان |
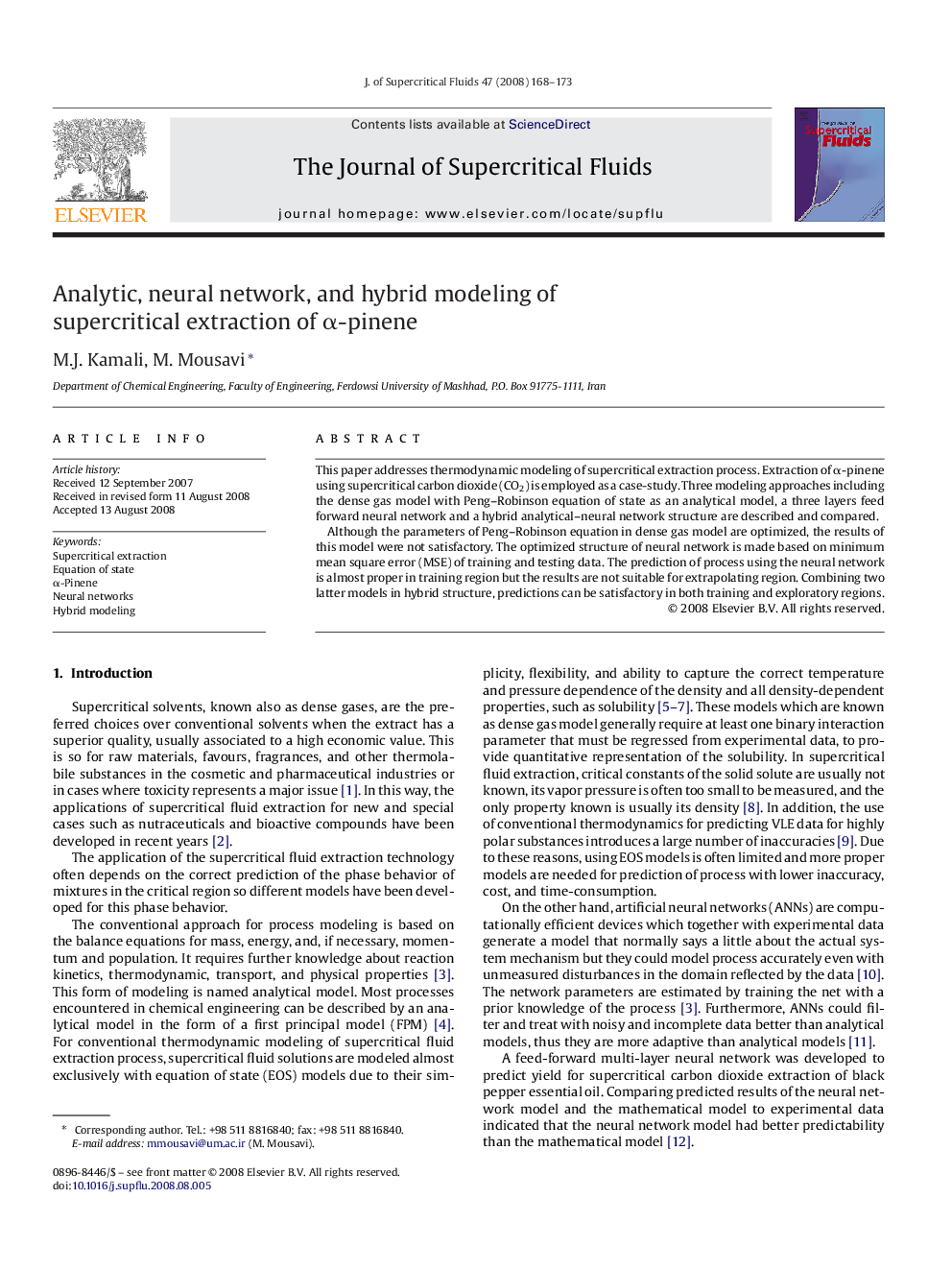
This paper addresses thermodynamic modeling of supercritical extraction process. Extraction of α-pinene using supercritical carbon dioxide (CO2) is employed as a case-study. Three modeling approaches including the dense gas model with Peng–Robinson equation of state as an analytical model, a three layers feed forward neural network and a hybrid analytical–neural network structure are described and compared.Although the parameters of Peng–Robinson equation in dense gas model are optimized, the results of this model were not satisfactory. The optimized structure of neural network is made based on minimum mean square error (MSE) of training and testing data. The prediction of process using the neural network is almost proper in training region but the results are not suitable for extrapolating region. Combining two latter models in hybrid structure, predictions can be satisfactory in both training and exploratory regions.
In this paper, three modeling approaches including the dense gas model with Peng–Robinson equation of state as an analytical model, a three layers feed forward neural network, and a hybrid analytical–neural network structure are described and compared in order to model supercritical extraction α-pinene using supercritical carbon dioxide. The predictions of hybrid model are more satisfactory.Figure optionsDownload as PowerPoint slide
Journal: The Journal of Supercritical Fluids - Volume 47, Issue 2, December 2008, Pages 168–173