کد مقاله | کد نشریه | سال انتشار | مقاله انگلیسی | نسخه تمام متن |
---|---|---|---|---|
403695 | 677312 | 2013 | 8 صفحه PDF | دانلود رایگان |
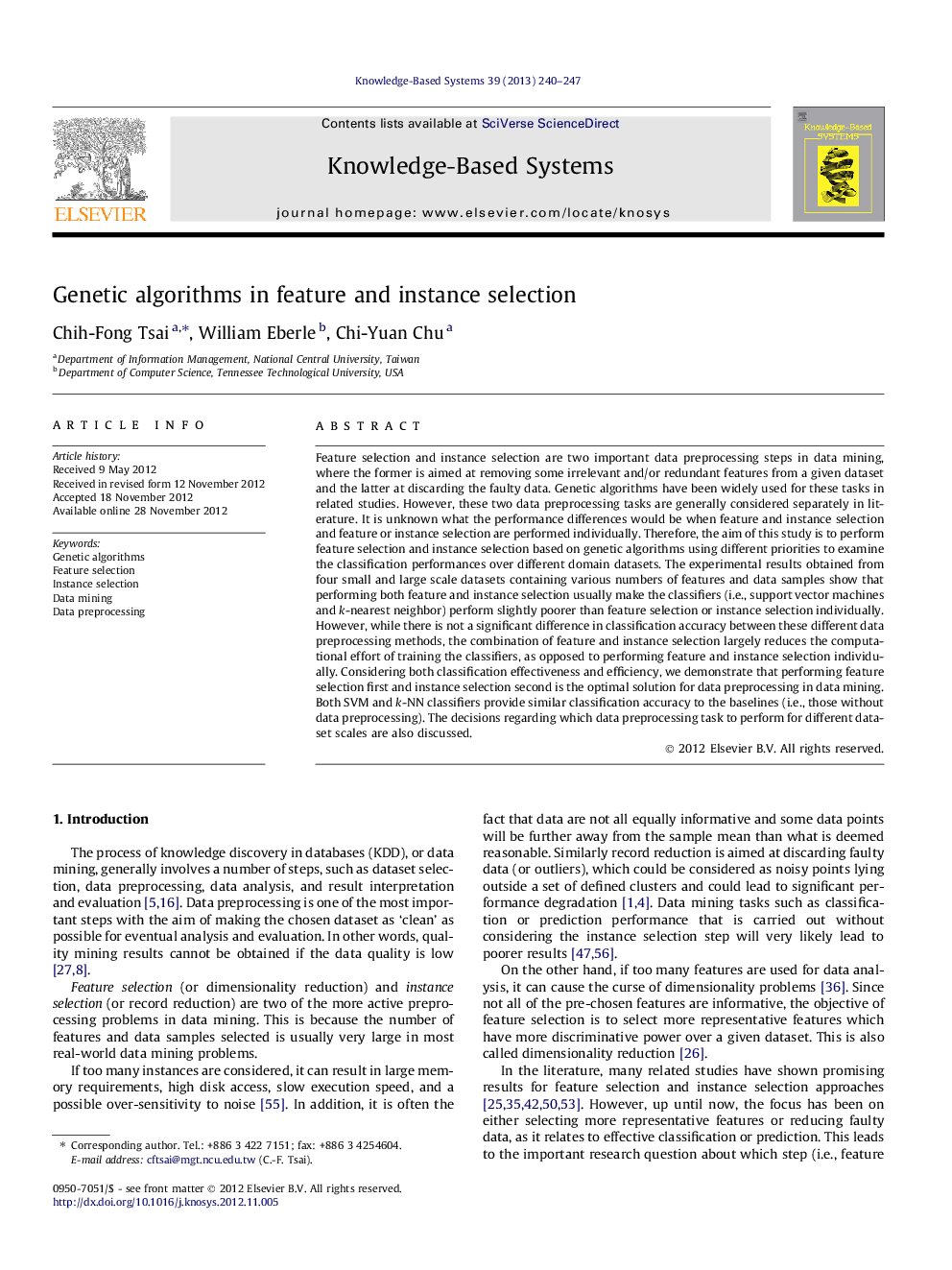
Feature selection and instance selection are two important data preprocessing steps in data mining, where the former is aimed at removing some irrelevant and/or redundant features from a given dataset and the latter at discarding the faulty data. Genetic algorithms have been widely used for these tasks in related studies. However, these two data preprocessing tasks are generally considered separately in literature. It is unknown what the performance differences would be when feature and instance selection and feature or instance selection are performed individually. Therefore, the aim of this study is to perform feature selection and instance selection based on genetic algorithms using different priorities to examine the classification performances over different domain datasets. The experimental results obtained from four small and large scale datasets containing various numbers of features and data samples show that performing both feature and instance selection usually make the classifiers (i.e., support vector machines and k-nearest neighbor) perform slightly poorer than feature selection or instance selection individually. However, while there is not a significant difference in classification accuracy between these different data preprocessing methods, the combination of feature and instance selection largely reduces the computational effort of training the classifiers, as opposed to performing feature and instance selection individually. Considering both classification effectiveness and efficiency, we demonstrate that performing feature selection first and instance selection second is the optimal solution for data preprocessing in data mining. Both SVM and k-NN classifiers provide similar classification accuracy to the baselines (i.e., those without data preprocessing). The decisions regarding which data preprocessing task to perform for different dataset scales are also discussed.
Journal: Knowledge-Based Systems - Volume 39, February 2013, Pages 240–247