کد مقاله | کد نشریه | سال انتشار | مقاله انگلیسی | نسخه تمام متن |
---|---|---|---|---|
406777 | 678111 | 2014 | 21 صفحه PDF | دانلود رایگان |
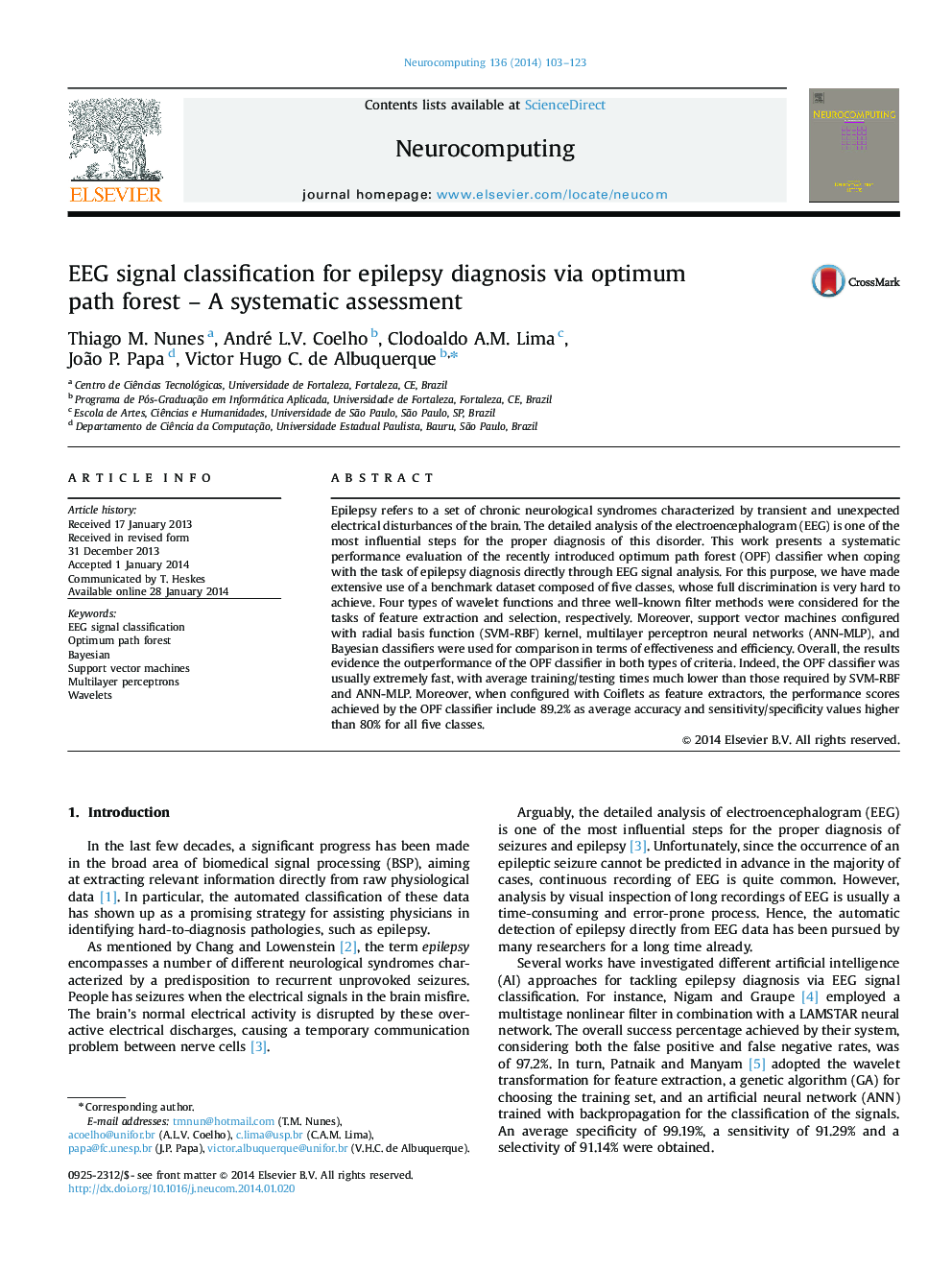
• Thorough assessment of the OPF classifier when coping with the task of epilepsy diagnosis through EEG signal classification.
• Analysis of the impact of the data preprocessing methods as well as the distance measure used.
• Comparison of the OPF classifier with SVM-RBF, ANN-MLP, and Bayesian classifiers.
• The OPF classifier has outperformed the other models in terms of efficiency and effectiveness criteria.
Epilepsy refers to a set of chronic neurological syndromes characterized by transient and unexpected electrical disturbances of the brain. The detailed analysis of the electroencephalogram (EEG) is one of the most influential steps for the proper diagnosis of this disorder. This work presents a systematic performance evaluation of the recently introduced optimum path forest (OPF) classifier when coping with the task of epilepsy diagnosis directly through EEG signal analysis. For this purpose, we have made extensive use of a benchmark dataset composed of five classes, whose full discrimination is very hard to achieve. Four types of wavelet functions and three well-known filter methods were considered for the tasks of feature extraction and selection, respectively. Moreover, support vector machines configured with radial basis function (SVM-RBF) kernel, multilayer perceptron neural networks (ANN-MLP), and Bayesian classifiers were used for comparison in terms of effectiveness and efficiency. Overall, the results evidence the outperformance of the OPF classifier in both types of criteria. Indeed, the OPF classifier was usually extremely fast, with average training/testing times much lower than those required by SVM-RBF and ANN-MLP. Moreover, when configured with Coiflets as feature extractors, the performance scores achieved by the OPF classifier include 89.2% as average accuracy and sensitivity/specificity values higher than 80% for all five classes.
Journal: Neurocomputing - Volume 136, 20 July 2014, Pages 103–123