کد مقاله | کد نشریه | سال انتشار | مقاله انگلیسی | نسخه تمام متن |
---|---|---|---|---|
3052383 | 1579927 | 2011 | 10 صفحه PDF | دانلود رایگان |
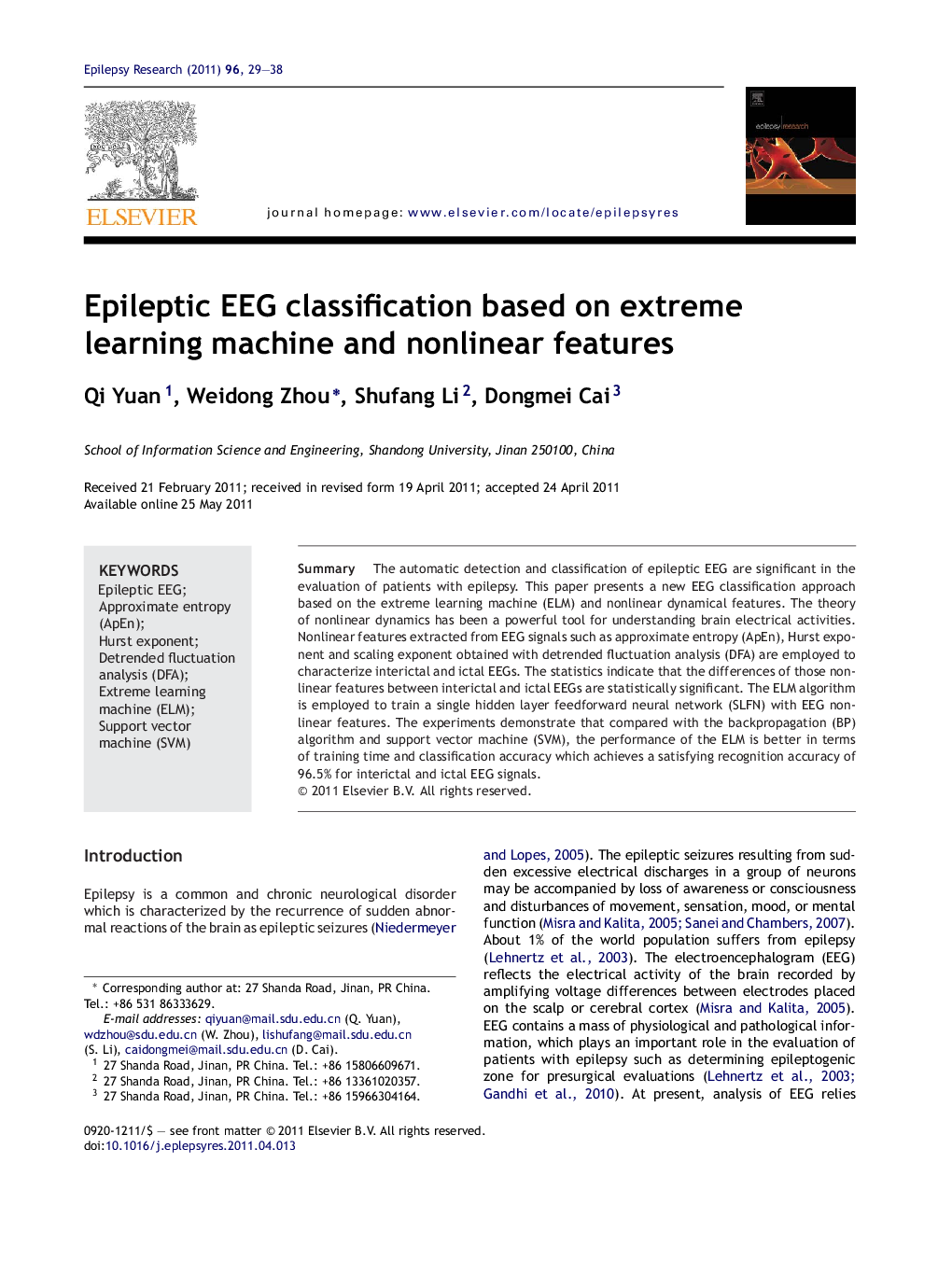
SummaryThe automatic detection and classification of epileptic EEG are significant in the evaluation of patients with epilepsy. This paper presents a new EEG classification approach based on the extreme learning machine (ELM) and nonlinear dynamical features. The theory of nonlinear dynamics has been a powerful tool for understanding brain electrical activities. Nonlinear features extracted from EEG signals such as approximate entropy (ApEn), Hurst exponent and scaling exponent obtained with detrended fluctuation analysis (DFA) are employed to characterize interictal and ictal EEGs. The statistics indicate that the differences of those nonlinear features between interictal and ictal EEGs are statistically significant. The ELM algorithm is employed to train a single hidden layer feedforward neural network (SLFN) with EEG nonlinear features. The experiments demonstrate that compared with the backpropagation (BP) algorithm and support vector machine (SVM), the performance of the ELM is better in terms of training time and classification accuracy which achieves a satisfying recognition accuracy of 96.5% for interictal and ictal EEG signals.
Journal: Epilepsy Research - Volume 96, Issues 1–2, September 2011, Pages 29–38