کد مقاله | کد نشریه | سال انتشار | مقاله انگلیسی | نسخه تمام متن |
---|---|---|---|---|
405286 | 677519 | 2011 | 9 صفحه PDF | دانلود رایگان |
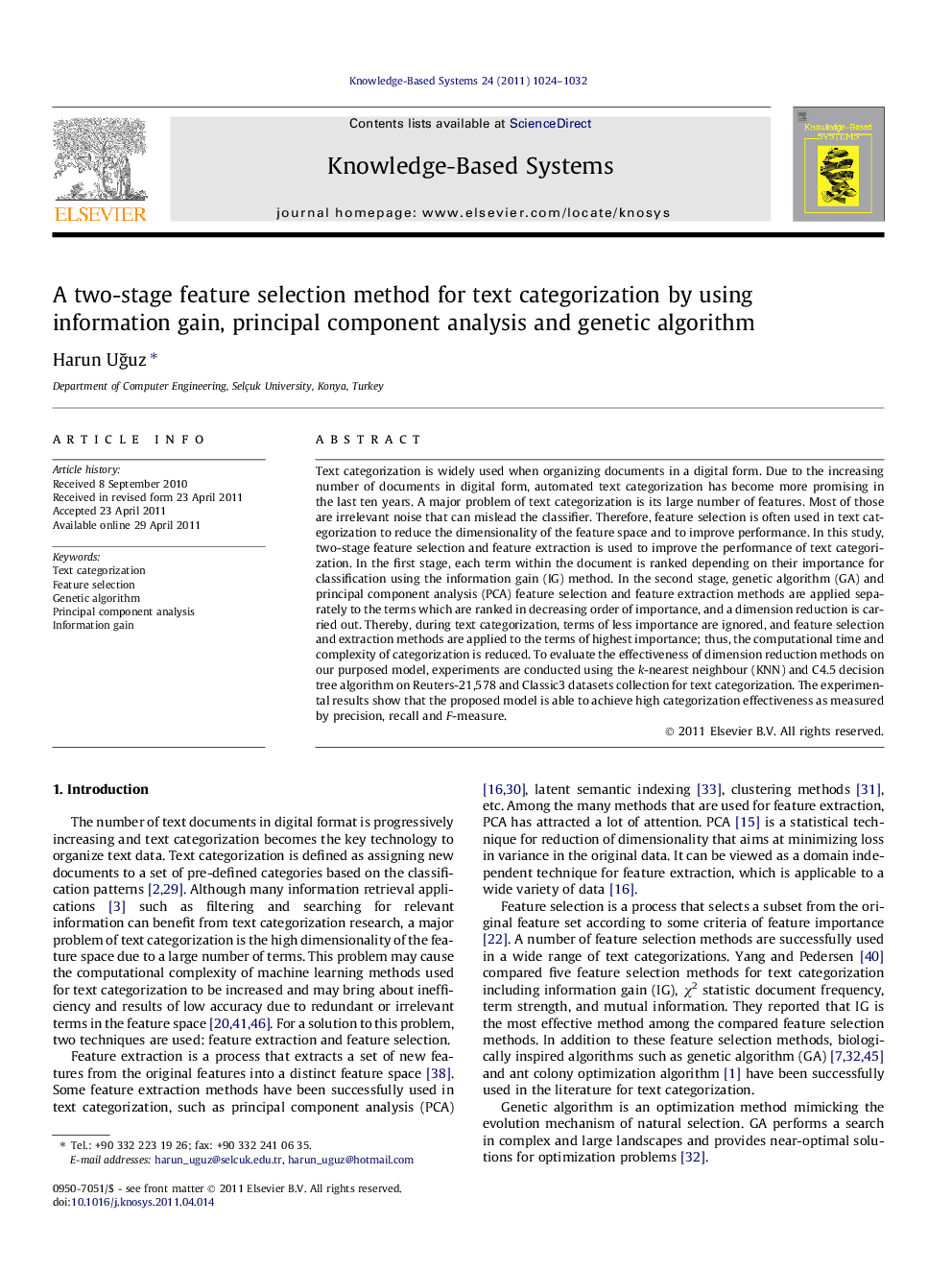
Text categorization is widely used when organizing documents in a digital form. Due to the increasing number of documents in digital form, automated text categorization has become more promising in the last ten years. A major problem of text categorization is its large number of features. Most of those are irrelevant noise that can mislead the classifier. Therefore, feature selection is often used in text categorization to reduce the dimensionality of the feature space and to improve performance. In this study, two-stage feature selection and feature extraction is used to improve the performance of text categorization. In the first stage, each term within the document is ranked depending on their importance for classification using the information gain (IG) method. In the second stage, genetic algorithm (GA) and principal component analysis (PCA) feature selection and feature extraction methods are applied separately to the terms which are ranked in decreasing order of importance, and a dimension reduction is carried out. Thereby, during text categorization, terms of less importance are ignored, and feature selection and extraction methods are applied to the terms of highest importance; thus, the computational time and complexity of categorization is reduced. To evaluate the effectiveness of dimension reduction methods on our purposed model, experiments are conducted using the k-nearest neighbour (KNN) and C4.5 decision tree algorithm on Reuters-21,578 and Classic3 datasets collection for text categorization. The experimental results show that the proposed model is able to achieve high categorization effectiveness as measured by precision, recall and F-measure.
Journal: Knowledge-Based Systems - Volume 24, Issue 7, October 2011, Pages 1024–1032