کد مقاله | کد نشریه | سال انتشار | مقاله انگلیسی | نسخه تمام متن |
---|---|---|---|---|
385652 | 660869 | 2011 | 8 صفحه PDF | دانلود رایگان |
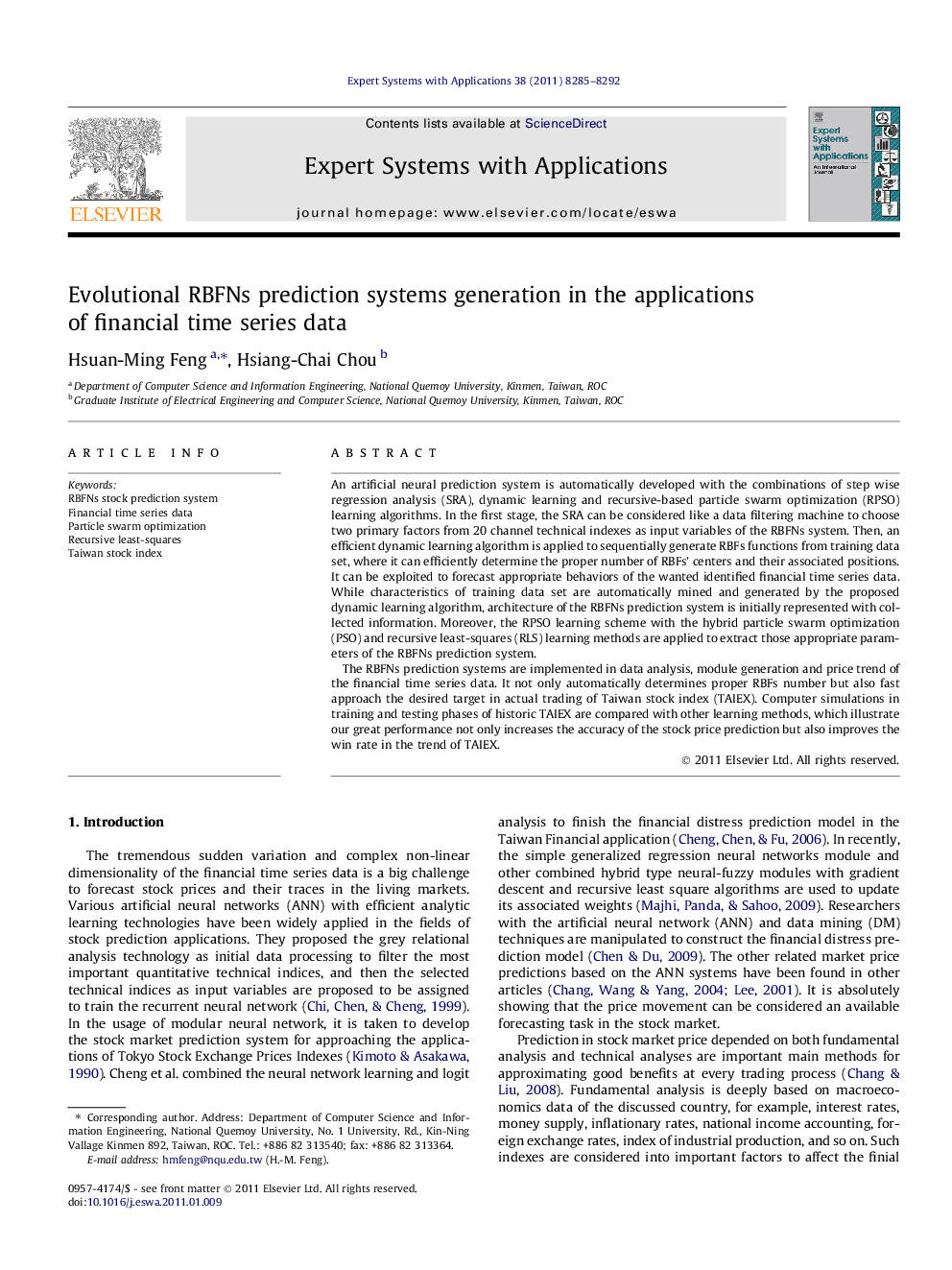
An artificial neural prediction system is automatically developed with the combinations of step wise regression analysis (SRA), dynamic learning and recursive-based particle swarm optimization (RPSO) learning algorithms. In the first stage, the SRA can be considered like a data filtering machine to choose two primary factors from 20 channel technical indexes as input variables of the RBFNs system. Then, an efficient dynamic learning algorithm is applied to sequentially generate RBFs functions from training data set, where it can efficiently determine the proper number of RBFs’ centers and their associated positions. It can be exploited to forecast appropriate behaviors of the wanted identified financial time series data. While characteristics of training data set are automatically mined and generated by the proposed dynamic learning algorithm, architecture of the RBFNs prediction system is initially represented with collected information. Moreover, the RPSO learning scheme with the hybrid particle swarm optimization (PSO) and recursive least-squares (RLS) learning methods are applied to extract those appropriate parameters of the RBFNs prediction system.The RBFNs prediction systems are implemented in data analysis, module generation and price trend of the financial time series data. It not only automatically determines proper RBFs number but also fast approach the desired target in actual trading of Taiwan stock index (TAIEX). Computer simulations in training and testing phases of historic TAIEX are compared with other learning methods, which illustrate our great performance not only increases the accuracy of the stock price prediction but also improves the win rate in the trend of TAIEX.
Research highlights
► Taiwan stock index prediction models are represented by the RBFNs.
► Primary inputs selection of RBFNs is to reduce the complicated stock dataset.
► Dynamic learning algorithms automatically develop the initial RBFNs system.
► Recursive-based particle swarm optimization can efficiently achieve the stock prices.
► Approaching both 0.94% error and 91% win rate in various stock index periods.
Journal: Expert Systems with Applications - Volume 38, Issue 7, July 2011, Pages 8285–8292