کد مقاله | کد نشریه | سال انتشار | مقاله انگلیسی | نسخه تمام متن |
---|---|---|---|---|
2589643 | 1562054 | 2014 | 11 صفحه PDF | دانلود رایگان |
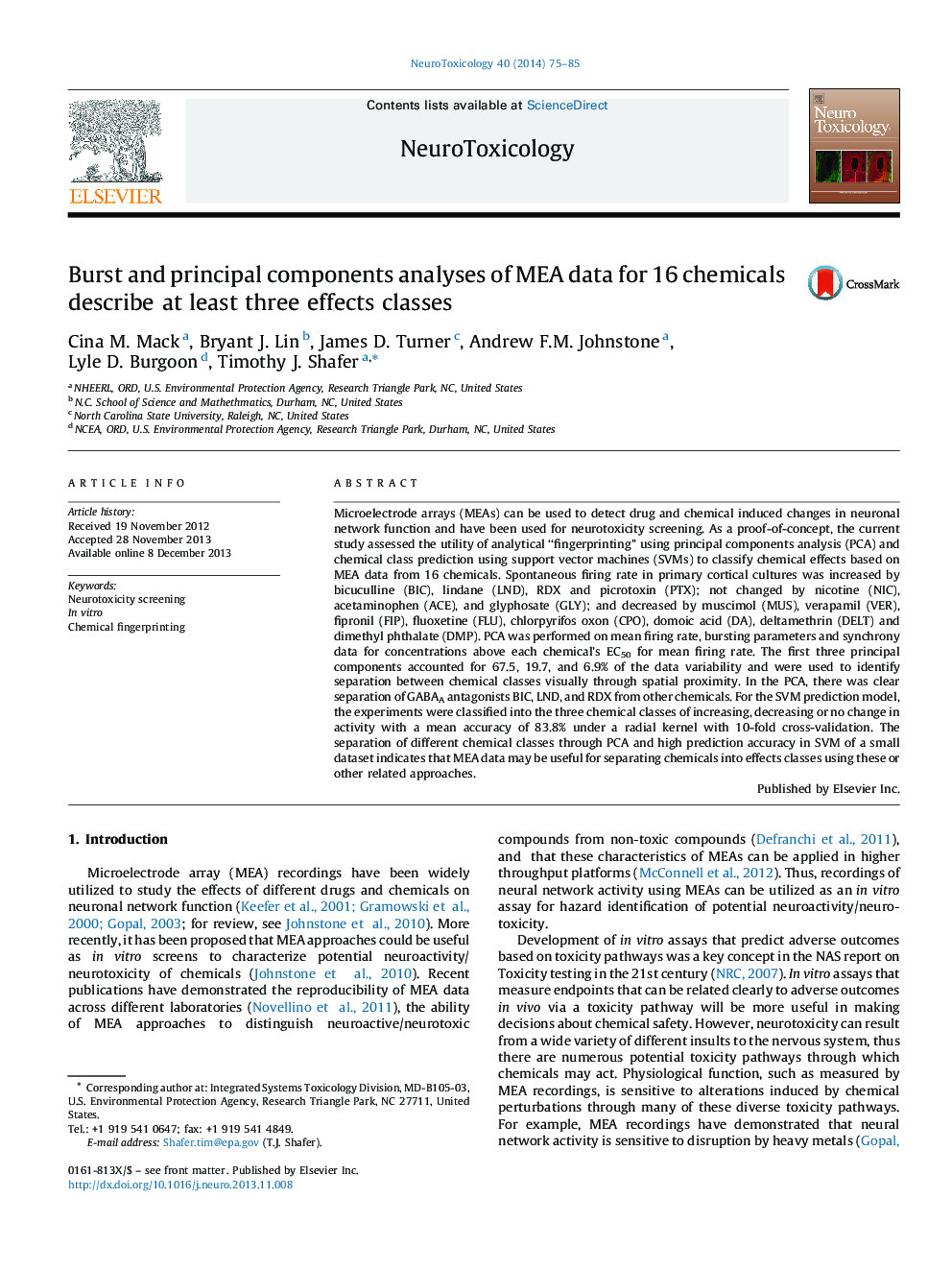
• Spontaneous activity in cortical networks in vitro was recorded with microelectrode arrays.
• Effects of 16 chemicals on firing rate, bursting parameters and network synchrony were determined.
• Principal components analysis and support vector machine analysis of the data were conducted.
• PCA separated some GABAA antagonists from other chemicals and SVM predicted three effects classes.
• These data indicate that MEA data may be useful to separate compounds by class.
Microelectrode arrays (MEAs) can be used to detect drug and chemical induced changes in neuronal network function and have been used for neurotoxicity screening. As a proof-of-concept, the current study assessed the utility of analytical “fingerprinting” using principal components analysis (PCA) and chemical class prediction using support vector machines (SVMs) to classify chemical effects based on MEA data from 16 chemicals. Spontaneous firing rate in primary cortical cultures was increased by bicuculline (BIC), lindane (LND), RDX and picrotoxin (PTX); not changed by nicotine (NIC), acetaminophen (ACE), and glyphosate (GLY); and decreased by muscimol (MUS), verapamil (VER), fipronil (FIP), fluoxetine (FLU), chlorpyrifos oxon (CPO), domoic acid (DA), deltamethrin (DELT) and dimethyl phthalate (DMP). PCA was performed on mean firing rate, bursting parameters and synchrony data for concentrations above each chemical's EC50 for mean firing rate. The first three principal components accounted for 67.5, 19.7, and 6.9% of the data variability and were used to identify separation between chemical classes visually through spatial proximity. In the PCA, there was clear separation of GABAA antagonists BIC, LND, and RDX from other chemicals. For the SVM prediction model, the experiments were classified into the three chemical classes of increasing, decreasing or no change in activity with a mean accuracy of 83.8% under a radial kernel with 10-fold cross-validation. The separation of different chemical classes through PCA and high prediction accuracy in SVM of a small dataset indicates that MEA data may be useful for separating chemicals into effects classes using these or other related approaches.
Journal: NeuroToxicology - Volume 40, January 2014, Pages 75–85