کد مقاله | کد نشریه | سال انتشار | مقاله انگلیسی | نسخه تمام متن |
---|---|---|---|---|
407626 | 678159 | 2012 | 12 صفحه PDF | دانلود رایگان |
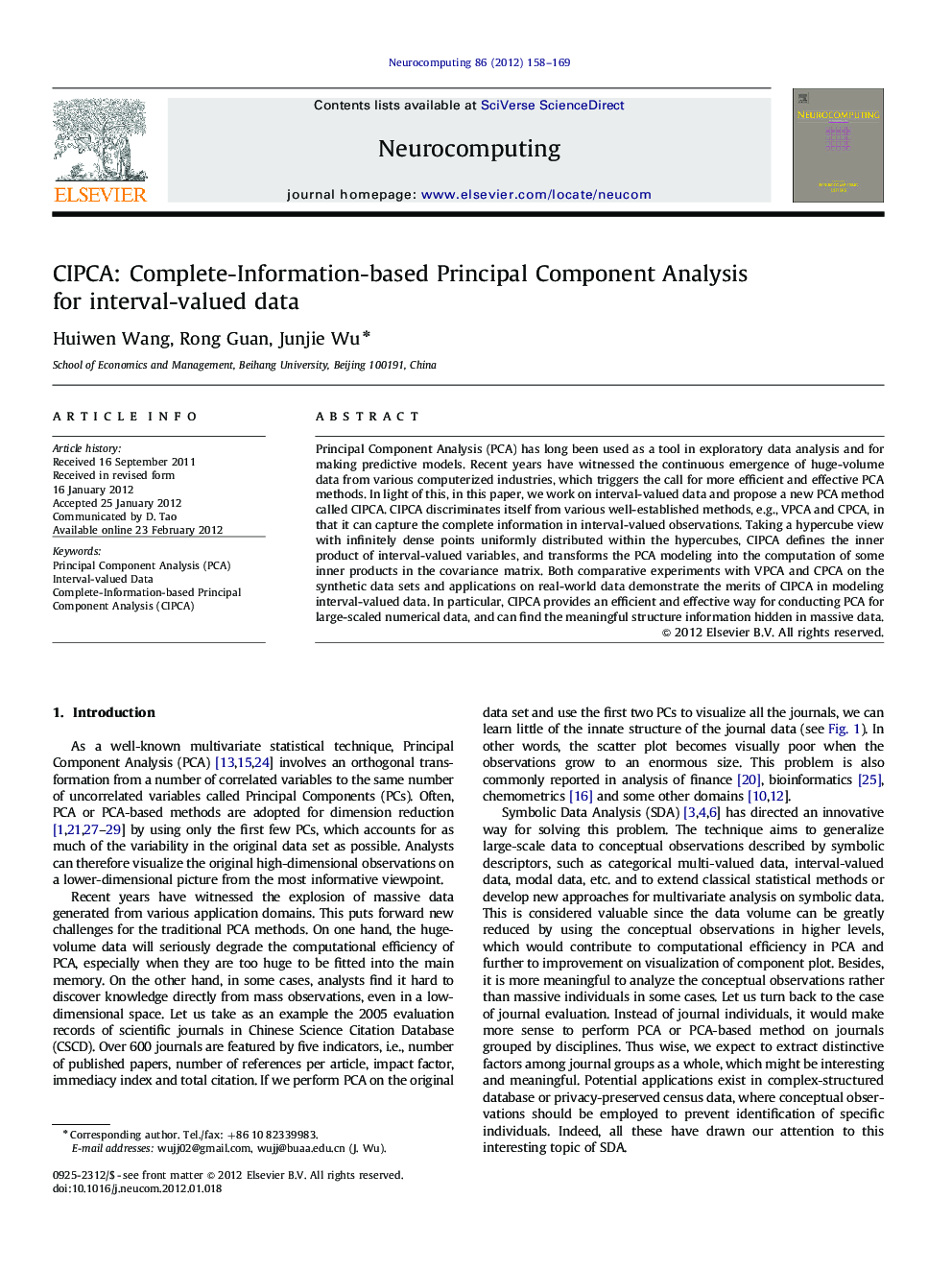
Principal Component Analysis (PCA) has long been used as a tool in exploratory data analysis and for making predictive models. Recent years have witnessed the continuous emergence of huge-volume data from various computerized industries, which triggers the call for more efficient and effective PCA methods. In light of this, in this paper, we work on interval-valued data and propose a new PCA method called CIPCA. CIPCA discriminates itself from various well-established methods, e.g., VPCA and CPCA, in that it can capture the complete information in interval-valued observations. Taking a hypercube view with infinitely dense points uniformly distributed within the hypercubes, CIPCA defines the inner product of interval-valued variables, and transforms the PCA modeling into the computation of some inner products in the covariance matrix. Both comparative experiments with VPCA and CPCA on the synthetic data sets and applications on real-world data demonstrate the merits of CIPCA in modeling interval-valued data. In particular, CIPCA provides an efficient and effective way for conducting PCA for large-scaled numerical data, and can find the meaningful structure information hidden in massive data.
Journal: Neurocomputing - Volume 86, 1 June 2012, Pages 158–169