کد مقاله | کد نشریه | سال انتشار | مقاله انگلیسی | نسخه تمام متن |
---|---|---|---|---|
378117 | 658883 | 2007 | 13 صفحه PDF | دانلود رایگان |
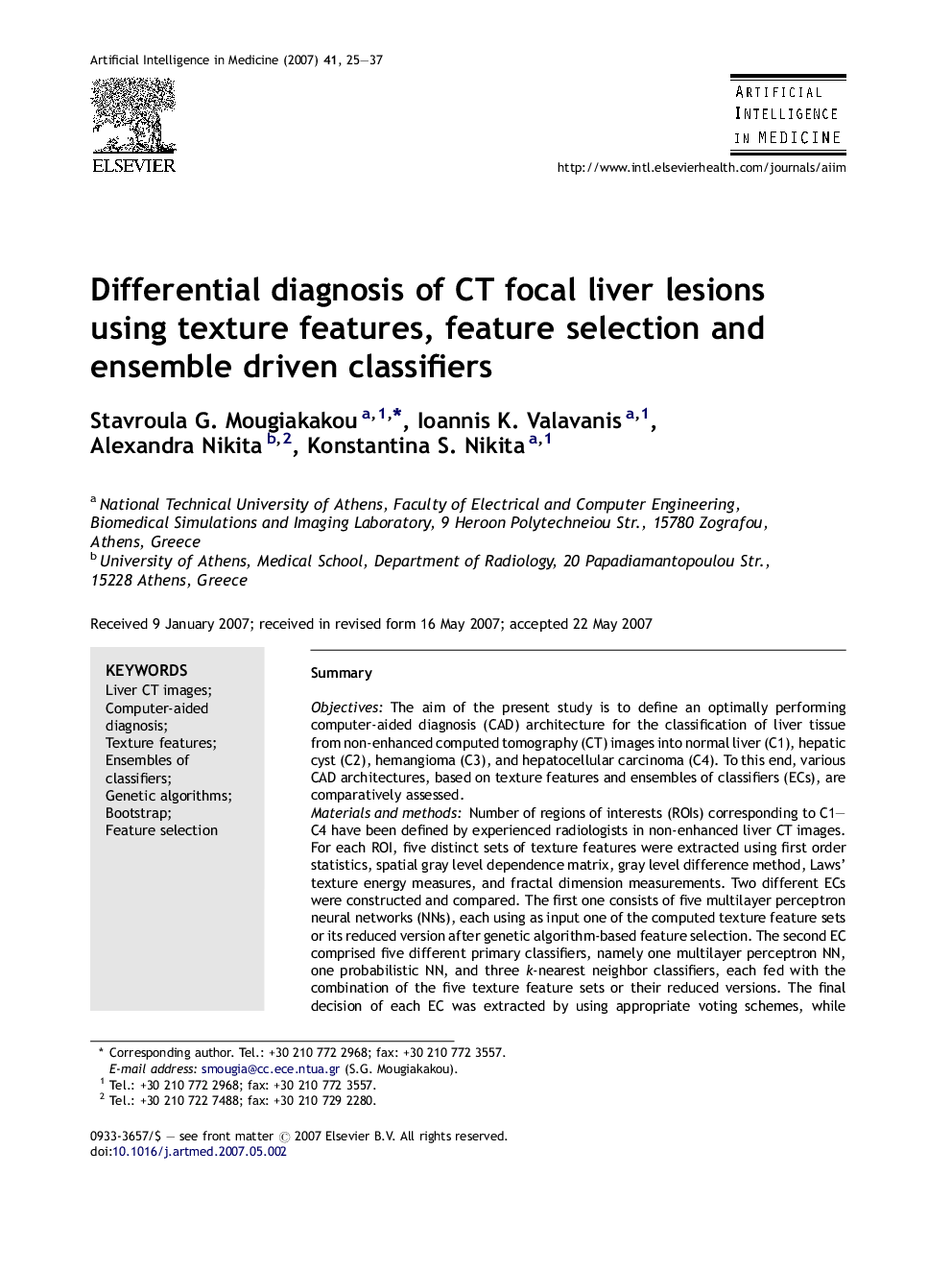
SummaryObjectivesThe aim of the present study is to define an optimally performing computer-aided diagnosis (CAD) architecture for the classification of liver tissue from non-enhanced computed tomography (CT) images into normal liver (C1), hepatic cyst (C2), hemangioma (C3), and hepatocellular carcinoma (C4). To this end, various CAD architectures, based on texture features and ensembles of classifiers (ECs), are comparatively assessed.Materials and methodsNumber of regions of interests (ROIs) corresponding to C1–C4 have been defined by experienced radiologists in non-enhanced liver CT images. For each ROI, five distinct sets of texture features were extracted using first order statistics, spatial gray level dependence matrix, gray level difference method, Laws’ texture energy measures, and fractal dimension measurements. Two different ECs were constructed and compared. The first one consists of five multilayer perceptron neural networks (NNs), each using as input one of the computed texture feature sets or its reduced version after genetic algorithm-based feature selection. The second EC comprised five different primary classifiers, namely one multilayer perceptron NN, one probabilistic NN, and three k-nearest neighbor classifiers, each fed with the combination of the five texture feature sets or their reduced versions. The final decision of each EC was extracted by using appropriate voting schemes, while bootstrap re-sampling was utilized in order to estimate the generalization ability of the CAD architectures based on the available relatively small-sized data set.ResultsThe best mean classification accuracy (84.96%) is achieved by the second EC using a fused feature set, and the weighted voting scheme. The fused feature set was obtained after appropriate feature selection applied to specific subsets of the original feature set.ConclusionsThe comparative assessment of the various CAD architectures shows that combining three types of classifiers with a voting scheme, fed with identical feature sets obtained after appropriate feature selection and fusion, may result in an accurate system able to assist differential diagnosis of focal liver lesions from non-enhanced CT images.
Journal: Artificial Intelligence in Medicine - Volume 41, Issue 1, September 2007, Pages 25–37