کد مقاله | کد نشریه | سال انتشار | مقاله انگلیسی | نسخه تمام متن |
---|---|---|---|---|
407596 | 678158 | 2013 | 12 صفحه PDF | دانلود رایگان |
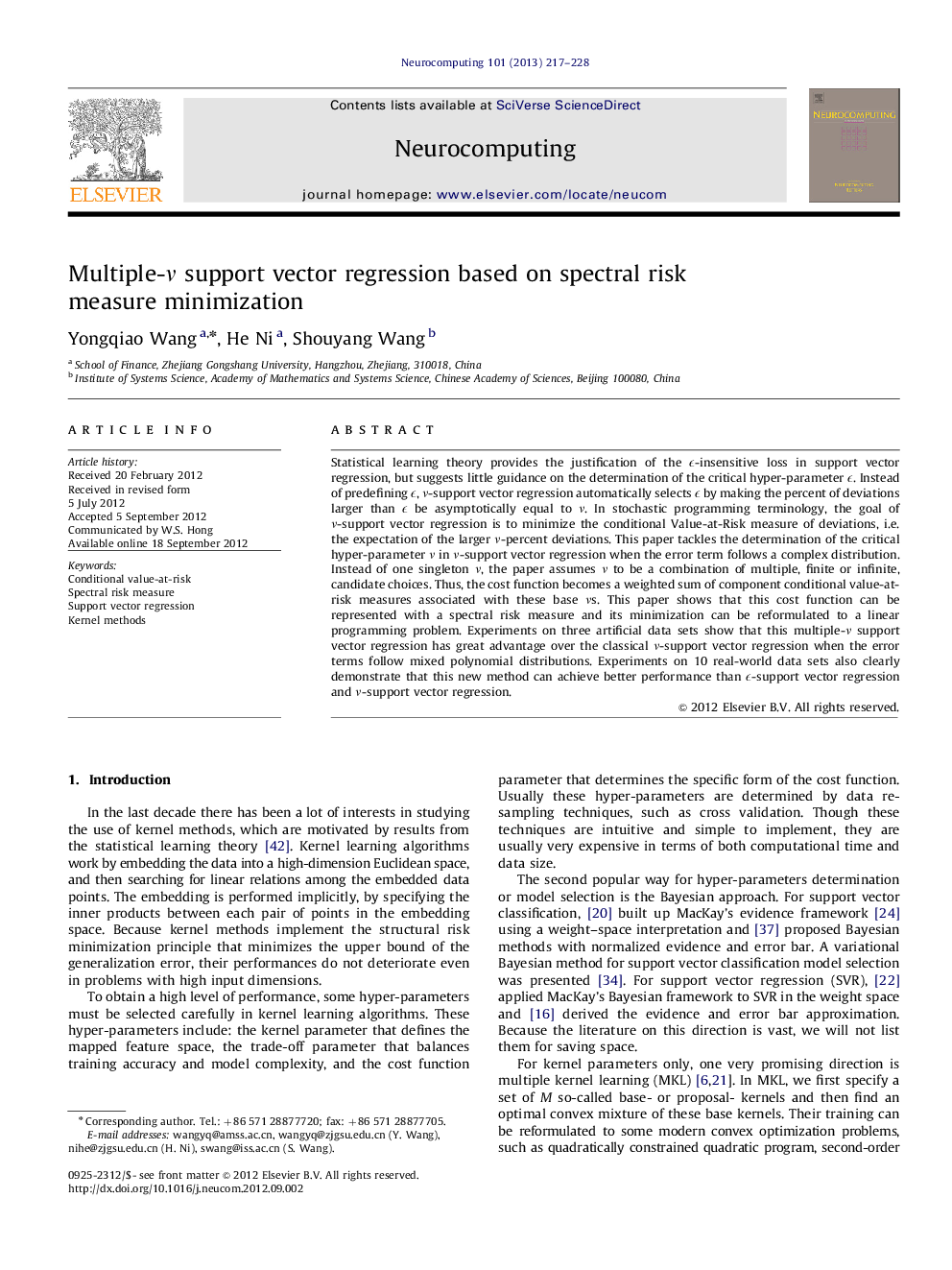
Statistical learning theory provides the justification of the ϵ-insensitiveϵ-insensitive loss in support vector regression, but suggests little guidance on the determination of the critical hyper-parameter ϵϵ. Instead of predefining ϵϵ, ν-supportν-support vector regression automatically selects ϵϵ by making the percent of deviations larger than ϵϵ be asymptotically equal to νν. In stochastic programming terminology, the goal of ν-supportν-support vector regression is to minimize the conditional Value-at-Risk measure of deviations, i.e. the expectation of the larger ν-percentν-percent deviations. This paper tackles the determination of the critical hyper-parameter νν in ν-supportν-support vector regression when the error term follows a complex distribution. Instead of one singleton νν, the paper assumes νν to be a combination of multiple, finite or infinite, candidate choices. Thus, the cost function becomes a weighted sum of component conditional value-at-risk measures associated with these base νsνs. This paper shows that this cost function can be represented with a spectral risk measure and its minimization can be reformulated to a linear programming problem. Experiments on three artificial data sets show that this multiple-νν support vector regression has great advantage over the classical ν-supportν-support vector regression when the error terms follow mixed polynomial distributions. Experiments on 10 real-world data sets also clearly demonstrate that this new method can achieve better performance than ϵ-supportϵ-support vector regression and ν-supportν-support vector regression.
Journal: Neurocomputing - Volume 101, 4 February 2013, Pages 217–228