کد مقاله | کد نشریه | سال انتشار | مقاله انگلیسی | نسخه تمام متن |
---|---|---|---|---|
404477 | 677427 | 2010 | 12 صفحه PDF | دانلود رایگان |
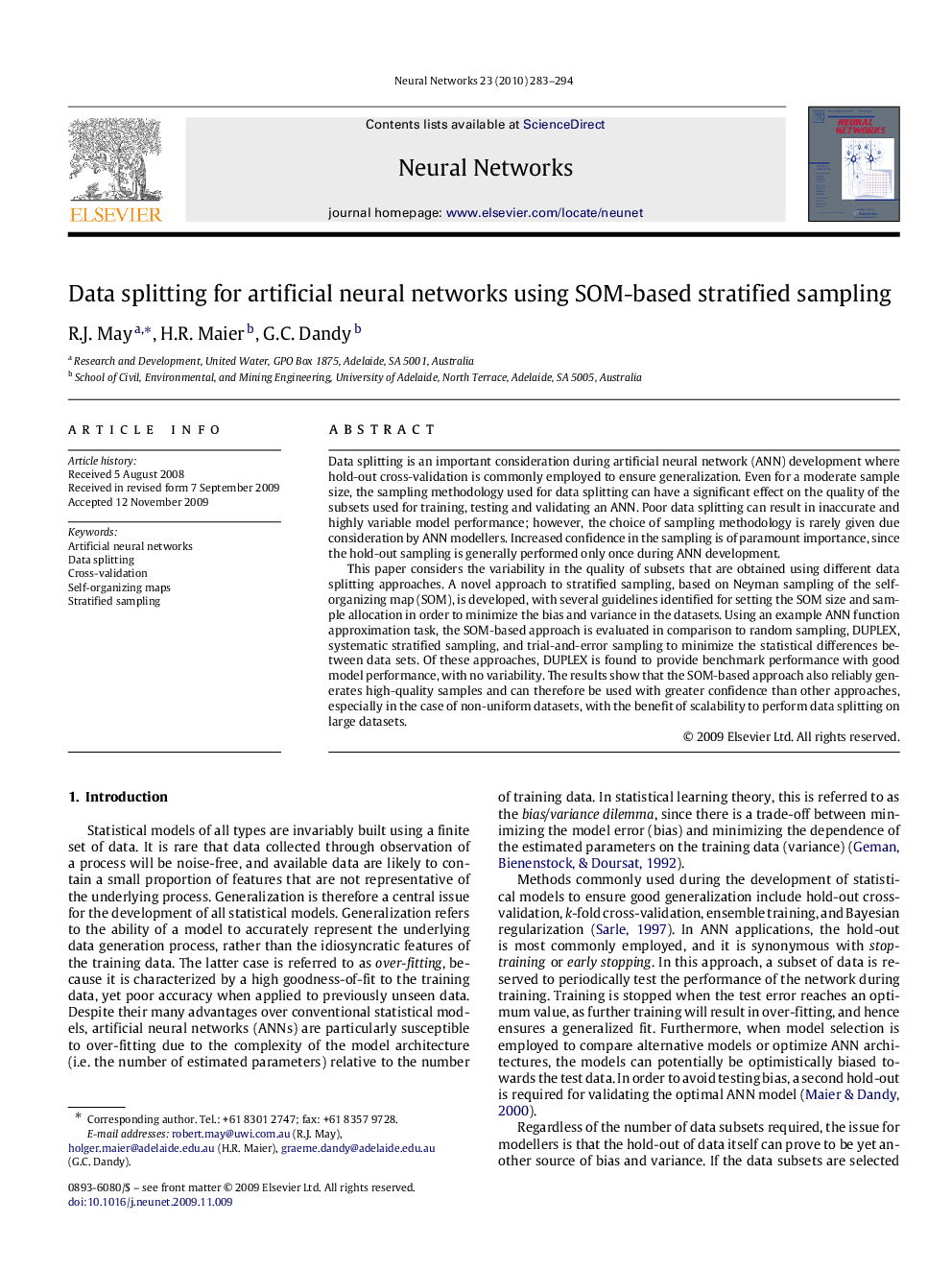
Data splitting is an important consideration during artificial neural network (ANN) development where hold-out cross-validation is commonly employed to ensure generalization. Even for a moderate sample size, the sampling methodology used for data splitting can have a significant effect on the quality of the subsets used for training, testing and validating an ANN. Poor data splitting can result in inaccurate and highly variable model performance; however, the choice of sampling methodology is rarely given due consideration by ANN modellers. Increased confidence in the sampling is of paramount importance, since the hold-out sampling is generally performed only once during ANN development.This paper considers the variability in the quality of subsets that are obtained using different data splitting approaches. A novel approach to stratified sampling, based on Neyman sampling of the self-organizing map (SOM), is developed, with several guidelines identified for setting the SOM size and sample allocation in order to minimize the bias and variance in the datasets. Using an example ANN function approximation task, the SOM-based approach is evaluated in comparison to random sampling, DUPLEX, systematic stratified sampling, and trial-and-error sampling to minimize the statistical differences between data sets. Of these approaches, DUPLEX is found to provide benchmark performance with good model performance, with no variability. The results show that the SOM-based approach also reliably generates high-quality samples and can therefore be used with greater confidence than other approaches, especially in the case of non-uniform datasets, with the benefit of scalability to perform data splitting on large datasets.
Journal: Neural Networks - Volume 23, Issue 2, March 2010, Pages 283–294