کد مقاله | کد نشریه | سال انتشار | مقاله انگلیسی | نسخه تمام متن |
---|---|---|---|---|
382963 | 660798 | 2015 | 16 صفحه PDF | دانلود رایگان |
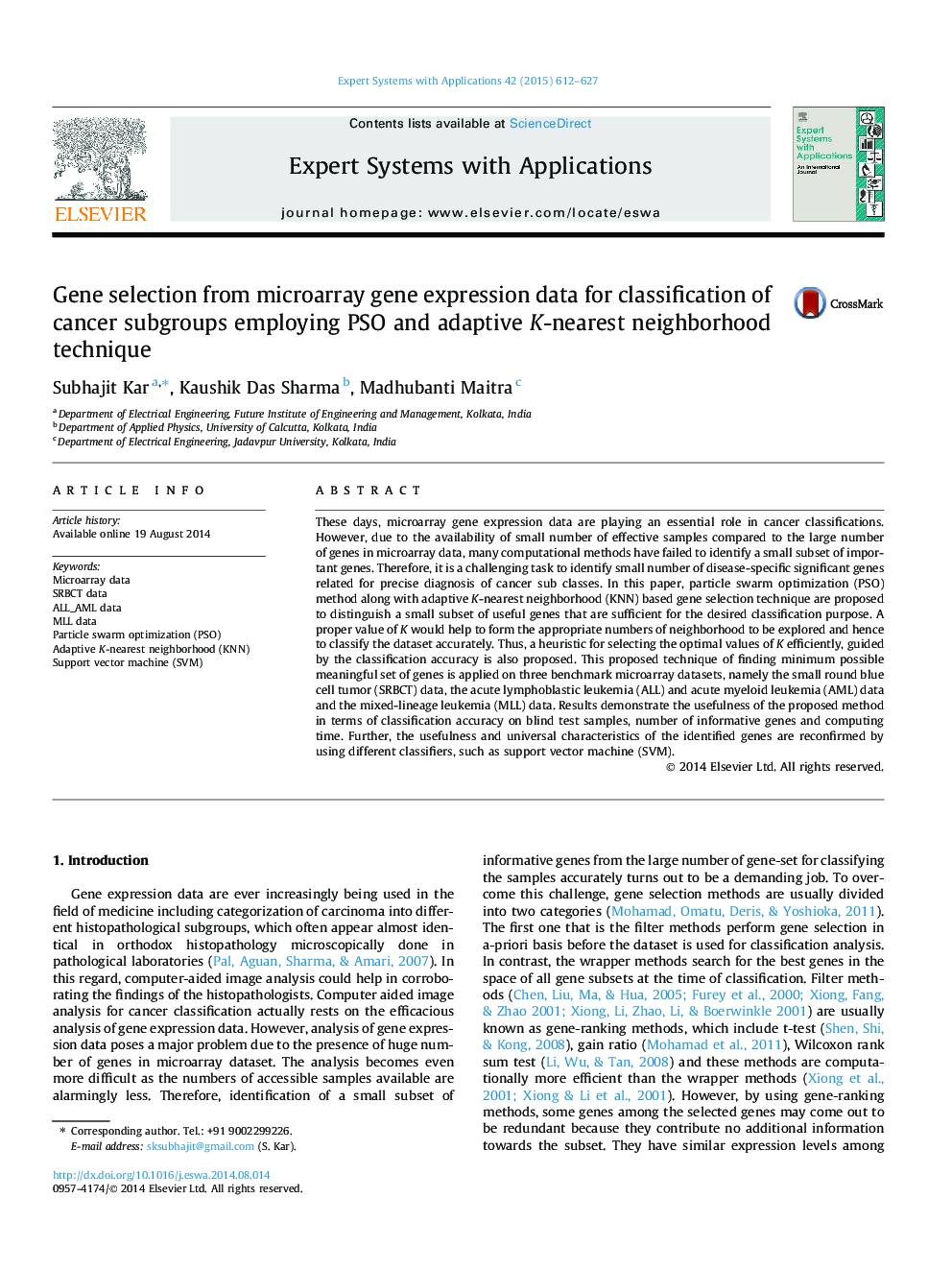
• A PSO–adaptive KNN based gene selection method is proposed to select useful genes.
• A heuristic for selecting the optimal values of K efficiently is also proposed.
• The proposed technique is applied on SRBCT, ALL_AML and MLL microarray datasets.
• The usefulness of the identified genes is reconfirmed using SVM classifier.
• The method finds 6, 3 and 4 genes for SRBCT, ALL_AML, and MLL with high accuracy.
These days, microarray gene expression data are playing an essential role in cancer classifications. However, due to the availability of small number of effective samples compared to the large number of genes in microarray data, many computational methods have failed to identify a small subset of important genes. Therefore, it is a challenging task to identify small number of disease-specific significant genes related for precise diagnosis of cancer sub classes. In this paper, particle swarm optimization (PSO) method along with adaptive K-nearest neighborhood (KNN) based gene selection technique are proposed to distinguish a small subset of useful genes that are sufficient for the desired classification purpose. A proper value of K would help to form the appropriate numbers of neighborhood to be explored and hence to classify the dataset accurately. Thus, a heuristic for selecting the optimal values of K efficiently, guided by the classification accuracy is also proposed. This proposed technique of finding minimum possible meaningful set of genes is applied on three benchmark microarray datasets, namely the small round blue cell tumor (SRBCT) data, the acute lymphoblastic leukemia (ALL) and acute myeloid leukemia (AML) data and the mixed-lineage leukemia (MLL) data. Results demonstrate the usefulness of the proposed method in terms of classification accuracy on blind test samples, number of informative genes and computing time. Further, the usefulness and universal characteristics of the identified genes are reconfirmed by using different classifiers, such as support vector machine (SVM).
Journal: Expert Systems with Applications - Volume 42, Issue 1, January 2015, Pages 612–627