کد مقاله | کد نشریه | سال انتشار | مقاله انگلیسی | نسخه تمام متن |
---|---|---|---|---|
406120 | 678064 | 2016 | 8 صفحه PDF | دانلود رایگان |
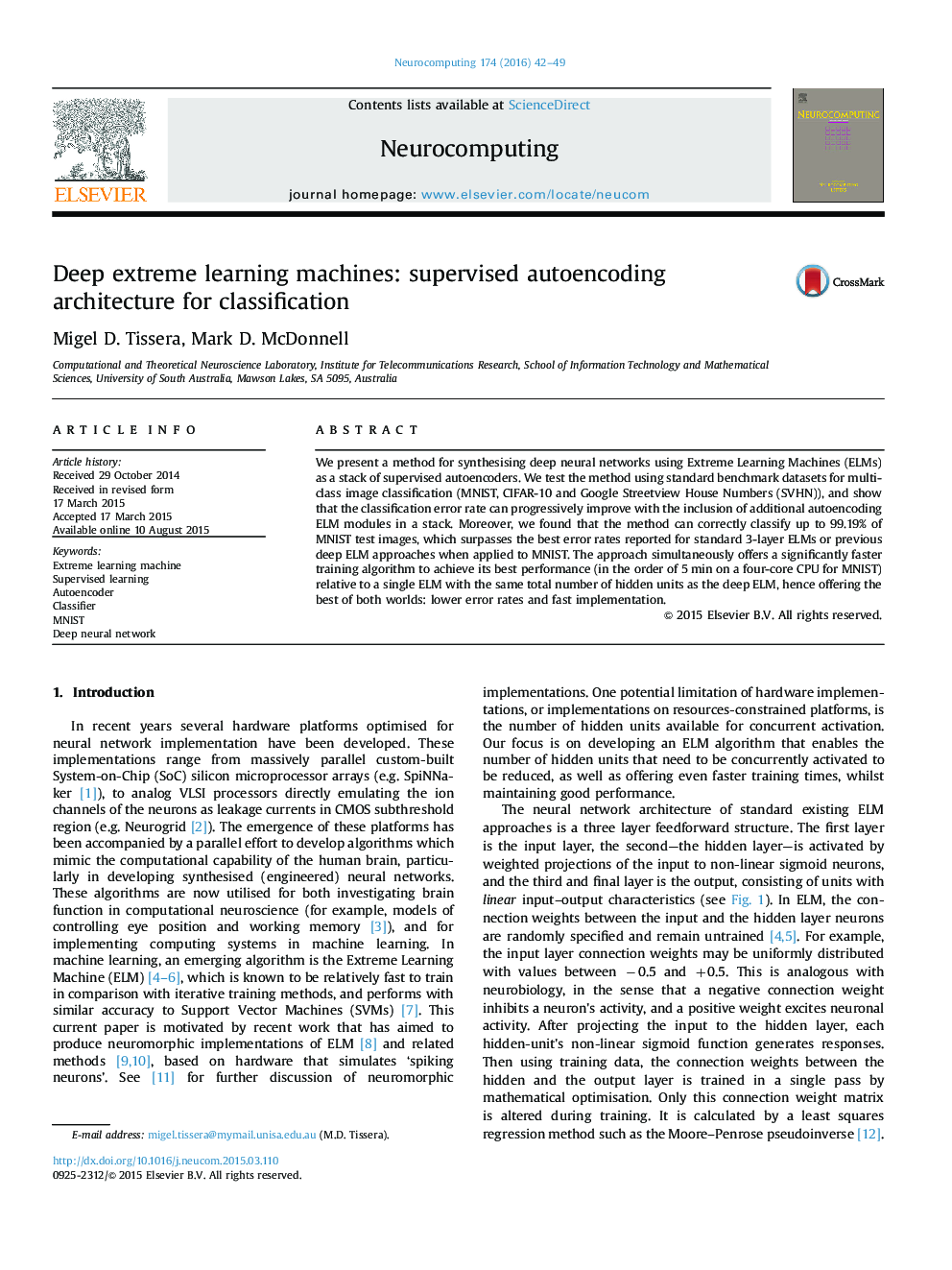
We present a method for synthesising deep neural networks using Extreme Learning Machines (ELMs) as a stack of supervised autoencoders. We test the method using standard benchmark datasets for multi-class image classification (MNIST, CIFAR-10 and Google Streetview House Numbers (SVHN)), and show that the classification error rate can progressively improve with the inclusion of additional autoencoding ELM modules in a stack. Moreover, we found that the method can correctly classify up to 99.19% of MNIST test images, which surpasses the best error rates reported for standard 3-layer ELMs or previous deep ELM approaches when applied to MNIST. The approach simultaneously offers a significantly faster training algorithm to achieve its best performance (in the order of 5 min on a four-core CPU for MNIST) relative to a single ELM with the same total number of hidden units as the deep ELM, hence offering the best of both worlds: lower error rates and fast implementation.
Journal: Neurocomputing - Volume 174, Part A, 22 January 2016, Pages 42–49