کد مقاله | کد نشریه | سال انتشار | مقاله انگلیسی | نسخه تمام متن |
---|---|---|---|---|
388541 | 660926 | 2011 | 10 صفحه PDF | دانلود رایگان |
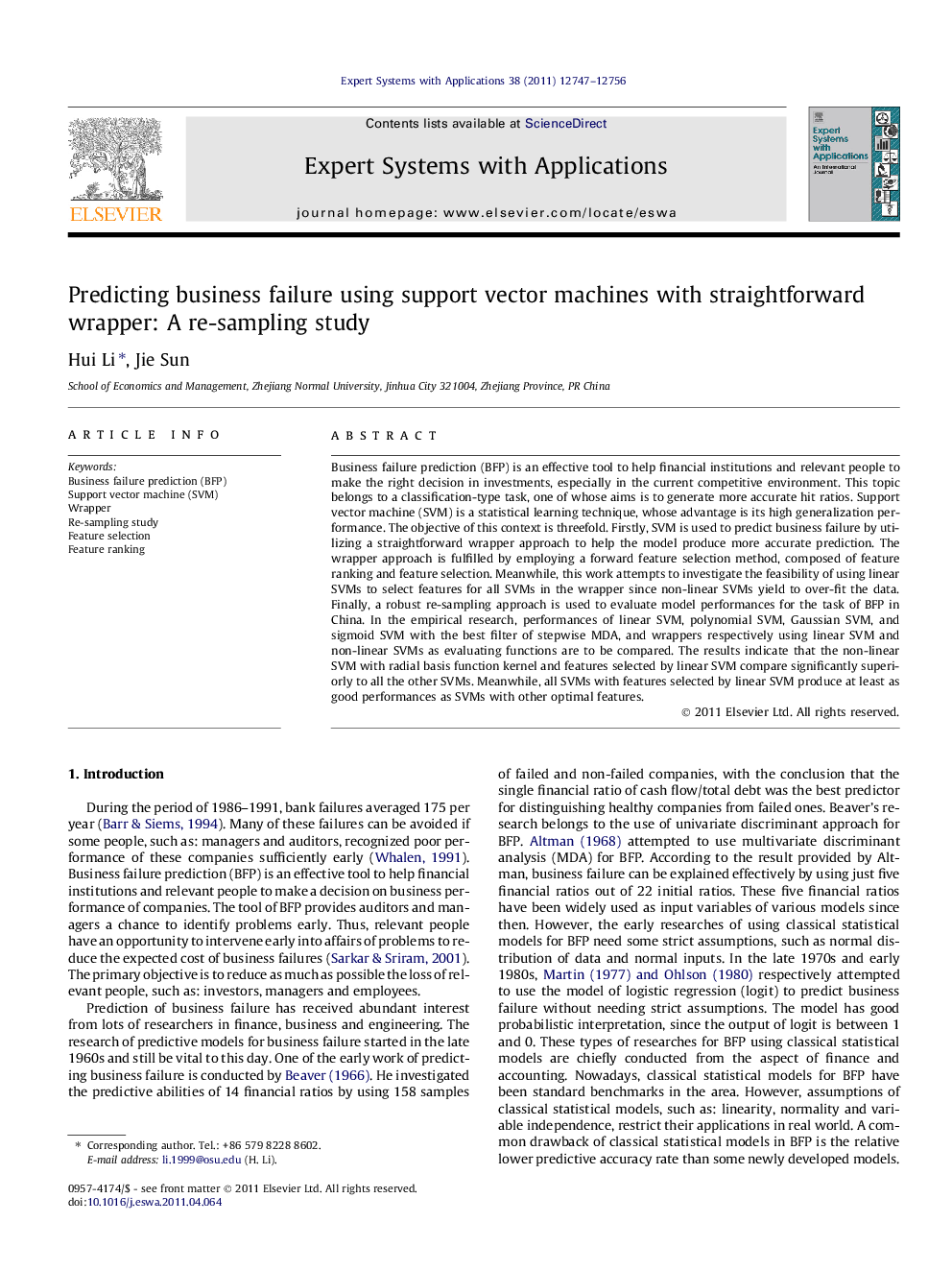
Business failure prediction (BFP) is an effective tool to help financial institutions and relevant people to make the right decision in investments, especially in the current competitive environment. This topic belongs to a classification-type task, one of whose aims is to generate more accurate hit ratios. Support vector machine (SVM) is a statistical learning technique, whose advantage is its high generalization performance. The objective of this context is threefold. Firstly, SVM is used to predict business failure by utilizing a straightforward wrapper approach to help the model produce more accurate prediction. The wrapper approach is fulfilled by employing a forward feature selection method, composed of feature ranking and feature selection. Meanwhile, this work attempts to investigate the feasibility of using linear SVMs to select features for all SVMs in the wrapper since non-linear SVMs yield to over-fit the data. Finally, a robust re-sampling approach is used to evaluate model performances for the task of BFP in China. In the empirical research, performances of linear SVM, polynomial SVM, Gaussian SVM, and sigmoid SVM with the best filter of stepwise MDA, and wrappers respectively using linear SVM and non-linear SVMs as evaluating functions are to be compared. The results indicate that the non-linear SVM with radial basis function kernel and features selected by linear SVM compare significantly superiorly to all the other SVMs. Meanwhile, all SVMs with features selected by linear SVM produce at least as good performances as SVMs with other optimal features.
► A straightforward wrapper approach was used to help SVM model produce more accurate prediction of business failure.
► Linear SVM was used to select features for all SVMs in the wrapper in order to avoid over-fitting in feature selection.
► The non-linear SVM with RBF kernel and features selected by linear SVM produced significantly superior performance.
► All SVMs with features selected by linear SVM produced at least as good performances as SVMs with other optimal features.
Journal: Expert Systems with Applications - Volume 38, Issue 10, 15 September 2011, Pages 12747–12756