کد مقاله | کد نشریه | سال انتشار | مقاله انگلیسی | نسخه تمام متن |
---|---|---|---|---|
404376 | 677417 | 2011 | 19 صفحه PDF | دانلود رایگان |
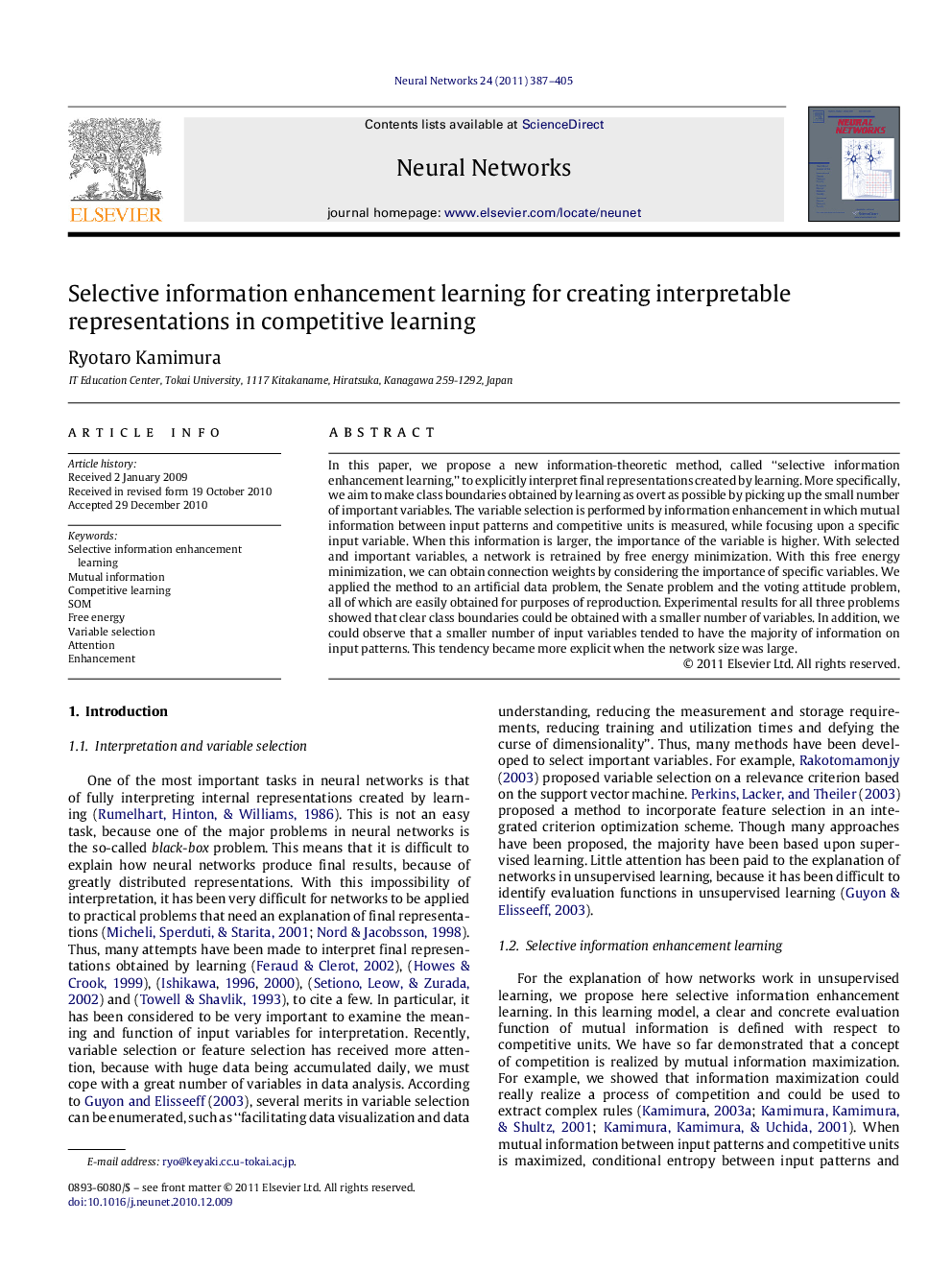
In this paper, we propose a new information-theoretic method, called “selective information enhancement learning,” to explicitly interpret final representations created by learning. More specifically, we aim to make class boundaries obtained by learning as overt as possible by picking up the small number of important variables. The variable selection is performed by information enhancement in which mutual information between input patterns and competitive units is measured, while focusing upon a specific input variable. When this information is larger, the importance of the variable is higher. With selected and important variables, a network is retrained by free energy minimization. With this free energy minimization, we can obtain connection weights by considering the importance of specific variables. We applied the method to an artificial data problem, the Senate problem and the voting attitude problem, all of which are easily obtained for purposes of reproduction. Experimental results for all three problems showed that clear class boundaries could be obtained with a smaller number of variables. In addition, we could observe that a smaller number of input variables tended to have the majority of information on input patterns. This tendency became more explicit when the network size was large.
Journal: Neural Networks - Volume 24, Issue 4, May 2011, Pages 387–405