کد مقاله | کد نشریه | سال انتشار | مقاله انگلیسی | نسخه تمام متن |
---|---|---|---|---|
385635 | 660869 | 2011 | 6 صفحه PDF | دانلود رایگان |
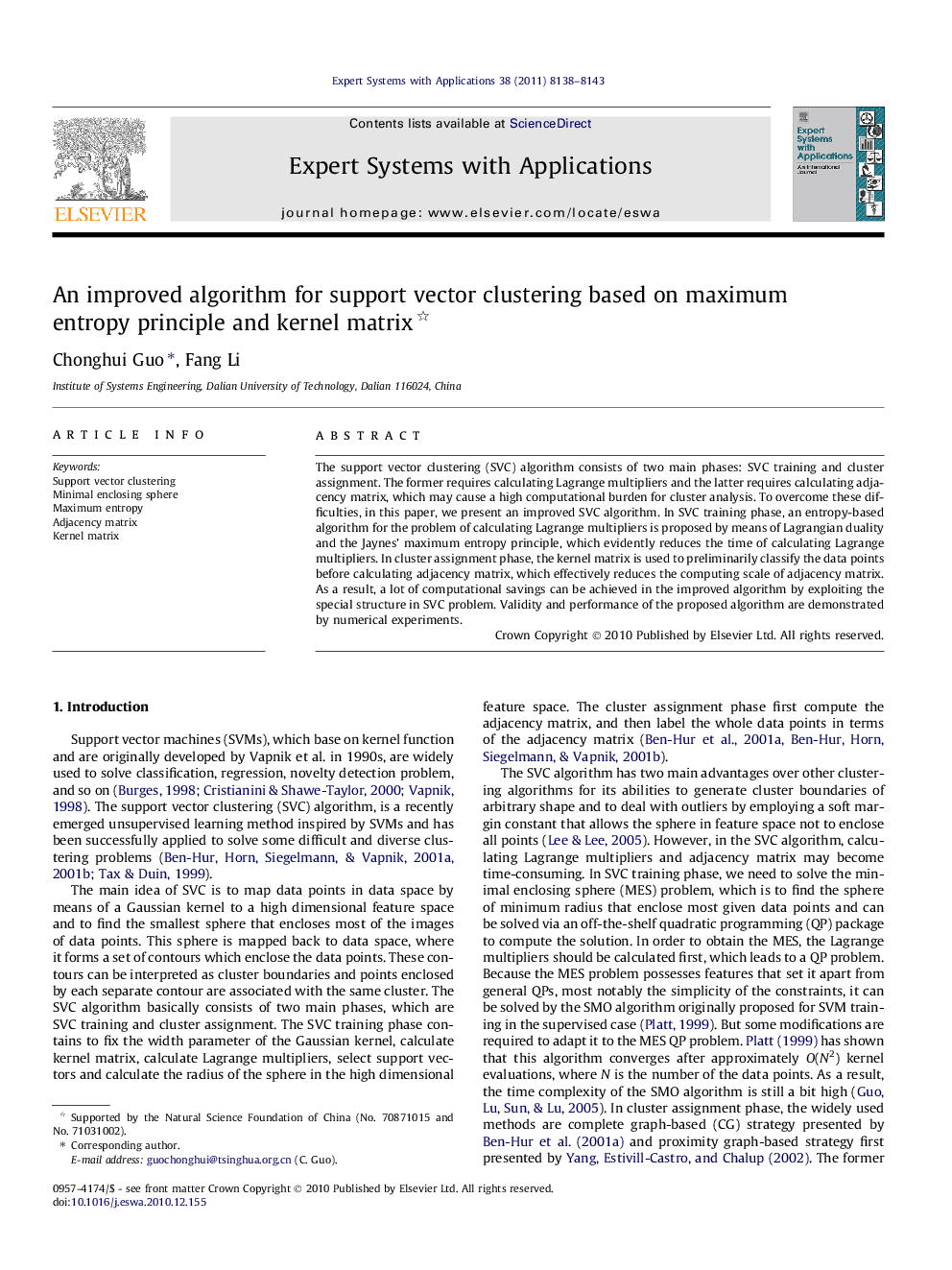
The support vector clustering (SVC) algorithm consists of two main phases: SVC training and cluster assignment. The former requires calculating Lagrange multipliers and the latter requires calculating adjacency matrix, which may cause a high computational burden for cluster analysis. To overcome these difficulties, in this paper, we present an improved SVC algorithm. In SVC training phase, an entropy-based algorithm for the problem of calculating Lagrange multipliers is proposed by means of Lagrangian duality and the Jaynes’ maximum entropy principle, which evidently reduces the time of calculating Lagrange multipliers. In cluster assignment phase, the kernel matrix is used to preliminarily classify the data points before calculating adjacency matrix, which effectively reduces the computing scale of adjacency matrix. As a result, a lot of computational savings can be achieved in the improved algorithm by exploiting the special structure in SVC problem. Validity and performance of the proposed algorithm are demonstrated by numerical experiments.
Research highlights
► We present an improved SVC algorithm support vector clustering based on maximum entropy principle and kernel matrix.
► In SVC training phase, an entropy-based algorithm for the problem of calculating Lagrange multipliers is proposed.
► In cluster assignment phase, the kernel matrix is used to preliminarily classify the data points before calculating adjacency matrix.
► A lot of computational savings can be achieved in the improved algorithm by exploiting the special structure in SVC problem.
Journal: Expert Systems with Applications - Volume 38, Issue 7, July 2011, Pages 8138–8143